AI Analysis of Immune Cells Predicts Breast Cancer Prognosis
By LabMedica International staff writers Posted on 20 Nov 2024 |
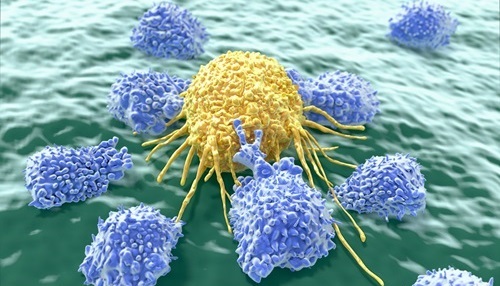
Tumor-infiltrating lymphocytes (TILs) are immune cells crucial in combating cancer. Their presence in a tumor indicates that the immune system is attempting to attack and eliminate cancer cells. TILs can be important indicators in predicting how patients with triple-negative breast cancer will respond to treatment and how the disease might progress. However, assessing these immune cells can yield inconsistent results. Artificial intelligence (AI) has the potential to standardize and automate this process, but proving its effectiveness for healthcare use has been challenging. Now, researchers have explored how different AI models can predict the prognosis of triple-negative breast cancer by analyzing specific immune cells within the tumor. This study, published in eClinicalMedicine, represents a significant step toward incorporating AI into cancer care to enhance patient outcomes.
Researchers at Karolinska Institutet (Stockholm, Sweden) tested ten different AI models to evaluate their ability to analyze tumor-infiltrating lymphocytes in tissue samples from patients with triple-negative breast cancer. The results revealed that the performance of the AI models varied, but eight out of the ten models demonstrated strong prognostic capability, meaning they could predict patient health outcomes with similar accuracy. Even models trained on smaller datasets showed promising results, suggesting that tumor-infiltrating lymphocytes are a reliable biomarker. The study highlights the need for large datasets to compare different AI models and validate their effectiveness before they can be used in clinical practice. Although the findings are promising, further validation is required.
“Our research highlights the importance of independent studies that mimic real clinical practice,” said Balazs Acs, researcher at the Department of Oncology-Pathology, Karolinska Institutet. “Only through such testing can we ensure that AI tools are reliable and effective for clinical use.”
Latest Pathology News
- AI Model Predicts Patient Response to Bladder Cancer Treatment
- New Laser-Based Method to Accelerate Cancer Diagnosis
- New AI Model Predicts Gene Variants’ Effects on Specific Diseases
- Powerful AI Tool Diagnoses Coeliac Disease from Biopsy Images with Over 97% Accuracy
- Pre-Analytical Conditions Influence Cell-Free MicroRNA Stability in Blood Plasma Samples
- 3D Cell Culture System Could Revolutionize Cancer Diagnostics
- Painless Technique Measures Glucose Concentrations in Solution and Tissue Via Sound Waves
- Skin-Based Test to Improve Diagnosis of Rare, Debilitating Neurodegenerative Disease
- Serum Uromodulin Could Indicate Acute Kidney Injury in COVID-19 Patients
- AI Model Reveals True Biological Age From Five Drops of Blood
- First-Of-Its-Kind AI Tool Visualizes Cell’s ‘Social Network’ To Treat Cancer
- New Test Diagnoses High-Risk Childhood Brain Tumors
- Informatics Solution Elevates Laboratory Efficiency and Patient Care
- Microfluidic Device Assesses Stickiness of Tumor Cells to Predict Cancer Spread
- New AI Tool Outperforms Previous Methods for Identifying Colorectal Cancer from Tissue Sample Analysis
- New Technique Predicts Aggressive Tumors Before They Metastasize
Channels
Clinical Chemistry
view channel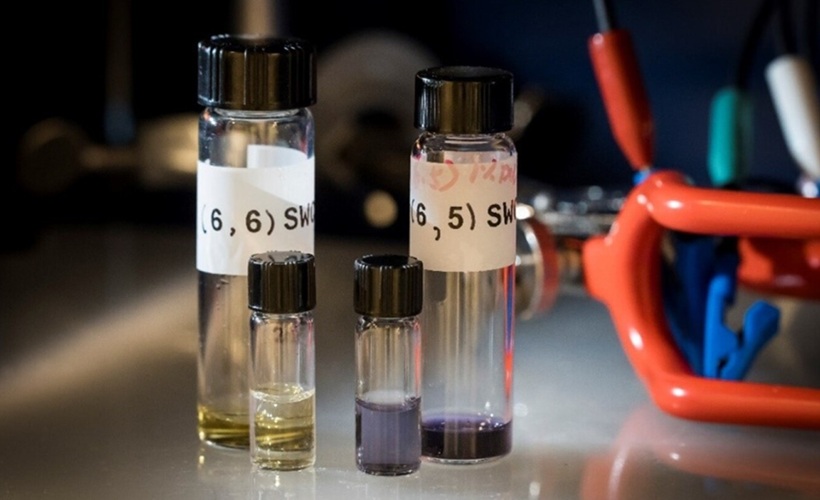
Carbon Nanotubes Help Build Highly Accurate Sensors for Continuous Health Monitoring
Current sensors can measure various health indicators, such as blood glucose levels, in the body. However, there is a need to develop more accurate and sensitive sensor materials that can detect lower... Read more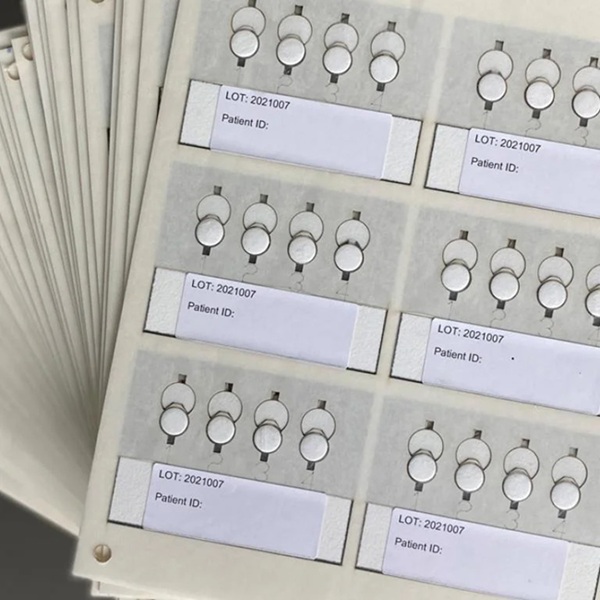
Paper-Based Device Boosts HIV Test Accuracy from Dried Blood Samples
In regions where access to clinics for routine blood tests presents financial and logistical obstacles, HIV patients are increasingly able to collect and send a drop of blood using paper-based devices... Read moreMolecular Diagnostics
view channel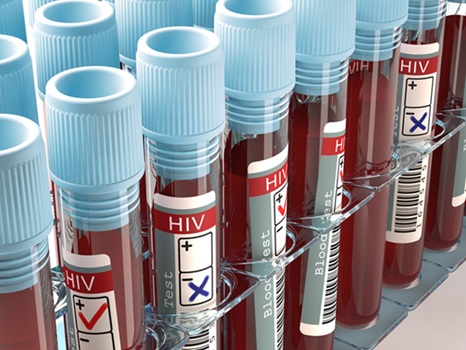
Novel Point-of-Care Technology Delivers Accurate HIV Results in Minutes
HIV diagnostic methods have traditionally relied on detecting HIV-specific antibodies, which typically appear weeks after infection. This delayed detection has hindered early diagnosis, complicating patient... Read more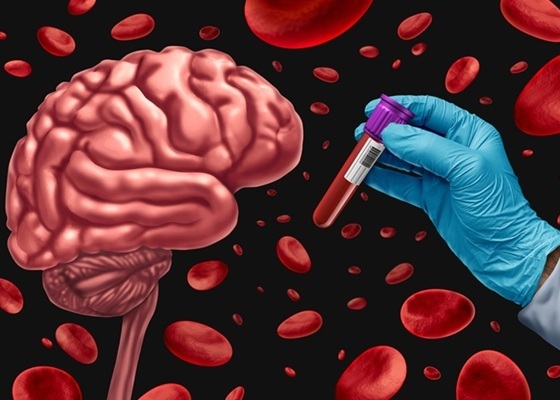
Blood Test Rules Out Future Dementia Risk
Previous studies have suggested that specific biomarkers, such as tau217, Neurofilament Light (NfL), and Glial Fibrillary Acidic Protein (GFAP), may be valuable for early dementia diagnosis.... Read moreHematology
view channel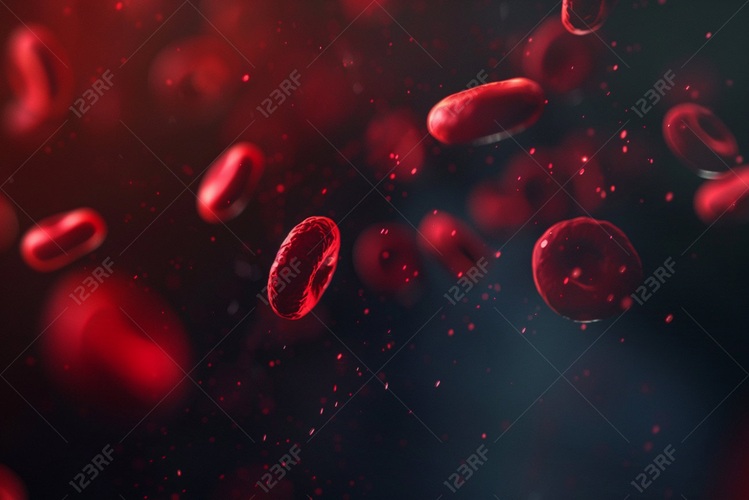
New Scoring System Predicts Risk of Developing Cancer from Common Blood Disorder
Clonal cytopenia of undetermined significance (CCUS) is a blood disorder commonly found in older adults, characterized by mutations in blood cells and a low blood count, but without any obvious cause or... Read more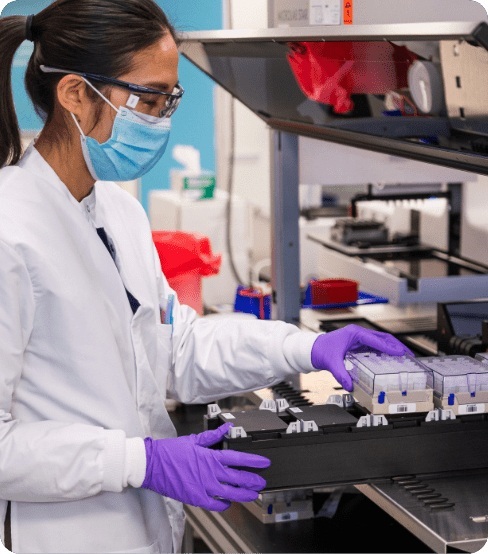
Non-Invasive Prenatal Test for Fetal RhD Status Demonstrates 100% Accuracy
In the United States, approximately 15% of pregnant individuals are RhD-negative. However, in about 40% of these cases, the fetus is also RhD-negative, making the administration of RhoGAM unnecessary.... Read moreImmunology
view channel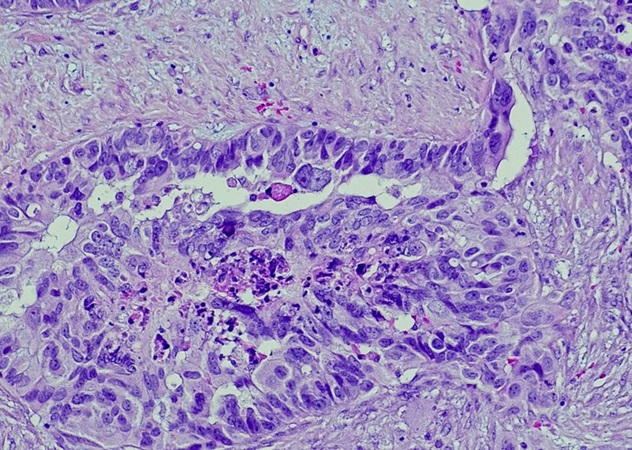
Stem Cell Test Predicts Treatment Outcome for Patients with Platinum-Resistant Ovarian Cancer
Epithelial ovarian cancer frequently responds to chemotherapy initially, but eventually, the tumor develops resistance to the therapy, leading to regrowth. This resistance is partially due to the activation... Read more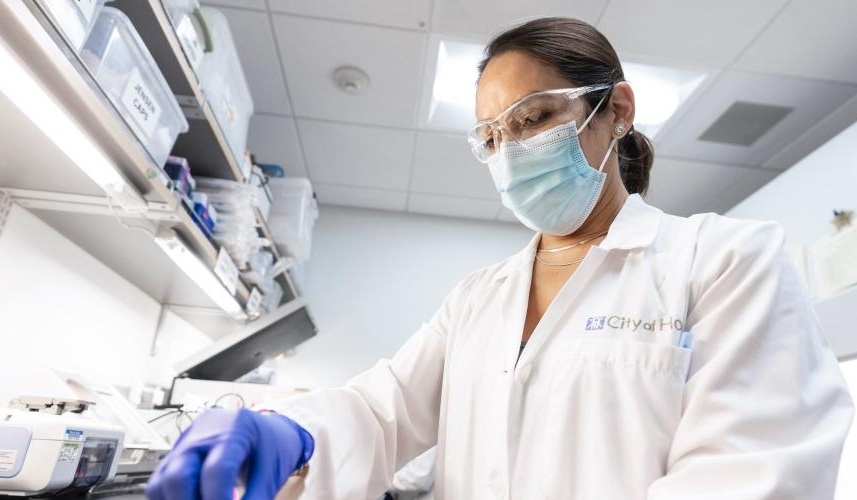
Machine Learning-Enabled Blood Test Predicts Immunotherapy Response in Lymphoma Patients
Chimeric antigen receptor (CAR) T-cell therapy has emerged as one of the most promising recent developments in the treatment of blood cancers. However, over half of non-Hodgkin lymphoma (NHL) patients... Read moreMicrobiology
view channel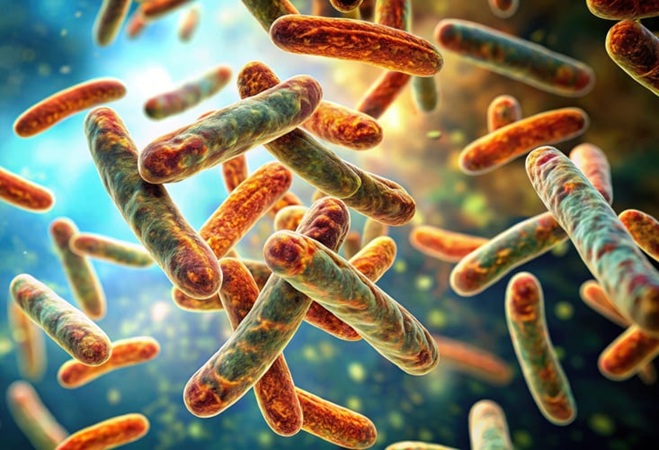
New AI-Based Method Improves Diagnosis of Drug-Resistant Infections
Drug-resistant infections, particularly those caused by deadly bacteria like tuberculosis and staphylococcus, are rapidly emerging as a global health emergency. These infections are more difficult to treat,... Read more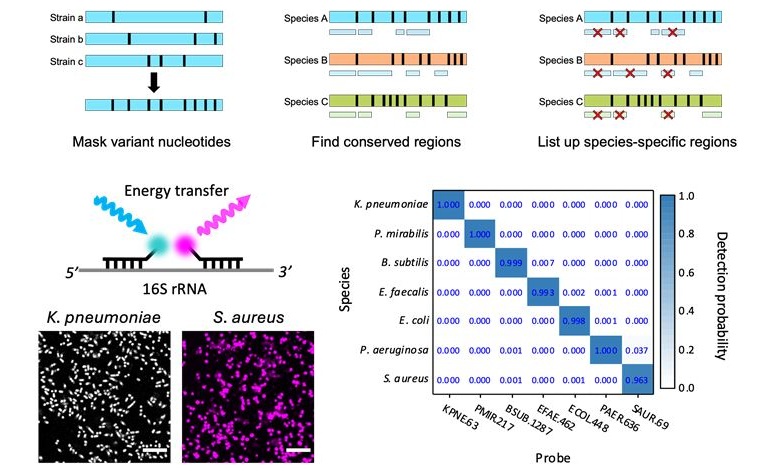
Breakthrough Diagnostic Technology Identifies Bacterial Infections with Almost 100% Accuracy within Three Hours
Rapid and precise identification of pathogenic microbes in patient samples is essential for the effective treatment of acute infectious diseases, such as sepsis. The fluorescence in situ hybridization... Read moreInnovative ID/AST System to Help Diagnose Infectious Diseases and Combat AMR
Each year, 11 million people across the world die of sepsis out of which 1.3 million deaths are due to antibiotic-resistant bacteria. The burden of antimicrobial resistance (AMR) continues to weigh heavily,... Read more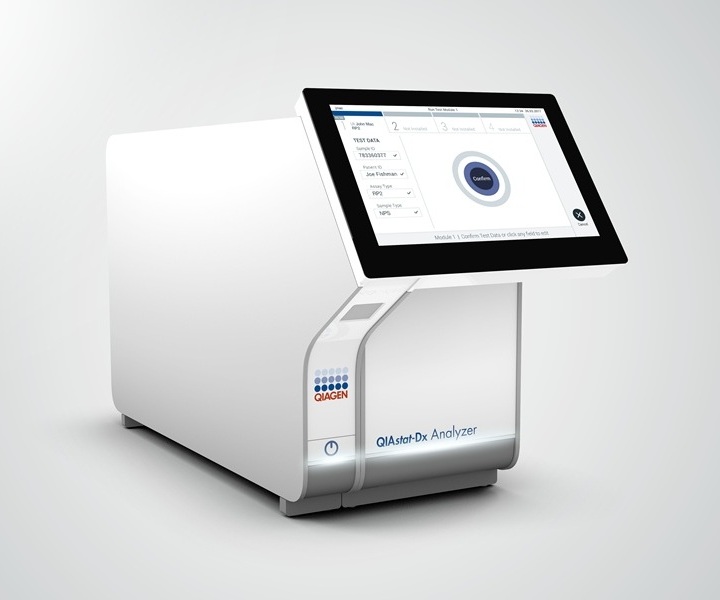
Gastrointestinal Panel Delivers Rapid Detection of Five Common Bacterial Pathogens for Outpatient Use
Acute infectious gastroenteritis results in approximately 179 million cases each year in the United States, leading to a significant number of outpatient visits and hospitalizations. To address this, a... Read moreTechnology
view channel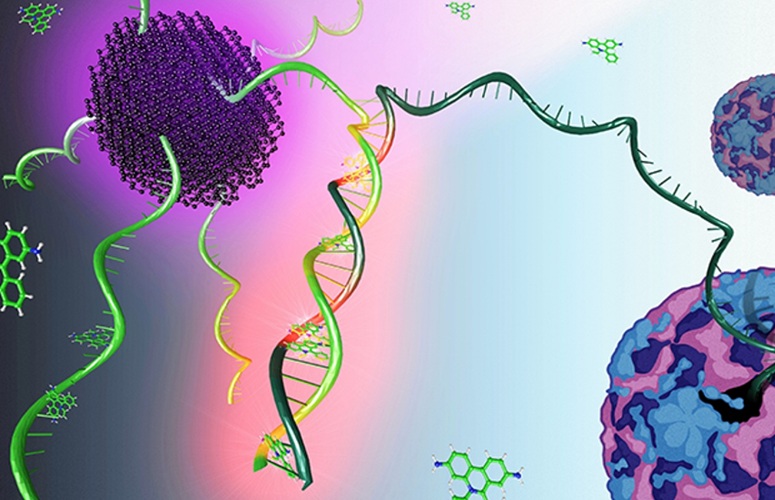
Innovative, Label-Free Ratiometric Fluorosensor Enables More Sensitive Viral RNA Detection
Viruses present a major global health risk, as demonstrated by recent pandemics, making early detection and identification essential for preventing new outbreaks. While traditional detection methods are... Read more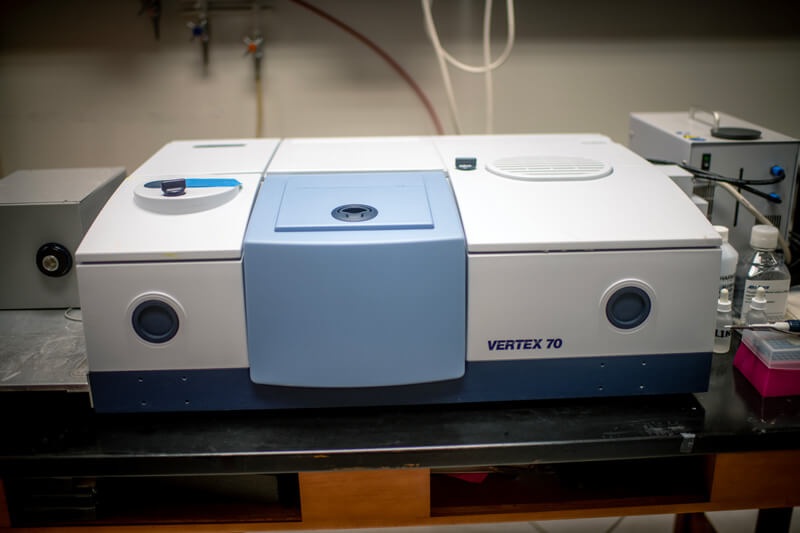
Smartphones Could Diagnose Diseases Using Infrared Scans
Rapid advancements in technology may soon make it possible for individuals to bypass invasive medical procedures by simply uploading a screenshot of their lab results from their phone directly to their doctor.... Read more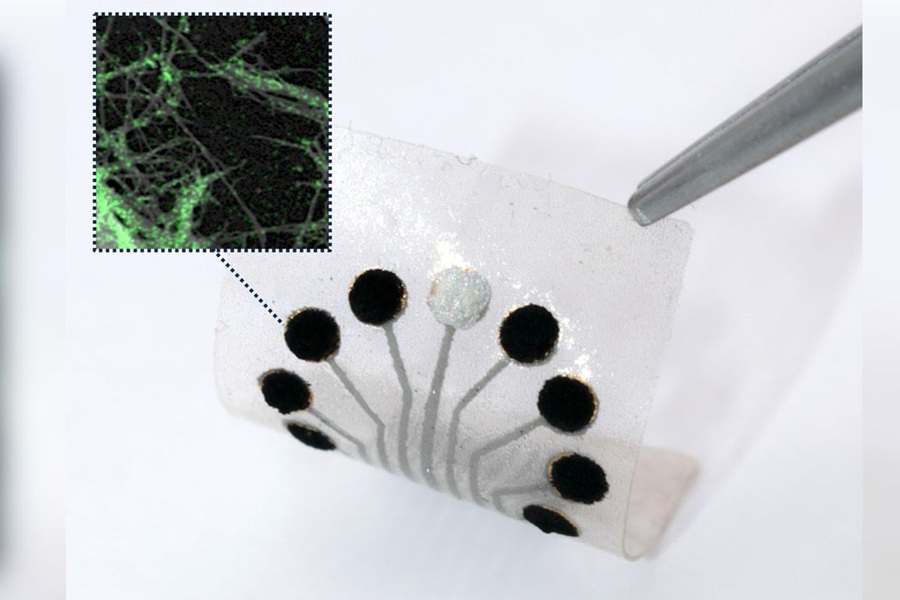
Novel Sensor Technology to Enable Early Diagnoses of Metabolic and Cardiovascular Disorders
Metabolites are critical compounds that fuel life's essential functions, playing a key role in producing energy, regulating cellular activities, and maintaining the balance of bodily systems.... Read more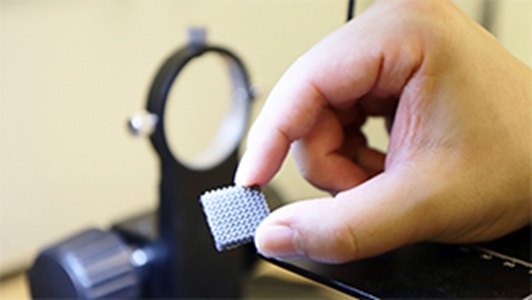
3D Printing Breakthrough Enables Large Scale Development of Tiny Microfluidic Devices
Microfluidic devices are diagnostic systems capable of analyzing small volumes of materials with precision and speed. These devices are used in a variety of applications, including cancer cell analysis,... Read moreIndustry
view channel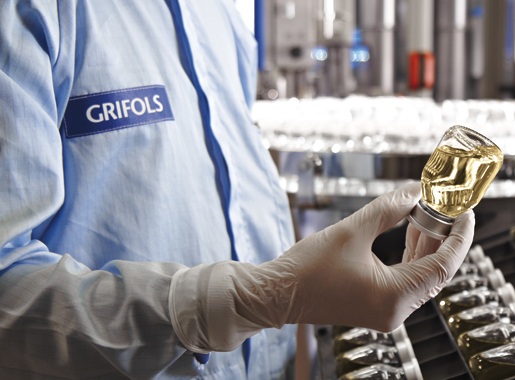
Grifols and Tecan’s IBL Collaborate on Advanced Biomarker Panels
Grifols (Barcelona, Spain), one of the world’s leading producers of plasma-derived medicines and innovative diagnostic solutions, is expanding its offer in clinical diagnostics through a strategic partnership... Read more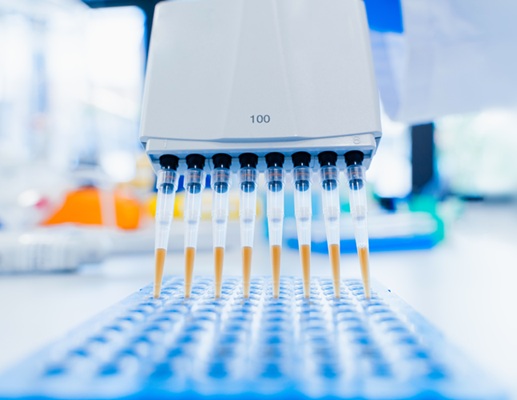