AI Advancements Enable Leap into 3D Pathology
Posted on 10 May 2024
Human tissue is complex, intricate, and naturally three-dimensional. However, the thin two-dimensional tissue slices commonly used by pathologists to diagnose diseases provide only a limited view of the tissue's full complexity. As a result, there is a growing trend in pathology towards the examination of tissue in its three-dimensional form. Unfortunately, 3D pathology datasets can contain vastly more data than their 2D counterparts, rendering manual analysis impractical. Now, researchers have developed new, deep-learning models capable of utilizing 3D pathology datasets to predict clinical outcomes.
Tripath, developed by researchers from Mass General Brigham (Somerville, MA, USA) and their collaborators, aims to bridge the computational challenges of processing 3D tissue and predicting outcomes based on 3D morphological features. In their study, the team utilized two 3D high-resolution imaging techniques to capture images of curated prostate cancer specimens. These models were trained to assess the risk of prostate cancer recurrence using volumetric human tissue biopsies.
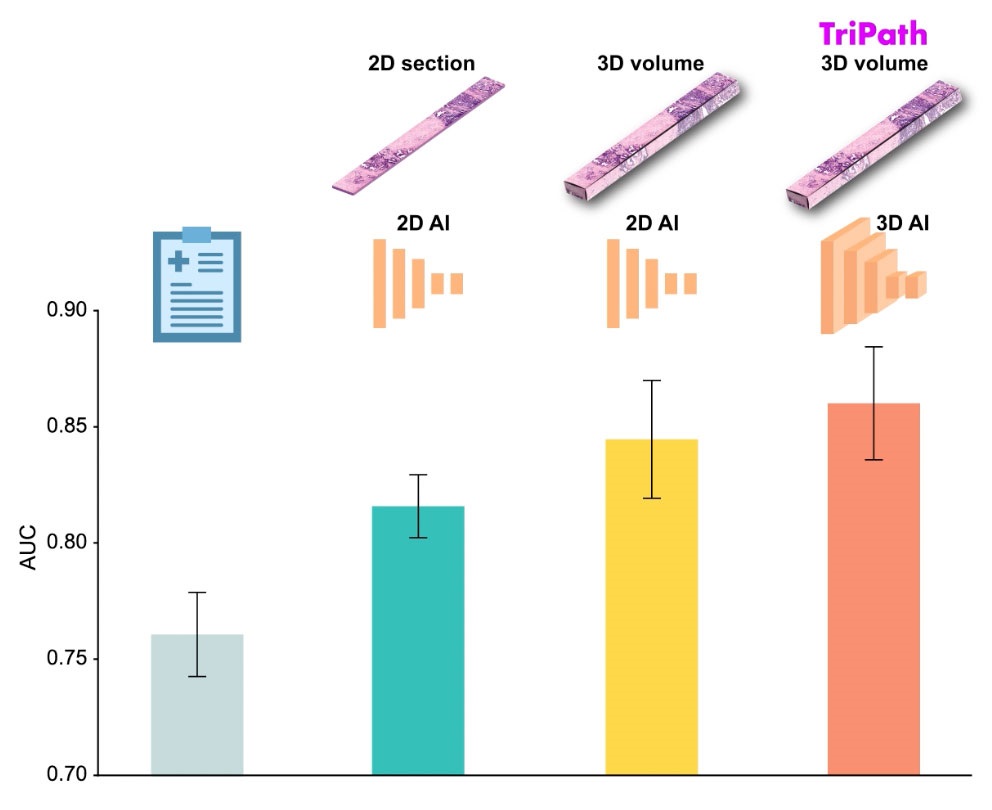
Tripath has demonstrated superior performance compared to traditional pathologists and has outperformed existing deep learning models that rely on 2D morphology and thin tissue slices, by comprehensively capturing 3D morphologies from the entire tissue volume. While further validation in larger datasets is necessary before this innovative approach can advance to clinical application, the research team remains optimistic about its potential to enhance clinical decision-making.
“Our approach underscores the importance of comprehensively analyzing the whole volume of a tissue sample for accurate patient risk prediction, which is the hallmark of the models we developed and only possible with the 3D pathology paradigm,” said lead author Andrew H. Song, PhD, of the Division of Computational Pathology in the Department of Pathology at Mass General Brigham.
Related Links:
Mass General Brigham