Powerful AI Tool Diagnoses Coeliac Disease from Biopsy Images with Over 97% Accuracy
Posted on 28 Mar 2025
Coeliac disease is an autoimmune disorder triggered by the consumption of gluten, causing symptoms such as stomach cramps, diarrhea, skin rashes, weight loss, fatigue, and anemia. Due to the wide variation in symptoms between individuals, patients often struggle to obtain an accurate diagnosis. The standard method for diagnosing coeliac disease involves performing a biopsy of the duodenum (the first part of the small intestine). Pathologists then examine the sample under a microscope or on a computer to identify damage to the villi, which are tiny hair-like structures lining the small intestine. Interpreting these biopsies can be challenging, as the changes often appear subtle. Pathologists typically use the Marsh-Oberhuber scale to assess the severity of the condition, ranging from zero (normal villi, indicating a low likelihood of coeliac disease) to four (completely flattened villi, indicating severe disease). New research now shows that a machine learning algorithm was able to accurately determine, in 97 out of 100 cases, whether an individual had coeliac disease based on their biopsy.
This AI tool, developed by scientists at the University of Cambridge (Cambridge, UK), could expedite the diagnosis of coeliac disease, alleviate pressure on strained healthcare systems, and improve diagnoses in developing countries, where there is a significant shortage of pathologists. In research published in The New England Journal of Medicine AI, the Cambridge researchers presented their machine learning algorithm designed to classify biopsy image data. The algorithm was trained on a comprehensive dataset of over 4,000 images obtained from five hospitals, utilizing five different scanners from four different manufacturers. The team also tested their algorithm on an independent dataset of almost 650 images from an unseen source. When compared with the original diagnoses made by pathologists, the model correctly identified the presence or absence of coeliac disease in more than 97 cases out of 100.
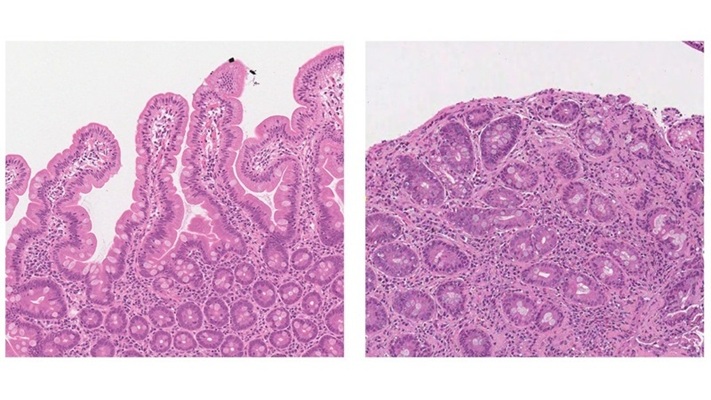
The model demonstrated a sensitivity of over 95%, meaning it accurately identified more than 95 out of 100 individuals with coeliac disease. Additionally, it had a specificity of nearly 98%, meaning it correctly identified almost 98 out of 100 individuals without the disease. Previous research by the team has shown that even pathologists can have differing opinions. In one study, when asked to diagnose coeliac disease on a series of 100 slides, more than one in five cases led to disagreements among pathologists. In this new study, the researchers asked four pathologists to review 30 slides and found that a pathologist was just as likely to agree with the AI model as they were with another pathologist.
“This is the first time AI has been shown to diagnose as accurately as an experienced pathologist whether an individual has coeliac or not. Because we trained it on data sets generated under a number of different conditions, we know that it should be able to work in a wide range of settings, where biopsies are processed and imaged differently,” said Dr. Florian Jaeckle, from the Department of Pathology, and a Research Fellow at Hughes Hall, Cambridge. “This is an important step towards speeding up diagnoses and freeing up pathologists’ time to focus on more complex or urgent cases. Our next step is to test the algorithm in a much larger clinical sample, putting us in a position to share this device with the regulator, bringing us nearer to this tool being used in the NHS.”