AI Model Effectively Predicts Patient Outcomes in Common Lung Cancer Type
Posted on 10 Apr 2025
Lung adenocarcinoma, the most common form of non-small cell lung cancer (NSCLC), typically adopts one of six distinct growth patterns, often combining multiple patterns within a single tumor. A global grading system developed by the International Association for the Study of Lung Cancer (IASLC) links these growth patterns to the likelihood of disease progression or recurrence. However, the presence of multiple pattern types within a tumor and the variation in how each pattern appears across different tumors complicates the task of determining a patient's prognosis. This complexity, coupled with the challenge of defining and quantifying these growth patterns, often leads to discrepancies in tumor grading among pathologists. As a result, inconsistent or suboptimal grading could result in patients receiving inadequate or inappropriate treatment, which might compromise their outcomes. Although previous studies have explored the use of deep learning models for classifying growth patterns in lung adenocarcinoma, these models have typically not considered the detailed morphological structure of the patterns, nor have they been capable of performing automated IASLC grading.
In response to this challenge, researchers at the Institute of Cancer Research (ICR, London, UK) have developed an artificial intelligence (AI)-based model designed to help pathologists grade lung cancer tumors and predict patient outcomes by analyzing tumor growth patterns, which can vary greatly among individuals. In a recent study, the ICR team demonstrated that the model, named ANORAK (pyrAmid pooliNg crOss stReam Attention networK), was able to predict disease-free survival (DFS), a critical measure of the length of time between treatment for lung adenocarcinoma and the return of symptoms or signs of the disease. In the long term, the model could assist clinicians in determining the most effective treatment strategies based on the predicted progression of cancer. This improved decision-making could ultimately lead to better patient outcomes, especially in light of recent advancements in cancer screening programs that have led to more early-stage lung cancer diagnoses, underscoring the need for enhanced treatment decisions. The research, conducted by ICR scientists, was published in Nature Cancer.
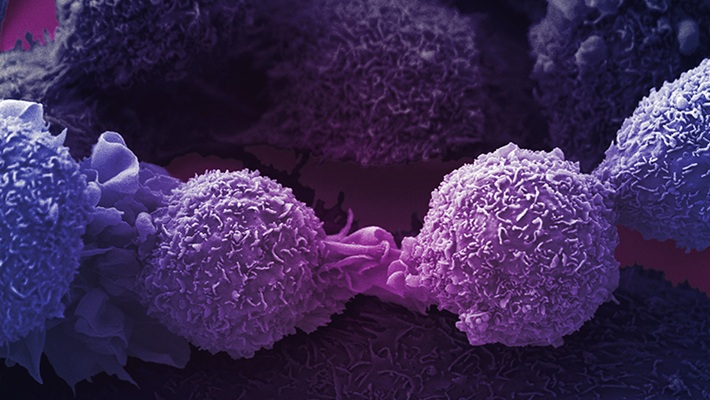
In this study, the researchers used ANORAK to assess six types of lung adenocarcinoma growth patterns at the pixel level. They applied the model to 5,540 tumor samples from diagnostic slides, which came from 1,372 patients with the disease. The model proved effective in enhancing patient risk stratification, showing that those with IASLC grade 1 or 2 tumors had significantly longer DFS than those with grade 3 tumors. To validate the AI grading, the researchers compared it to manual grading results from three pathologists. They found that ANORAK’s grading was consistent with the pathologists' assessments, even slightly outperforming them for one cohort of patients. By referencing previous studies, the team confirmed that the agreement between AI and manual grading on the predominant growth pattern of a tumor was comparable to the level of agreement typically seen between different pathologists.
The study concluded that AI grading adds significant prognostic value, especially in early-stage lung adenocarcinoma, where treatment decisions are often challenging. In the second phase of the study, the researchers examined four specific scenarios that are typically difficult for pathologists, including cases with multiple diagnostic slides per tumor and those with highly diversified growth patterns. ANORAK performed well in all four scenarios, demonstrating its potential to assist pathologists even in complex cases. Additionally, the researchers focused on the acinar pattern, the most common of the six growth patterns, using ANORAK to better understand its structures and shapes. They also identified correlations between different acinar subtypes and tumor characteristics, some of which were associated with poorer prognoses. Moving forward, the researchers plan to incorporate genetic data into their model to gain deeper insights into tumor progression and the influence of surrounding cells and tissues. The team also intends to test ANORAK on larger groups of early-stage lung adenocarcinoma patients to gather more evidence of its effectiveness.
“Diagnostic inaccuracies and variability among pathologists are longstanding issues in lung adenocarcinoma. Our study is the first to implement the IASLC grading system with an AI-powered tool and validate the prognostic values on two distinct cohorts,” said Dr. Xiaoxi Pan, then a Postdoctoral Training Fellow in ICR’s Computational Pathology and Integrative Genomics Group and first author. “Our AI method enables the precise and automated quantification of unique growth patterns within a tumor, thereby inferring the predominant pattern and grading. It has also identified previously undiscovered morphological and spatial features of certain tissues that were not achievable using existing algorithms or human observations.”
Related Links:
ICR