Researchers Use Natural-Language Processing (NLP) Algorithms to Predict SARS-CoV-2 Virus Mutations
By LabMedica International staff writers Posted on 18 Jan 2021 |
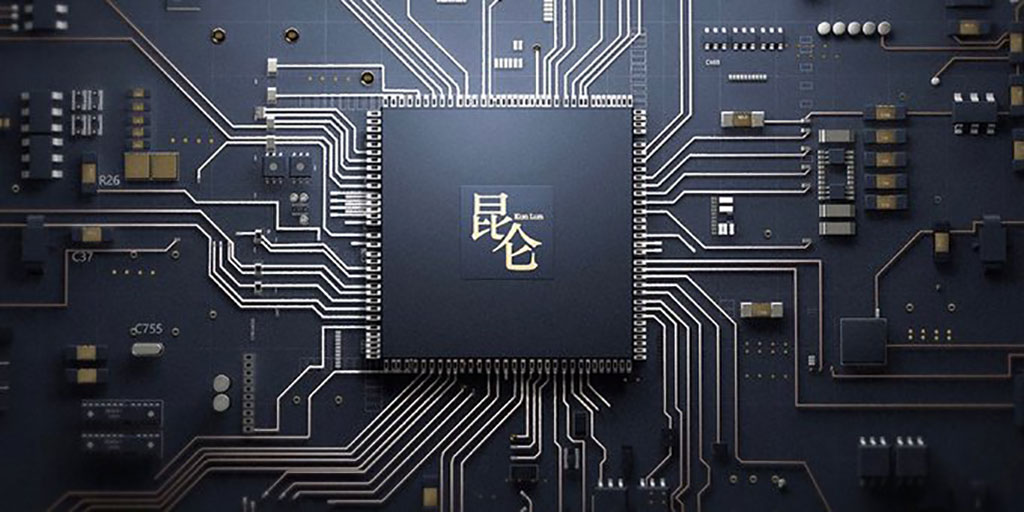
Image: Researchers Use NLP Algorithms to Predict SARS-CoV-2 Virus Mutations (Photo courtesy of Baidu)
Natural-language processing (NLP) algorithms are now able to generate protein sequences and predict virus mutations, including key changes that help the SARS-CoV-2 virus evade the immune system.
The key insight making this possible is that many properties of biological systems can be interpreted in terms of words and sentences. In the last few years, a handful of researchers have shown that protein sequences and genetic codes can be modeled using NLP techniques. Now, computational biologists at the Massachusetts Institute of Technology (MIT; Cambridge, MA, USA) have pulled several of these strands together and use NLP to predict mutations that allow viruses to avoid being detected by antibodies in the human immune system, a process known as viral immune escape. The basic idea is that the interpretation of a virus by an immune system is analogous to the interpretation of a sentence by a human.
The team uses two different linguistic concepts: grammar and semantics (or meaning). The genetic or evolutionary fitness of a virus - characteristics such as how good it is at infecting a host - can be interpreted in terms of grammatical correctness. A successful, infectious virus is grammatically correct; an unsuccessful one is not. Similarly, mutations of a virus can be interpreted in terms of semantics. Mutations that make a virus appear different to things in its environment - such as changes in its surface proteins that make it invisible to certain antibodies - have altered its meaning. Viruses with different mutations can have different meanings, and a virus with a different meaning may need different antibodies to read it.
To model these properties, the researchers used an LSTM, a type of neural network that predates the transformer-based ones used by large language models like GPT-3. These older networks can be trained on far less data than transformers and still perform well for many applications. Instead of millions of sentences, they trained the NLP model on thousands of genetic sequences taken from three different viruses: 45,000 unique sequences for a strain of influenza, 60,000 for a strain of HIV, and between 3,000 and 4,000 for a strain of the SARS-CoV-2 virus.
NLP models work by encoding words in a mathematical space in such a way that words with similar meanings are closer together than words with different meanings. This is known as an embedding. For viruses, the embedding of the genetic sequences grouped viruses according to how similar their mutations were. The overall aim of the approach is to identify mutations that might let a virus escape an immune system without making it less infectious - that is, mutations that change a virus’s meaning without making it grammatically incorrect.
To test their approach, the team used a common metric for assessing predictions made by machine-learning models that scores accuracy on a scale between 0.5 (no better than chance) and 1 (perfect). In this case, they took the top mutations identified by the tool and, using real viruses in a lab, checked how many of them were actual escape mutations. Their results ranged from 0.69 for HIV to 0.85 for one coronavirus strain. This is better than results from other state-of-the-art models, according to the researchers.
The team has been running models on new variants of the coronavirus, including the so-called UK mutation, the mink mutation from Denmark, and variants taken from South Africa, Singapore and Malaysia. Using NLP accelerates a slow process. Previously, the genome of the virus taken from a COVID-19 patient in hospital could be sequenced and its mutations re-created and studied in a lab. However, that can take weeks, whereas the NLP model predicts potential mutations straight away, which focuses the lab work and speeds it up.
“We’re learning the language of evolution,” said Bonnie Berger, a computational biologist at the Massachusetts Institute of Technology. “Biology has its own language.”
Related Links:
Massachusetts Institute of Technology (MIT)
The key insight making this possible is that many properties of biological systems can be interpreted in terms of words and sentences. In the last few years, a handful of researchers have shown that protein sequences and genetic codes can be modeled using NLP techniques. Now, computational biologists at the Massachusetts Institute of Technology (MIT; Cambridge, MA, USA) have pulled several of these strands together and use NLP to predict mutations that allow viruses to avoid being detected by antibodies in the human immune system, a process known as viral immune escape. The basic idea is that the interpretation of a virus by an immune system is analogous to the interpretation of a sentence by a human.
The team uses two different linguistic concepts: grammar and semantics (or meaning). The genetic or evolutionary fitness of a virus - characteristics such as how good it is at infecting a host - can be interpreted in terms of grammatical correctness. A successful, infectious virus is grammatically correct; an unsuccessful one is not. Similarly, mutations of a virus can be interpreted in terms of semantics. Mutations that make a virus appear different to things in its environment - such as changes in its surface proteins that make it invisible to certain antibodies - have altered its meaning. Viruses with different mutations can have different meanings, and a virus with a different meaning may need different antibodies to read it.
To model these properties, the researchers used an LSTM, a type of neural network that predates the transformer-based ones used by large language models like GPT-3. These older networks can be trained on far less data than transformers and still perform well for many applications. Instead of millions of sentences, they trained the NLP model on thousands of genetic sequences taken from three different viruses: 45,000 unique sequences for a strain of influenza, 60,000 for a strain of HIV, and between 3,000 and 4,000 for a strain of the SARS-CoV-2 virus.
NLP models work by encoding words in a mathematical space in such a way that words with similar meanings are closer together than words with different meanings. This is known as an embedding. For viruses, the embedding of the genetic sequences grouped viruses according to how similar their mutations were. The overall aim of the approach is to identify mutations that might let a virus escape an immune system without making it less infectious - that is, mutations that change a virus’s meaning without making it grammatically incorrect.
To test their approach, the team used a common metric for assessing predictions made by machine-learning models that scores accuracy on a scale between 0.5 (no better than chance) and 1 (perfect). In this case, they took the top mutations identified by the tool and, using real viruses in a lab, checked how many of them were actual escape mutations. Their results ranged from 0.69 for HIV to 0.85 for one coronavirus strain. This is better than results from other state-of-the-art models, according to the researchers.
The team has been running models on new variants of the coronavirus, including the so-called UK mutation, the mink mutation from Denmark, and variants taken from South Africa, Singapore and Malaysia. Using NLP accelerates a slow process. Previously, the genome of the virus taken from a COVID-19 patient in hospital could be sequenced and its mutations re-created and studied in a lab. However, that can take weeks, whereas the NLP model predicts potential mutations straight away, which focuses the lab work and speeds it up.
“We’re learning the language of evolution,” said Bonnie Berger, a computational biologist at the Massachusetts Institute of Technology. “Biology has its own language.”
Related Links:
Massachusetts Institute of Technology (MIT)
Latest COVID-19 News
- New Immunosensor Paves Way to Rapid POC Testing for COVID-19 and Emerging Infectious Diseases
- Long COVID Etiologies Found in Acute Infection Blood Samples
- Novel Device Detects COVID-19 Antibodies in Five Minutes
- CRISPR-Powered COVID-19 Test Detects SARS-CoV-2 in 30 Minutes Using Gene Scissors
- Gut Microbiome Dysbiosis Linked to COVID-19
- Novel SARS CoV-2 Rapid Antigen Test Validated for Diagnostic Accuracy
- New COVID + Flu + R.S.V. Test to Help Prepare for `Tripledemic`
- AI Takes Guesswork Out Of Lateral Flow Testing
- Fastest Ever SARS-CoV-2 Antigen Test Designed for Non-Invasive COVID-19 Testing in Any Setting
- Rapid Antigen Tests Detect Omicron, Delta SARS-CoV-2 Variants
- Health Care Professionals Showed Increased Interest in POC Technologies During Pandemic, Finds Study
- Set Up Reserve Lab Capacity Now for Faster Response to Next Pandemic, Say Researchers
- Blood Test Performed During Initial Infection Predicts Long COVID Risk
- Low-Cost COVID-19 Testing Platform Combines Sensitivity of PCR and Speed of Antigen Tests
- Finger-Prick Blood Test Identifies Immunity to COVID-19
- Quick Test Kit Determines Immunity Against COVID-19 and Its Variants
Channels
Clinical Chemistry
view channel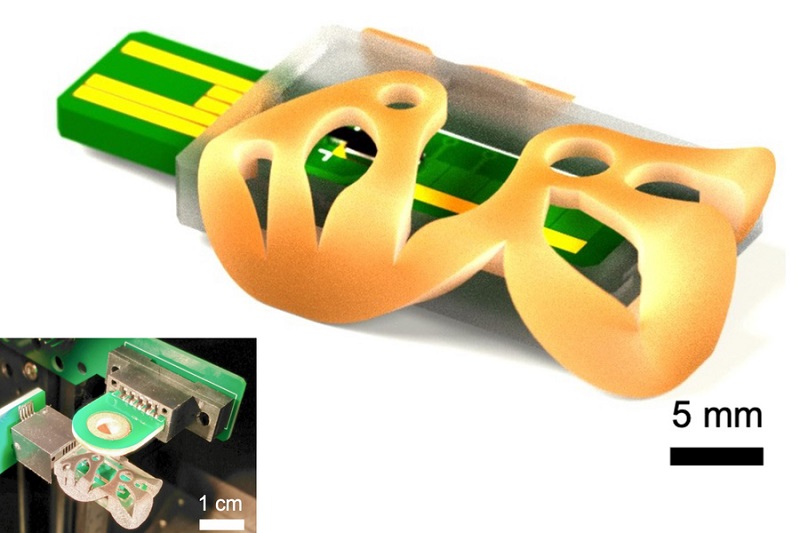
3D Printed Point-Of-Care Mass Spectrometer Outperforms State-Of-The-Art Models
Mass spectrometry is a precise technique for identifying the chemical components of a sample and has significant potential for monitoring chronic illness health states, such as measuring hormone levels... Read more.jpg)
POC Biomedical Test Spins Water Droplet Using Sound Waves for Cancer Detection
Exosomes, tiny cellular bioparticles carrying a specific set of proteins, lipids, and genetic materials, play a crucial role in cell communication and hold promise for non-invasive diagnostics.... Read more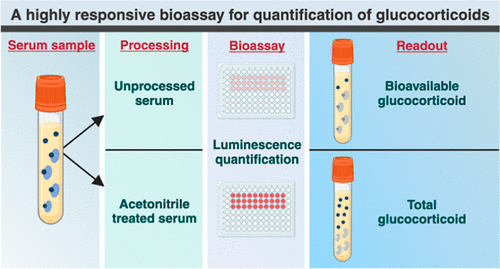
Highly Reliable Cell-Based Assay Enables Accurate Diagnosis of Endocrine Diseases
The conventional methods for measuring free cortisol, the body's stress hormone, from blood or saliva are quite demanding and require sample processing. The most common method, therefore, involves collecting... Read moreMolecular Diagnostics
view channel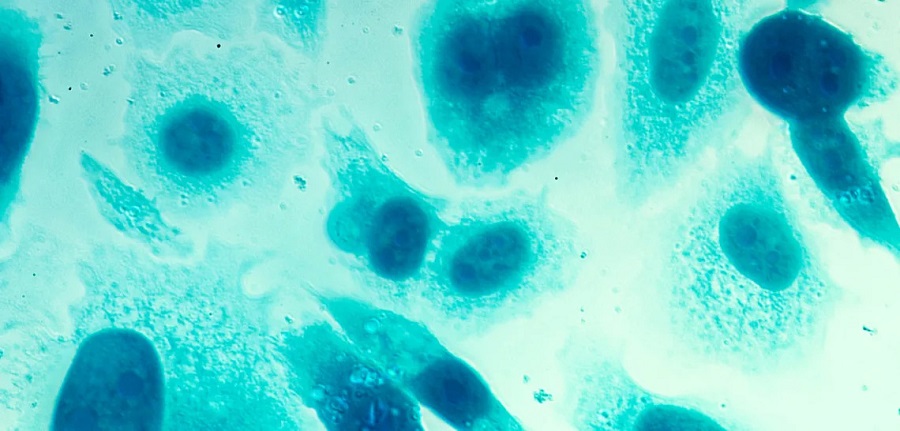
Blood Proteins Could Warn of Cancer Seven Years before Diagnosis
Two studies have identified proteins in the blood that could potentially alert individuals to the presence of cancer more than seven years before the disease is clinically diagnosed. Researchers found... Read more (1)_1.jpg)
Ultrasound-Aided Blood Testing Detects Cancer Biomarkers from Cells
Ultrasound imaging serves as a noninvasive method to locate and monitor cancerous tumors effectively. However, crucial details about the cancer, such as the specific types of cells and genetic mutations... Read moreHematology
view channel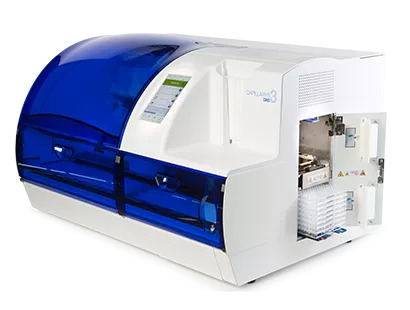
Next Generation Instrument Screens for Hemoglobin Disorders in Newborns
Hemoglobinopathies, the most widespread inherited conditions globally, affect about 7% of the population as carriers, with 2.7% of newborns being born with these conditions. The spectrum of clinical manifestations... Read more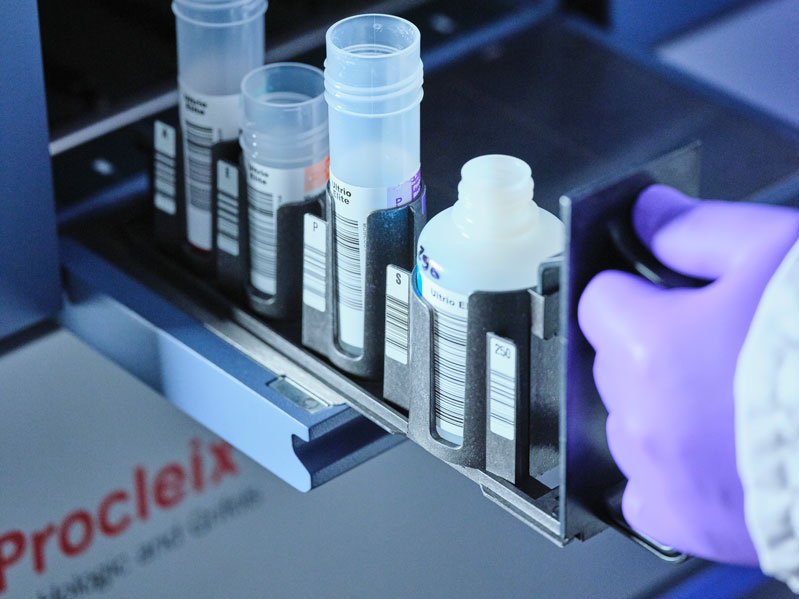
First 4-in-1 Nucleic Acid Test for Arbovirus Screening to Reduce Risk of Transfusion-Transmitted Infections
Arboviruses represent an emerging global health threat, exacerbated by climate change and increased international travel that is facilitating their spread across new regions. Chikungunya, dengue, West... Read more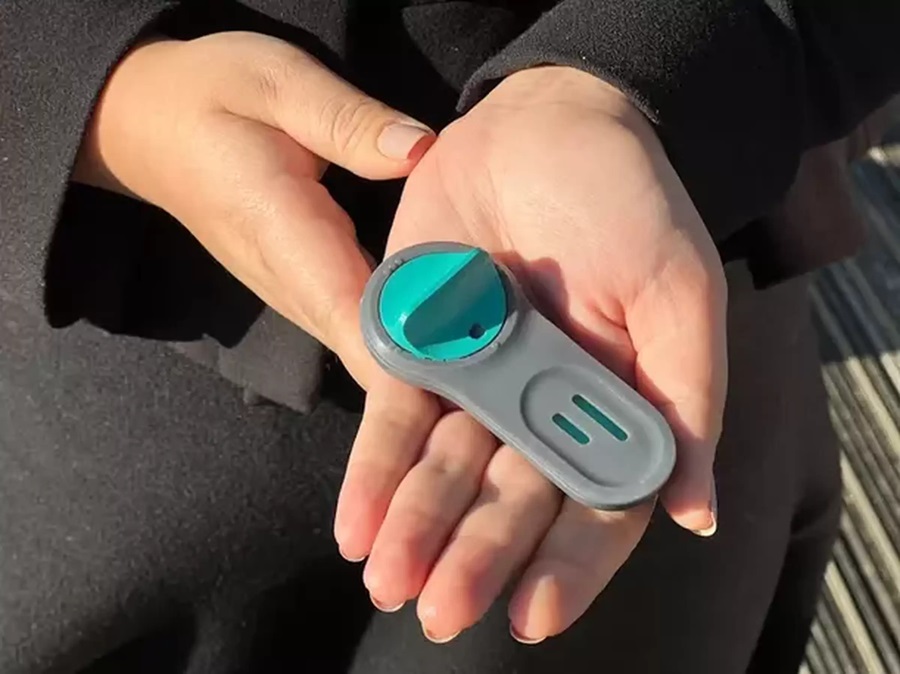
POC Finger-Prick Blood Test Determines Risk of Neutropenic Sepsis in Patients Undergoing Chemotherapy
Neutropenia, a decrease in neutrophils (a type of white blood cell crucial for fighting infections), is a frequent side effect of certain cancer treatments. This condition elevates the risk of infections,... Read more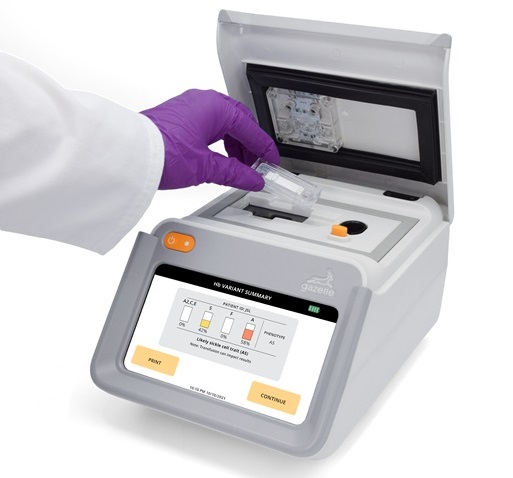
First Affordable and Rapid Test for Beta Thalassemia Demonstrates 99% Diagnostic Accuracy
Hemoglobin disorders rank as some of the most prevalent monogenic diseases globally. Among various hemoglobin disorders, beta thalassemia, a hereditary blood disorder, affects about 1.5% of the world's... Read moreImmunology
view channel.jpg)
AI Predicts Tumor-Killing Cells with High Accuracy
Cellular immunotherapy involves extracting immune cells from a patient's tumor, potentially enhancing their cancer-fighting capabilities through engineering, and then expanding and reintroducing them into the body.... Read more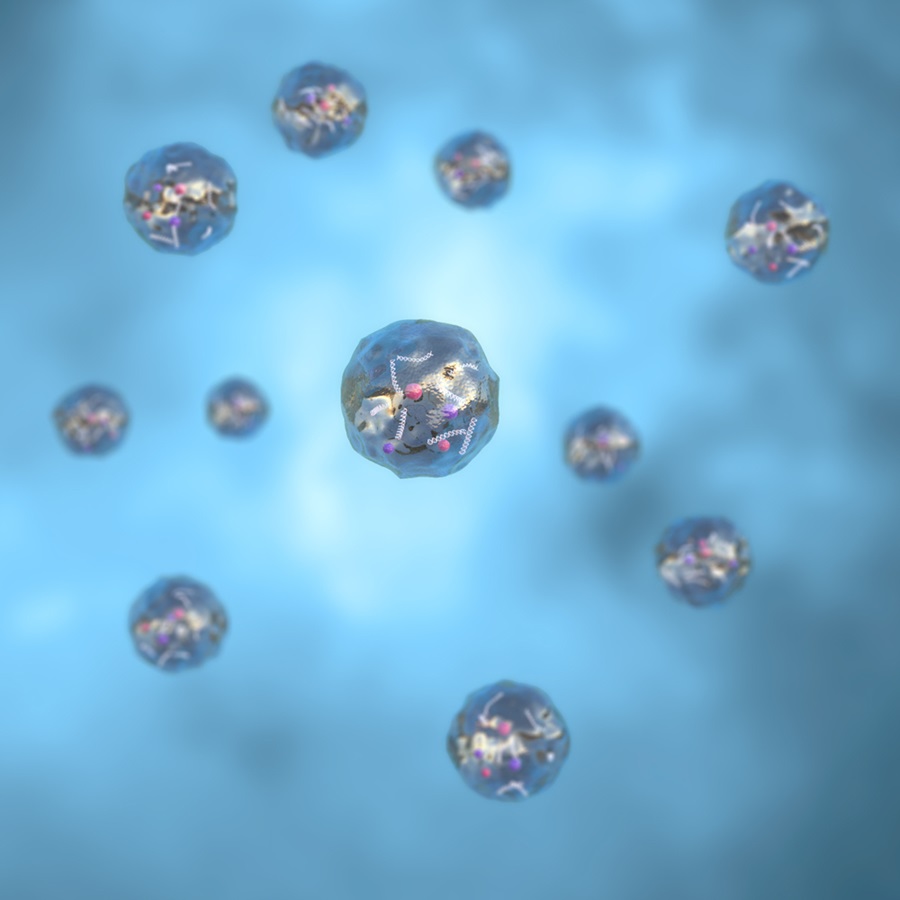
Diagnostic Blood Test for Cellular Rejection after Organ Transplant Could Replace Surgical Biopsies
Transplanted organs constantly face the risk of being rejected by the recipient's immune system which differentiates self from non-self using T cells and B cells. T cells are commonly associated with acute... Read more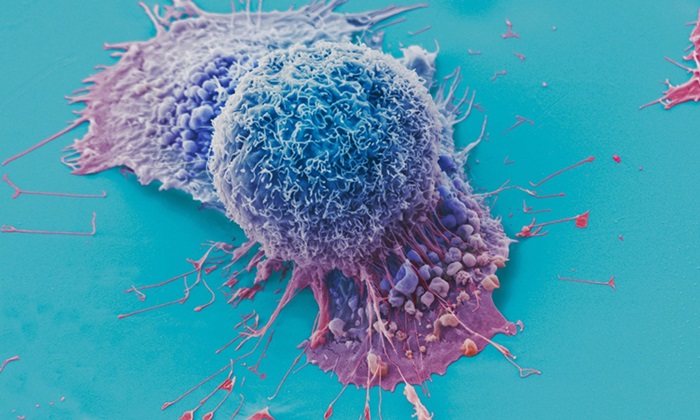
AI Tool Precisely Matches Cancer Drugs to Patients Using Information from Each Tumor Cell
Current strategies for matching cancer patients with specific treatments often depend on bulk sequencing of tumor DNA and RNA, which provides an average profile from all cells within a tumor sample.... Read more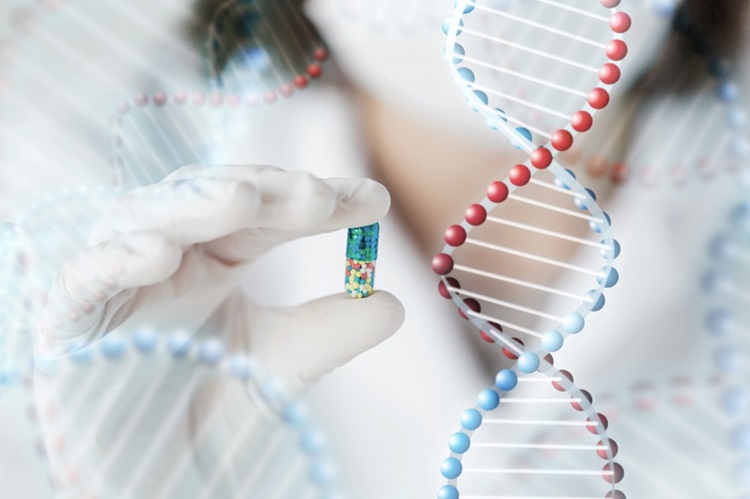
Genetic Testing Combined With Personalized Drug Screening On Tumor Samples to Revolutionize Cancer Treatment
Cancer treatment typically adheres to a standard of care—established, statistically validated regimens that are effective for the majority of patients. However, the disease’s inherent variability means... Read moreMicrobiology
view channel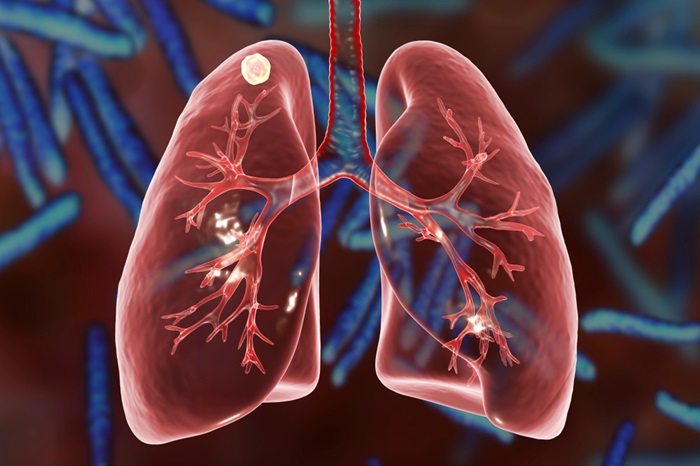
Integrated Solution Ushers New Era of Automated Tuberculosis Testing
Tuberculosis (TB) is responsible for 1.3 million deaths every year, positioning it as one of the top killers globally due to a single infectious agent. In 2022, around 10.6 million people were diagnosed... Read more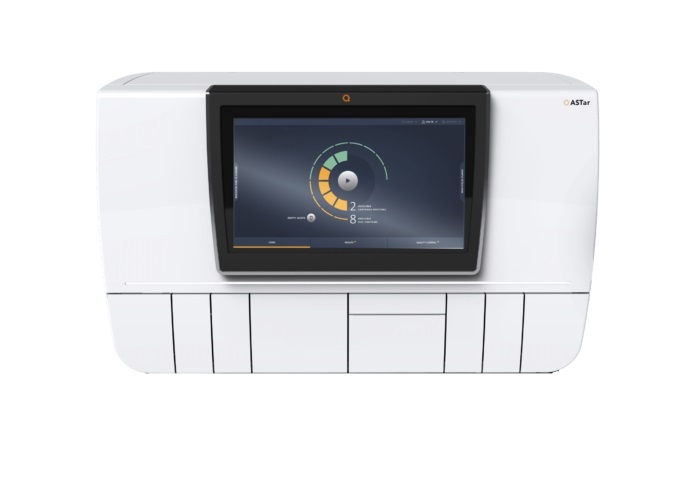
Automated Sepsis Test System Enables Rapid Diagnosis for Patients with Severe Bloodstream Infections
Sepsis affects up to 50 million people globally each year, with bacteraemia, formerly known as blood poisoning, being a major cause. In the United States alone, approximately two million individuals are... Read more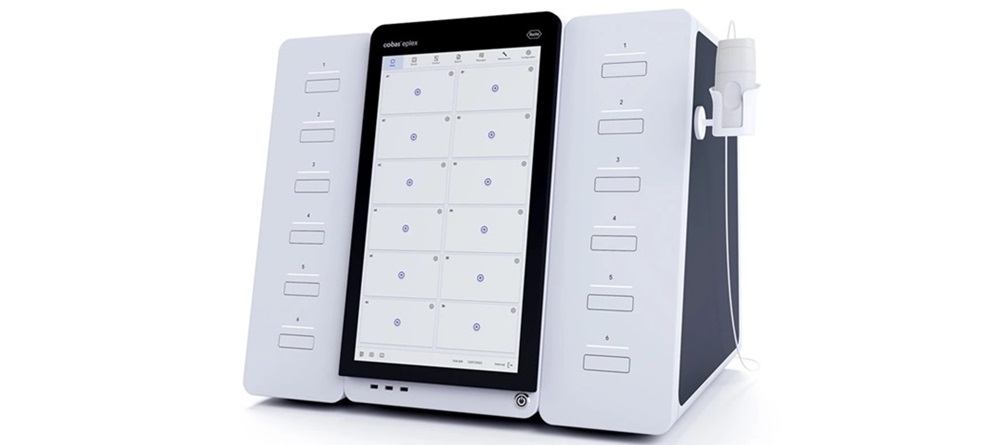
Enhanced Rapid Syndromic Molecular Diagnostic Solution Detects Broad Range of Infectious Diseases
GenMark Diagnostics (Carlsbad, CA, USA), a member of the Roche Group (Basel, Switzerland), has rebranded its ePlex® system as the cobas eplex system. This rebranding under the globally renowned cobas name... Read more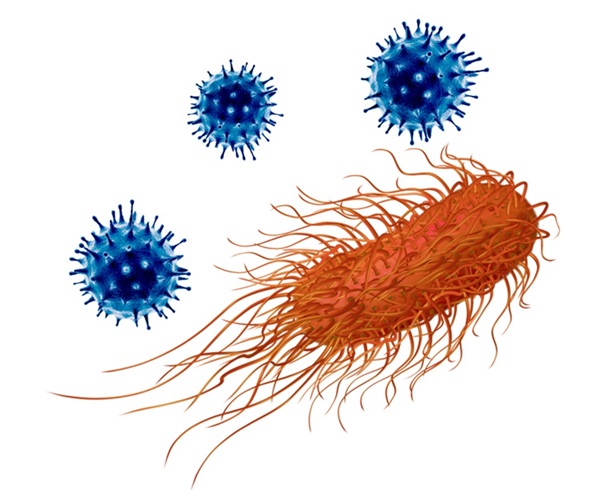
Clinical Decision Support Software a Game-Changer in Antimicrobial Resistance Battle
Antimicrobial resistance (AMR) is a serious global public health concern that claims millions of lives every year. It primarily results from the inappropriate and excessive use of antibiotics, which reduces... Read morePathology
view channel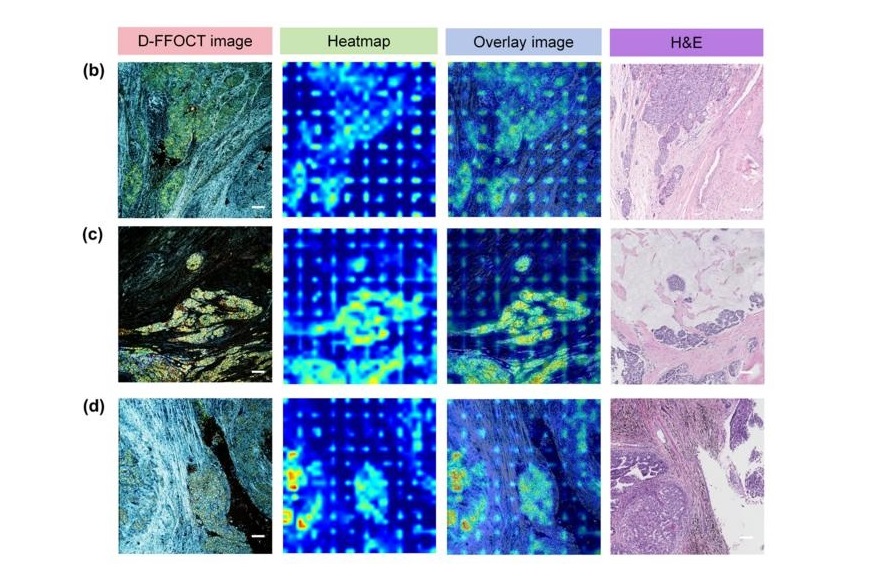
AI Integrated With Optical Imaging Technology Enables Rapid Intraoperative Diagnosis
Rapid and accurate intraoperative diagnosis is essential for tumor surgery as it guides surgical decisions with precision. Traditional intraoperative assessments, such as frozen sections based on H&E... Read more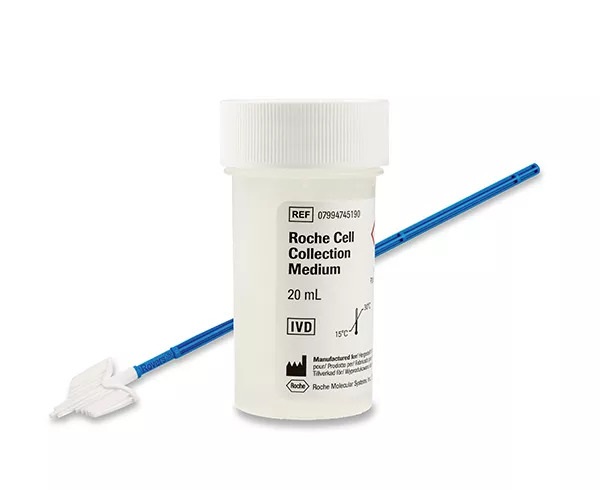
HPV Self-Collection Solution Improves Access to Cervical Cancer Testing
Annually, over 604,000 women across the world are diagnosed with cervical cancer, and about 342,000 die from this disease, which is preventable and primarily caused by the Human Papillomavirus (HPV).... Read more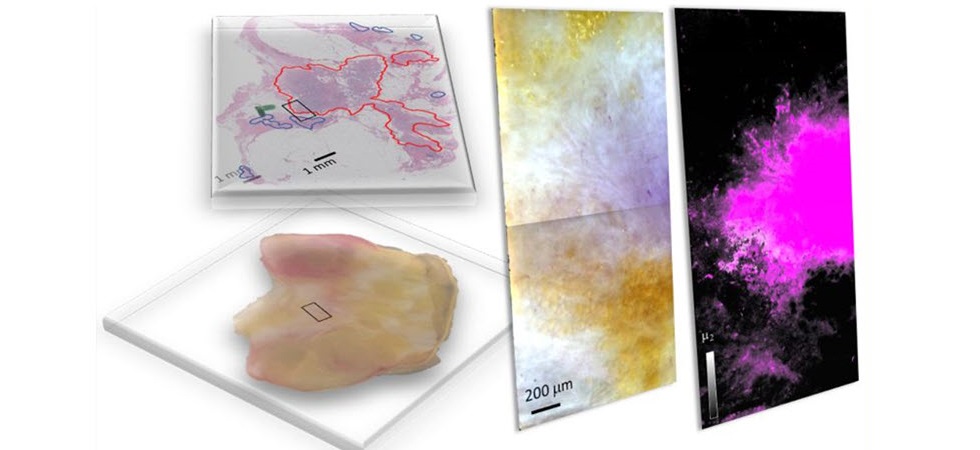
Hyperspectral Dark-Field Microscopy Enables Rapid and Accurate Identification of Cancerous Tissues
Breast cancer remains a major cause of cancer-related mortality among women. Breast-conserving surgery (BCS), also known as lumpectomy, is the removal of the cancerous lump and a small margin of surrounding tissue.... Read moreTechnology
view channel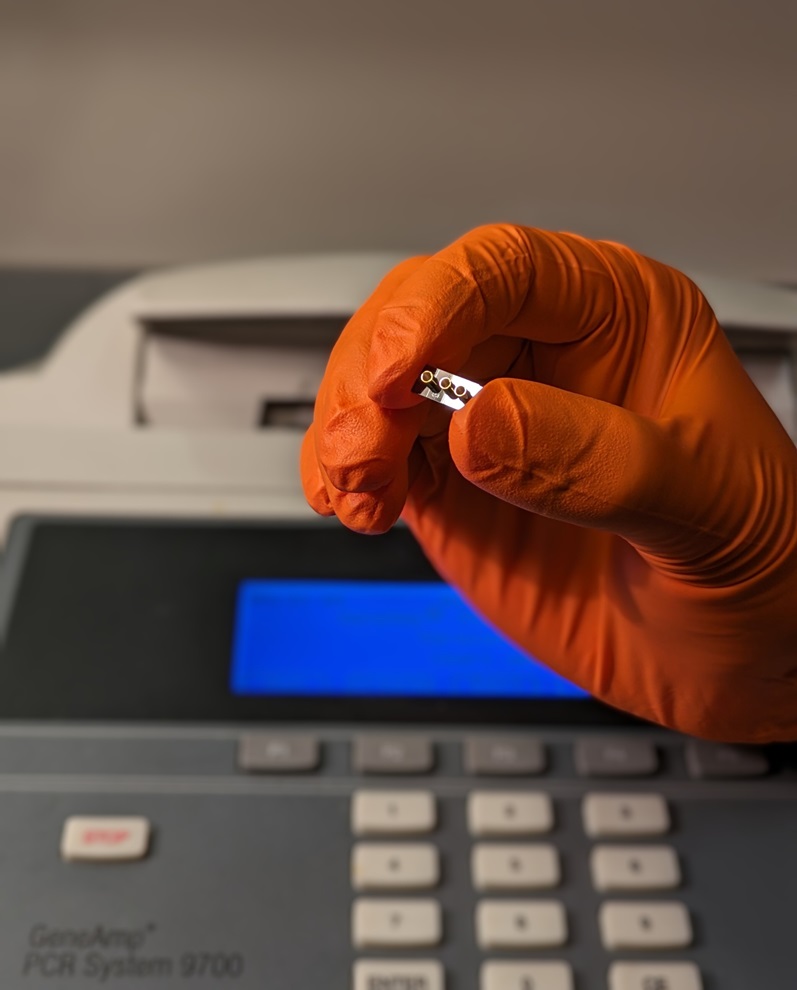
New Diagnostic System Achieves PCR Testing Accuracy
While PCR tests are the gold standard of accuracy for virology testing, they come with limitations such as complexity, the need for skilled lab operators, and longer result times. They also require complex... Read more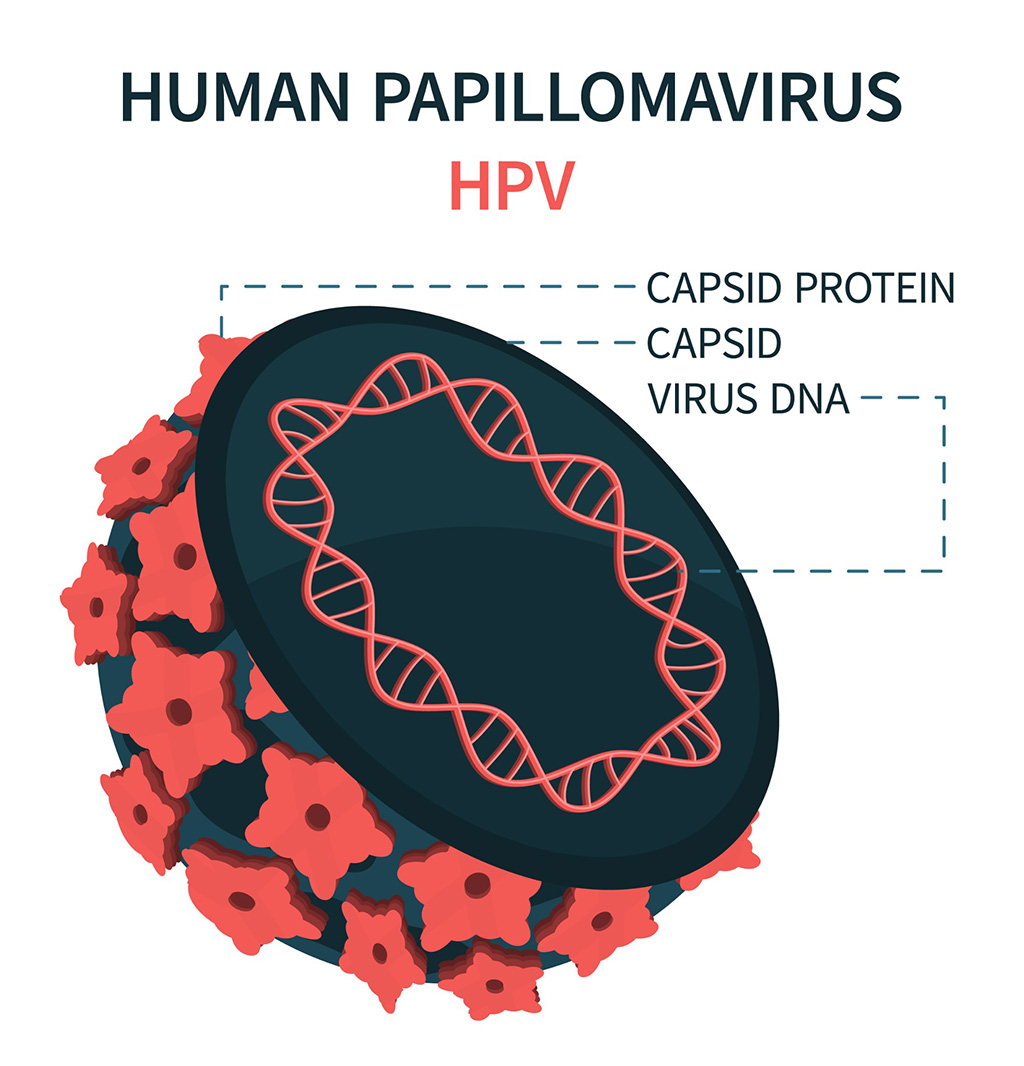
DNA Biosensor Enables Early Diagnosis of Cervical Cancer
Molybdenum disulfide (MoS2), recognized for its potential to form two-dimensional nanosheets like graphene, is a material that's increasingly catching the eye of the scientific community.... Read more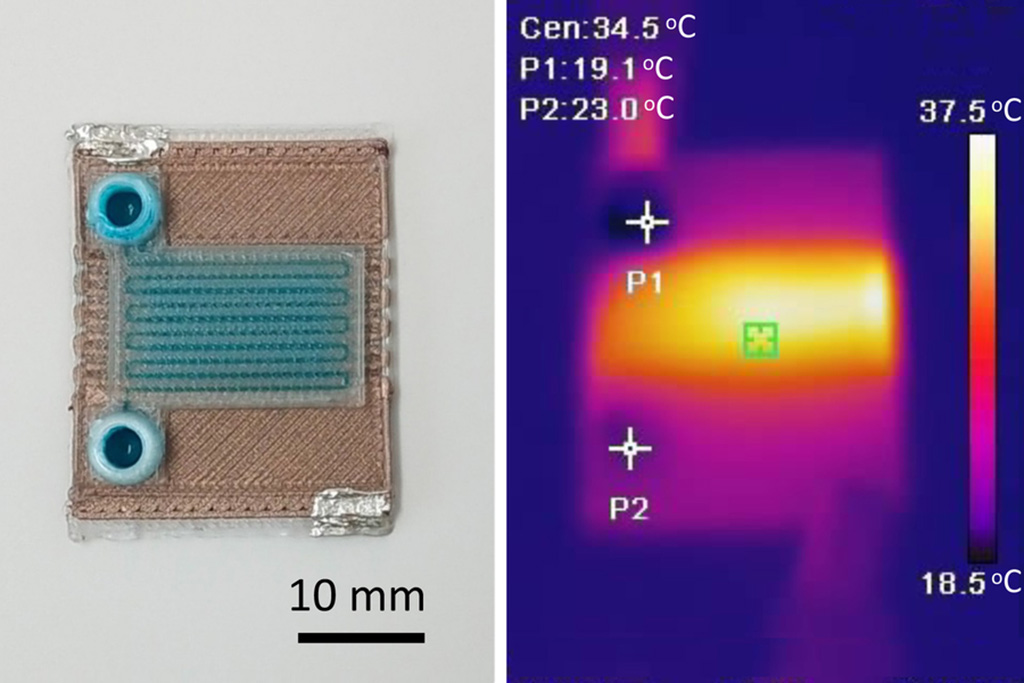
Self-Heating Microfluidic Devices Can Detect Diseases in Tiny Blood or Fluid Samples
Microfluidics, which are miniature devices that control the flow of liquids and facilitate chemical reactions, play a key role in disease detection from small samples of blood or other fluids.... Read more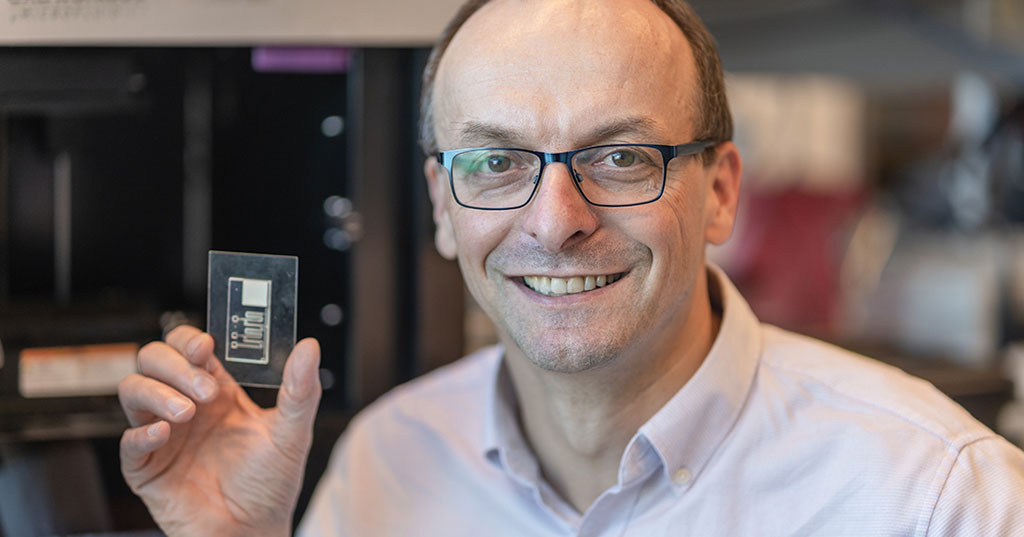
Breakthrough in Diagnostic Technology Could Make On-The-Spot Testing Widely Accessible
Home testing gained significant importance during the COVID-19 pandemic, yet the availability of rapid tests is limited, and most of them can only drive one liquid across the strip, leading to continued... Read moreIndustry
view channel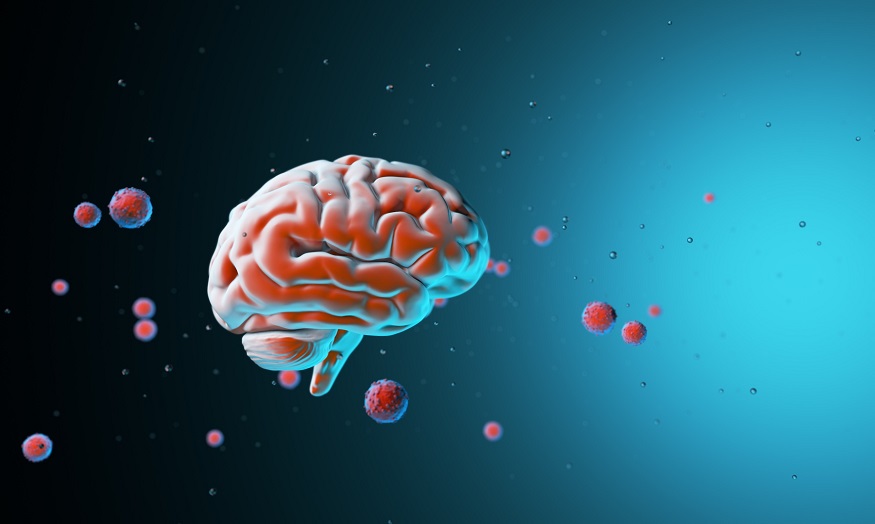
Danaher and Johns Hopkins University Collaborate to Improve Neurological Diagnosis
Unlike severe traumatic brain injury (TBI), mild TBI often does not show clear correlations with abnormalities detected through head computed tomography (CT) scans. Consequently, there is a pressing need... Read more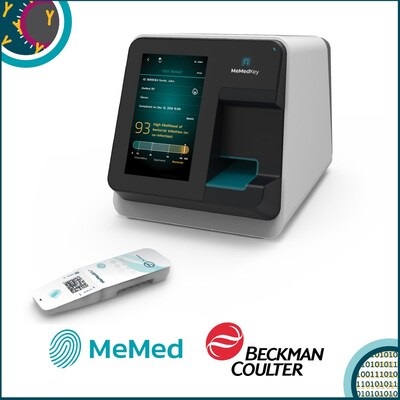
Beckman Coulter and MeMed Expand Host Immune Response Diagnostics Partnership
Beckman Coulter Diagnostics (Brea, CA, USA) and MeMed BV (Haifa, Israel) have expanded their host immune response diagnostics partnership. Beckman Coulter is now an authorized distributor of the MeMed... Read more_1.jpg)