Prostate Cancer Identified By Profiling Immune Cell Subsets
By LabMedica International staff writers Posted on 19 Aug 2020 |
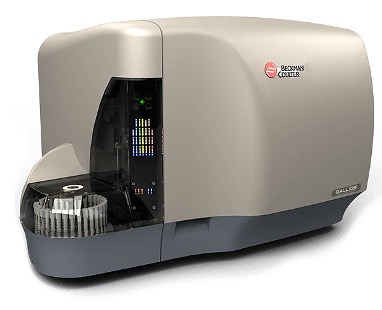
The Gallios 10-color/3-laser flow cytometer (Photo courtesy of Beckman Coulter).
With an estimated 1.8 million new cases in 2018 alone, prostate cancer is the fourth most common cancer in the world. Diagnosing the disease early increases the chances of survival, but this cancer remains difficult to detect.
The best diagnostic test currently available measures the blood level of a protein called the prostate-specific antigen (PSA for short). Heightened amounts of PSA may mean that the patient has cancer, but 15% of individuals with prostate cancer have normal levels of the protein.
A team of scientists at Nottingham Trent University (Nottingham, UK) and their colleagues obtained peripheral blood samples from individuals suspected of having prostate cancer that attended the Urology Clinic at Leicester General Hospital (Leicester, UK) between 24th October 2012 and 15th August 2014. Peripheral blood (60 mL) was collected from all patients using standard clinical procedures, and the peripheral blood mononuclear cell (PBMC) fraction was harvested. The PBMCs were processed and incubated with a monoclonal antibody (mAb) panel. Data on viable cells were acquired within 1 hour using a Gallios 10-color/3-laser flow cytometer (Beckman Coulter, Indianapolis, IN, USA).
The team collected and examined the natural killer cells of 72 participants with slightly elevated PSA levels and no other symptoms. Amongst these, 31 individuals had prostate cancer and 41 were healthy. These biological data were then used to produce computer models that could detect the presence of the disease, as well as assess its severity. The algorithms were developed using machine learning, where previous patient information is used to make prediction on new data.
Statistical and computational methods identified a panel of eight phenotypic features (CD56dimCD16high, CD56+DNAM−1−, CD56+LAIR−1+, CD56+LAIR−1−, CD56brightCD8+, CD56+NKp30+, CD56+NKp30−, CD56+NKp46+) that, when incorporated into an Ensemble machine learning prediction model, distinguished between the presence of benign prostate disease and prostate cancer. The machine learning model was then adapted to predict the D’Amico Risk Classification using data from 54 patients with prostate cancer and was shown to accurately differentiate between the presence of low-/intermediate-risk disease and high-risk disease without the need for additional clinical data.
The authors concluded that their study resulted in a new detection tool which was 12.5% more accurate than the PSA test in detecting prostate cancer; and in a detection tool that was 99% accurate in predicting the risk of the disease (in terms of clinical significance) in individuals with prostate cancer. This simple blood test has the potential to transform prostate cancer diagnostics. The study was published on July 28, 2020 in the journal eLife.
Related Links:
Nottingham Trent University
Leicester General Hospital
Beckman Coulter
The best diagnostic test currently available measures the blood level of a protein called the prostate-specific antigen (PSA for short). Heightened amounts of PSA may mean that the patient has cancer, but 15% of individuals with prostate cancer have normal levels of the protein.
A team of scientists at Nottingham Trent University (Nottingham, UK) and their colleagues obtained peripheral blood samples from individuals suspected of having prostate cancer that attended the Urology Clinic at Leicester General Hospital (Leicester, UK) between 24th October 2012 and 15th August 2014. Peripheral blood (60 mL) was collected from all patients using standard clinical procedures, and the peripheral blood mononuclear cell (PBMC) fraction was harvested. The PBMCs were processed and incubated with a monoclonal antibody (mAb) panel. Data on viable cells were acquired within 1 hour using a Gallios 10-color/3-laser flow cytometer (Beckman Coulter, Indianapolis, IN, USA).
The team collected and examined the natural killer cells of 72 participants with slightly elevated PSA levels and no other symptoms. Amongst these, 31 individuals had prostate cancer and 41 were healthy. These biological data were then used to produce computer models that could detect the presence of the disease, as well as assess its severity. The algorithms were developed using machine learning, where previous patient information is used to make prediction on new data.
Statistical and computational methods identified a panel of eight phenotypic features (CD56dimCD16high, CD56+DNAM−1−, CD56+LAIR−1+, CD56+LAIR−1−, CD56brightCD8+, CD56+NKp30+, CD56+NKp30−, CD56+NKp46+) that, when incorporated into an Ensemble machine learning prediction model, distinguished between the presence of benign prostate disease and prostate cancer. The machine learning model was then adapted to predict the D’Amico Risk Classification using data from 54 patients with prostate cancer and was shown to accurately differentiate between the presence of low-/intermediate-risk disease and high-risk disease without the need for additional clinical data.
The authors concluded that their study resulted in a new detection tool which was 12.5% more accurate than the PSA test in detecting prostate cancer; and in a detection tool that was 99% accurate in predicting the risk of the disease (in terms of clinical significance) in individuals with prostate cancer. This simple blood test has the potential to transform prostate cancer diagnostics. The study was published on July 28, 2020 in the journal eLife.
Related Links:
Nottingham Trent University
Leicester General Hospital
Beckman Coulter
Latest Immunology News
- Stem Cell Test Predicts Treatment Outcome for Patients with Platinum-Resistant Ovarian Cancer
- Machine Learning-Enabled Blood Test Predicts Immunotherapy Response in Lymphoma Patients
- Post-Treatment Blood Test Could Inform Future Cancer Therapy Decisions
- Cerebrospinal Fluid Test Predicts Dangerous Side Effect of Cancer Treatment
- New Test Measures Preterm Infant Immunity Using Only Two Drops of Blood
- Simple Blood Test Could Help Choose Better Treatments for Patients with Recurrent Endometrial Cancer
- Novel Analytical Method Tracks Progression of Autoimmune Diseases
- 3D Bioprinted Gastric Cancer Model Uses Patient-Derived Tissue Fragments to Predict Drug Response
- Blood Test for Fungal Infections Could End Invasive Tissue Biopsies
- Cutting-Edge Microscopy Technology Enables Tailored Rheumatology Therapies
- New Discovery in Blood Immune Cells Paves Way for Parkinson's Disease Diagnostic Test
- AI Tool Uses Routine Blood Tests to Predict Immunotherapy Response for Various Cancers
- Blood Test Can Predict How Long Vaccine Immunity Will Last
- Microfluidic Chip-Based Device to Measure Viral Immunity
- Simple Blood Test Could Detect Drug Resistance in Ovarian Cancer Patients
Channels
Clinical Chemistry
view channel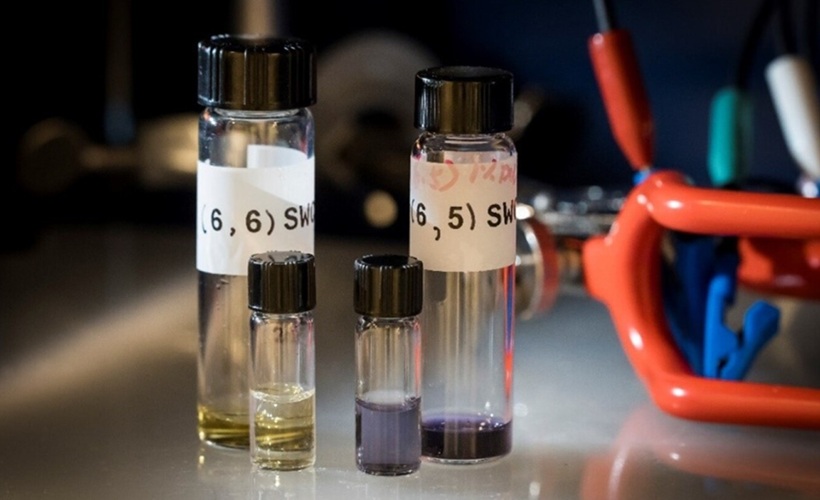
Carbon Nanotubes Help Build Highly Accurate Sensors for Continuous Health Monitoring
Current sensors can measure various health indicators, such as blood glucose levels, in the body. However, there is a need to develop more accurate and sensitive sensor materials that can detect lower... Read more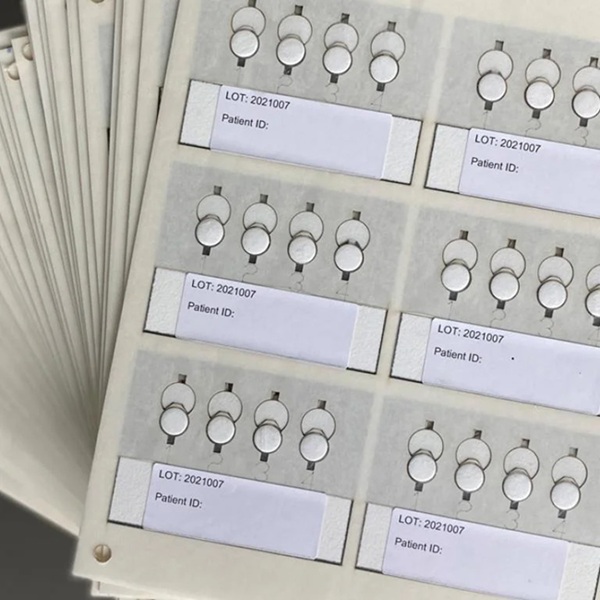
Paper-Based Device Boosts HIV Test Accuracy from Dried Blood Samples
In regions where access to clinics for routine blood tests presents financial and logistical obstacles, HIV patients are increasingly able to collect and send a drop of blood using paper-based devices... Read moreMolecular Diagnostics
view channel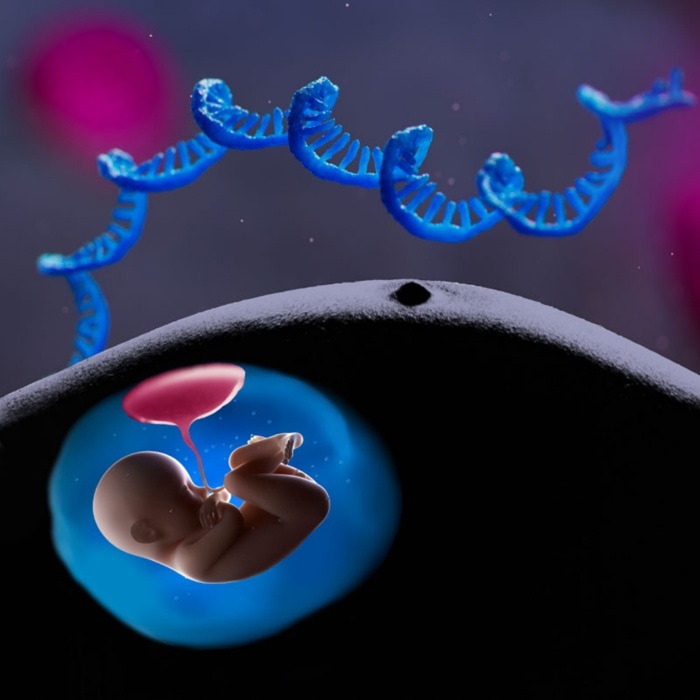
RNA-Based Blood Test Detects Preeclampsia Risk Months Before Symptoms
Preeclampsia remains a major cause of maternal morbidity and mortality, as well as preterm births. Despite current guidelines that aim to identify pregnant women at increased risk of preeclampsia using... Read more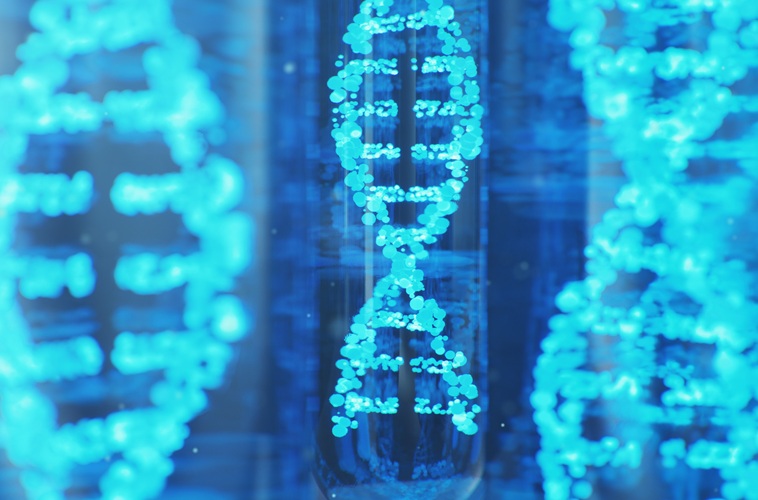
First Of Its Kind Test Uses microRNAs to Predict Toxicity from Cancer Therapy
Many men with early-stage prostate cancer receive stereotactic body radiotherapy (SBRT), a highly precise form of radiation treatment that is completed in just five sessions. Compared to traditional radiation,... Read more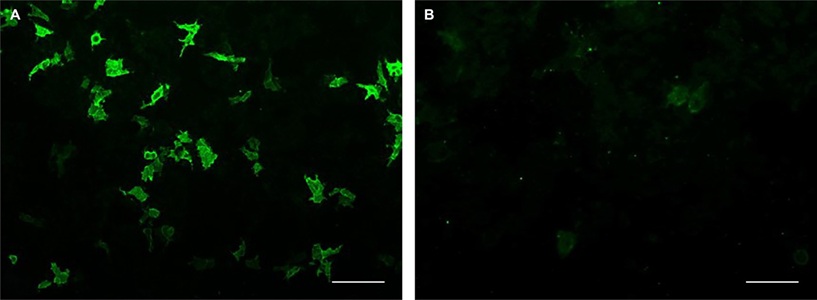
Novel Cell-Based Assay Provides Sensitive and Specific Autoantibody Detection in Demyelination
Anti-myelin-associated glycoprotein (MAG) antibodies serve as markers for an autoimmune demyelinating disorder that affects the peripheral nervous system, leading to sensory impairment. Anti-MAG-IgM antibodies... Read moreHematology
view channel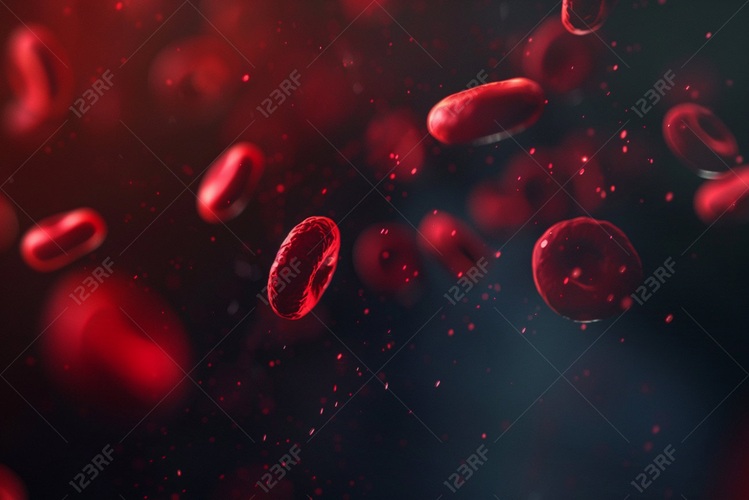
New Scoring System Predicts Risk of Developing Cancer from Common Blood Disorder
Clonal cytopenia of undetermined significance (CCUS) is a blood disorder commonly found in older adults, characterized by mutations in blood cells and a low blood count, but without any obvious cause or... Read more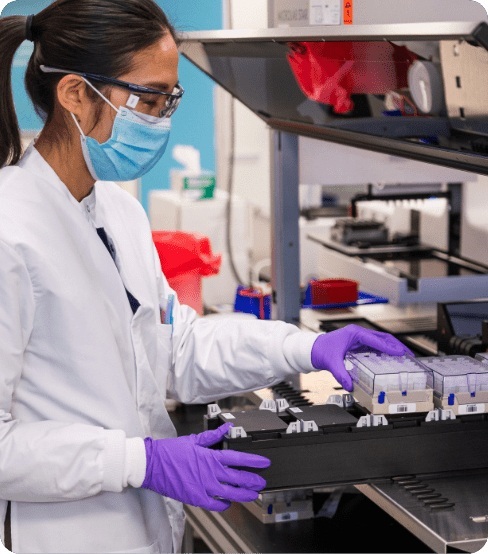
Non-Invasive Prenatal Test for Fetal RhD Status Demonstrates 100% Accuracy
In the United States, approximately 15% of pregnant individuals are RhD-negative. However, in about 40% of these cases, the fetus is also RhD-negative, making the administration of RhoGAM unnecessary.... Read moreMicrobiology
view channel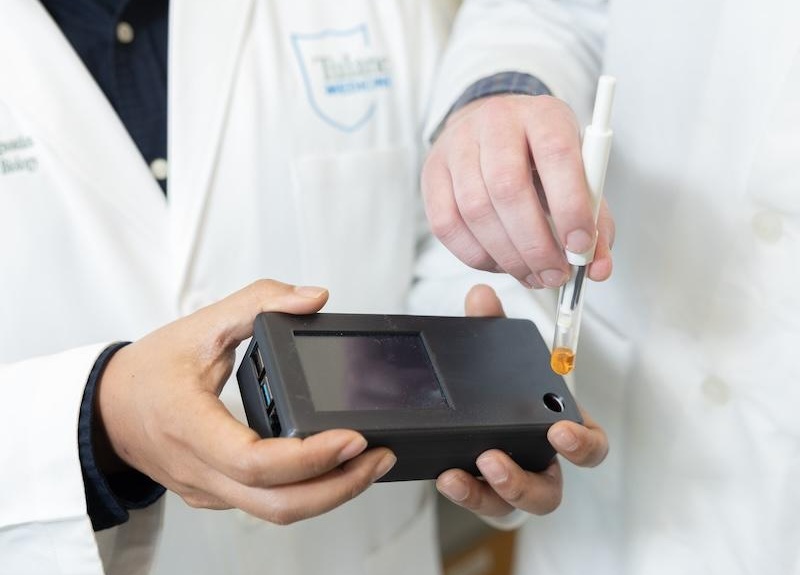
Handheld Device Deliver Low-Cost TB Results in Less Than One Hour
Tuberculosis (TB) remains the deadliest infectious disease globally, affecting an estimated 10 million people annually. In 2021, about 4.2 million TB cases went undiagnosed or unreported, mainly due to... Read more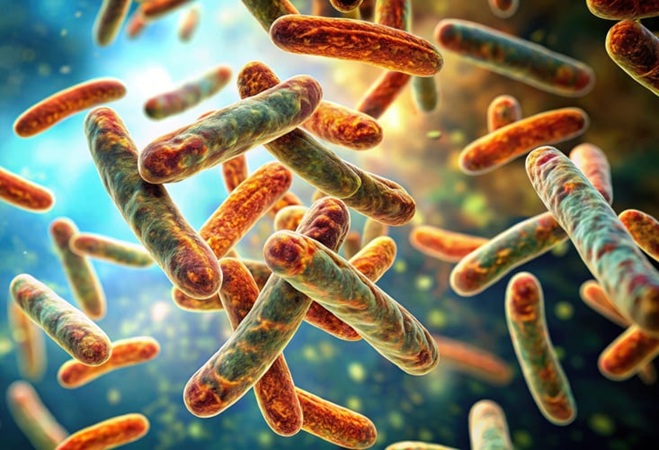
New AI-Based Method Improves Diagnosis of Drug-Resistant Infections
Drug-resistant infections, particularly those caused by deadly bacteria like tuberculosis and staphylococcus, are rapidly emerging as a global health emergency. These infections are more difficult to treat,... Read more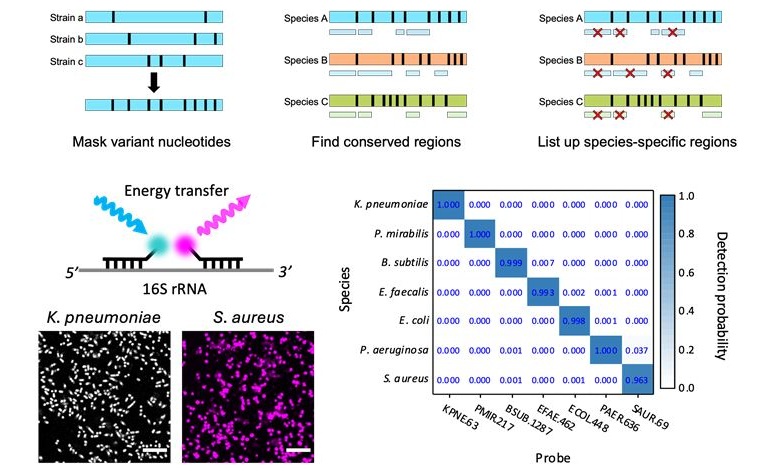
Breakthrough Diagnostic Technology Identifies Bacterial Infections with Almost 100% Accuracy within Three Hours
Rapid and precise identification of pathogenic microbes in patient samples is essential for the effective treatment of acute infectious diseases, such as sepsis. The fluorescence in situ hybridization... Read morePathology
view channel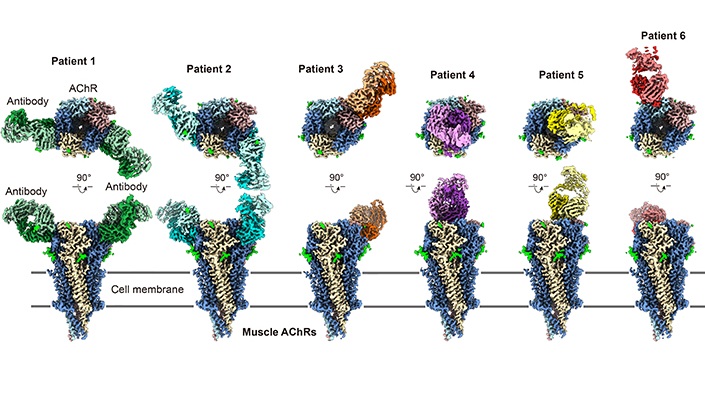
Advanced Imaging Reveals Mechanisms Causing Autoimmune Disease
Myasthenia gravis, an autoimmune disease, leads to muscle weakness that can affect a range of muscles, including those needed for basic actions like blinking, smiling, or moving. Researchers have long... Read more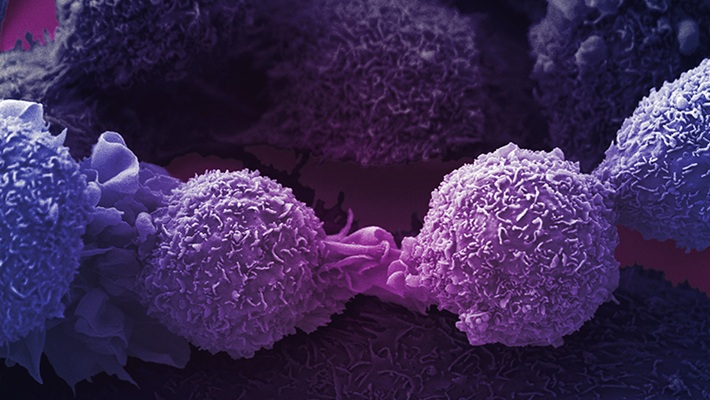
AI Model Effectively Predicts Patient Outcomes in Common Lung Cancer Type
Lung adenocarcinoma, the most common form of non-small cell lung cancer (NSCLC), typically adopts one of six distinct growth patterns, often combining multiple patterns within a single tumor.... Read moreTechnology
view channel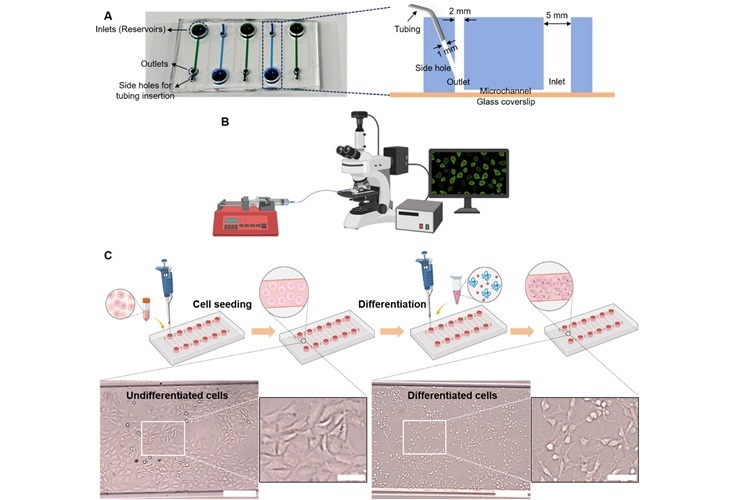
Pain-On-A-Chip Microfluidic Device Determines Types of Chronic Pain from Blood Samples
Chronic pain is a widespread condition that remains difficult to manage, and existing clinical methods for its treatment rely largely on self-reporting, which can be subjective and especially problematic... Read more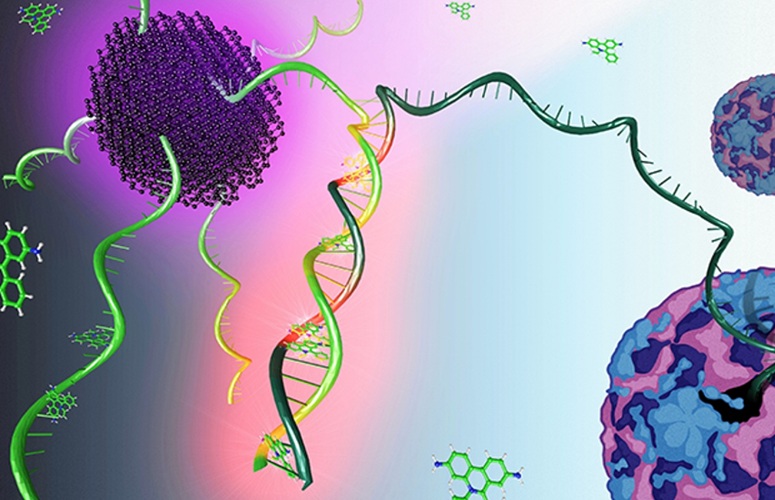
Innovative, Label-Free Ratiometric Fluorosensor Enables More Sensitive Viral RNA Detection
Viruses present a major global health risk, as demonstrated by recent pandemics, making early detection and identification essential for preventing new outbreaks. While traditional detection methods are... Read moreIndustry
view channel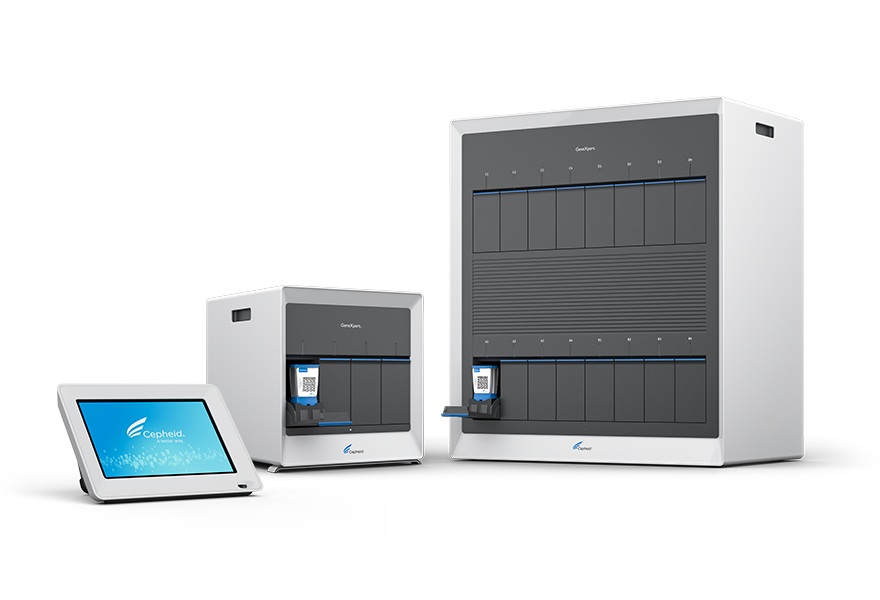
Cepheid and Oxford Nanopore Technologies Partner on Advancing Automated Sequencing-Based Solutions
Cepheid (Sunnyvale, CA, USA), a leading molecular diagnostics company, and Oxford Nanopore Technologies (Oxford, UK), the company behind a new generation of sequencing-based molecular analysis technologies,... Read more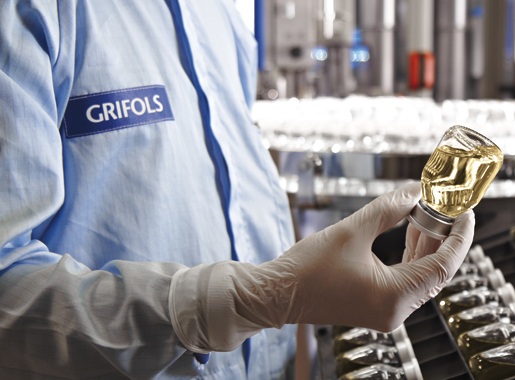
Grifols and Tecan’s IBL Collaborate on Advanced Biomarker Panels
Grifols (Barcelona, Spain), one of the world’s leading producers of plasma-derived medicines and innovative diagnostic solutions, is expanding its offer in clinical diagnostics through a strategic partnership... Read more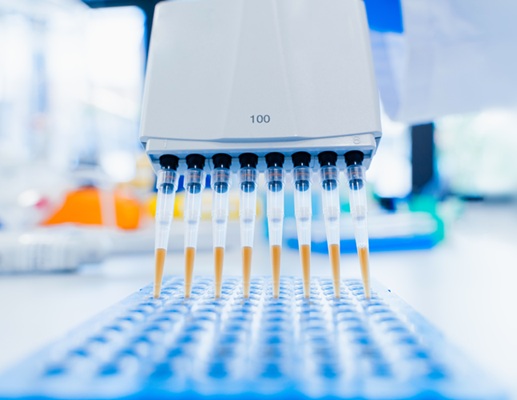