AI-Powered Deep Learning Model Accurately Counts Cell Types in Whole Slide Images
By LabMedica International staff writers Posted on 19 Apr 2023 |
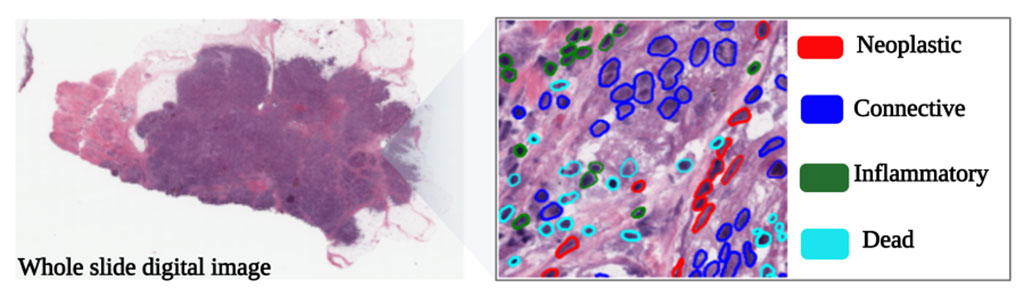
Improved methods for counting cell types in pathology images using deep learning approaches are much needed. Current techniques based on segmentation and regression face challenges such as the necessity for precise pixel-level annotations, difficulties in handling overlapping nuclei or obscured regions, and insufficient information on individual cell type locations. Moreover, probabilistic models tend to yield uncertain predictions and can lead to overconfident predictions. Researchers have now developed an advanced deep learning model to predict and count various cell types in the tumor microenvironment, which is expected to enhance the accuracy and efficiency of cancer diagnostics and treatment planning.
Identifying the different cell types in the tumor microenvironment can offer valuable insights into the tumor's histology and underlying biology. Precise and reliable cell type counting is also crucial for research and clinical applications. In addition, cell counts can be used to study the distribution of different cell types in the tumor microenvironment and its correlation with patient outcomes. In clinical settings, cell counts can help monitor therapy response and track disease progression. Researchers from the University of Eastern Finland (Kuopio, Finland) have proposed a new evidential multi-task deep learning approach, called CT-EMT, to overcome the limitations of current methods for cell type counting in whole slide tumor images. This approach formulates cell type density estimation and cell type counting as regression tasks, and nuclei segmentation as a pixel-level classification task.
The proposed cell type segmentation and counting approach has outperformed state-of-the-art HoVer-Net and StarDist models, with relative improvements of 21% and 12% in terms of mean panoptic quality. The developed model can deliver persuasive interpretations of diverse cell types and can be applied to various computational pathology tasks, such as tumor grading, prognosis, and treatment planning. This work will pave the way for the creation of more accurate and robust digital pathology tools that can support pathologists and clinicians in diagnosing and treating cancer patients.
“The UEF Cancer AI research team aims to explore the potential of using deep learning technology in cancer and health data analysis,” said senior researcher Hamid Behravan of the University of Eastern Finland. “Our study will involve the development and evaluation of cutting-edge deep learning algorithms for analyzing cancer and various types of health-related data, including medical images, genomic data, and electronic health records. We believe that this approach has the potential to significantly improve the accuracy and efficiency of breast cancer diagnosis and treatment planning, as well as to facilitate the discovery of new insights and patterns in cancer data. We hope that our research will contribute to the advancement of precision medicine and the development of more effective and personalized approaches to breast cancer prevention and prognosis.”
Related Links:
University of Eastern Finland
Latest Pathology News
- Spit Test More Accurate at Identifying Future Prostate Cancer Risk
- DNA Nanotechnology Boosts Sensitivity of Test Strips
- Novel UV and Machine Learning-Aided Method Detects Microbial Contamination in Cell Cultures
- New Error-Corrected Method to Help Detect Cancer from Blood Samples Alone
- "Metal Detector" Algorithm Hunts Down Vulnerable Tumors
- Novel Technique Uses ‘Sugar’ Signatures to Identify and Classify Pancreatic Cancer Cell Subtypes
- Advanced Imaging Reveals Mechanisms Causing Autoimmune Disease
- AI Model Effectively Predicts Patient Outcomes in Common Lung Cancer Type
- AI Model Predicts Patient Response to Bladder Cancer Treatment
- New Laser-Based Method to Accelerate Cancer Diagnosis
- New AI Model Predicts Gene Variants’ Effects on Specific Diseases
- Powerful AI Tool Diagnoses Coeliac Disease from Biopsy Images with Over 97% Accuracy
- Pre-Analytical Conditions Influence Cell-Free MicroRNA Stability in Blood Plasma Samples
- 3D Cell Culture System Could Revolutionize Cancer Diagnostics
- Painless Technique Measures Glucose Concentrations in Solution and Tissue Via Sound Waves
- Skin-Based Test to Improve Diagnosis of Rare, Debilitating Neurodegenerative Disease
Channels
Clinical Chemistry
view channel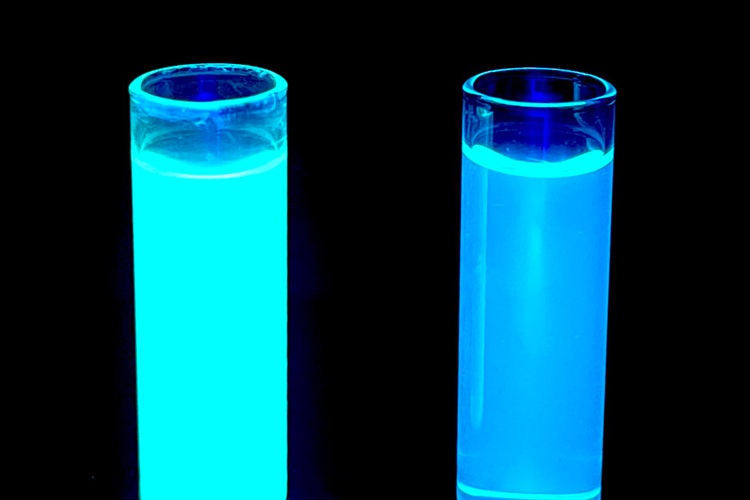
‘Brilliantly Luminous’ Nanoscale Chemical Tool to Improve Disease Detection
Thousands of commercially available glowing molecules known as fluorophores are commonly used in medical imaging, disease detection, biomarker tagging, and chemical analysis. They are also integral in... Read more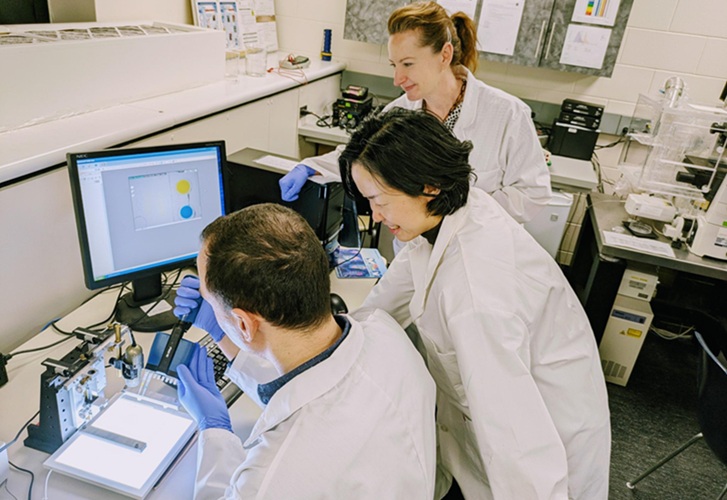
Low-Cost Portable Screening Test to Transform Kidney Disease Detection
Millions of individuals suffer from kidney disease, which often remains undiagnosed until it has reached a critical stage. This silent epidemic not only diminishes the quality of life for those affected... Read more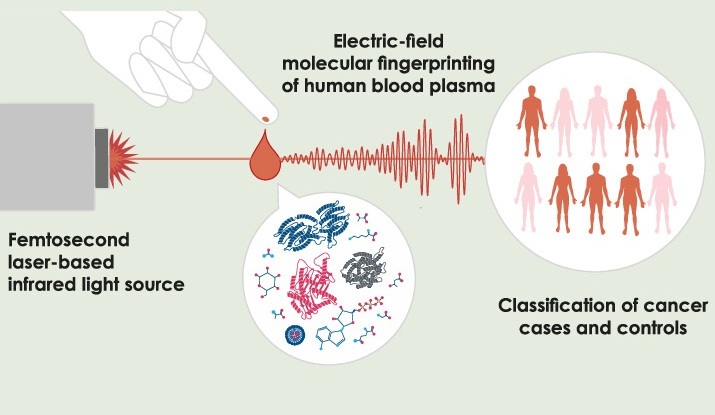
New Method Uses Pulsed Infrared Light to Find Cancer's 'Fingerprints' In Blood Plasma
Cancer diagnoses have traditionally relied on invasive or time-consuming procedures like tissue biopsies. Now, new research published in ACS Central Science introduces a method that utilizes pulsed infrared... Read moreMolecular Diagnostics
view channel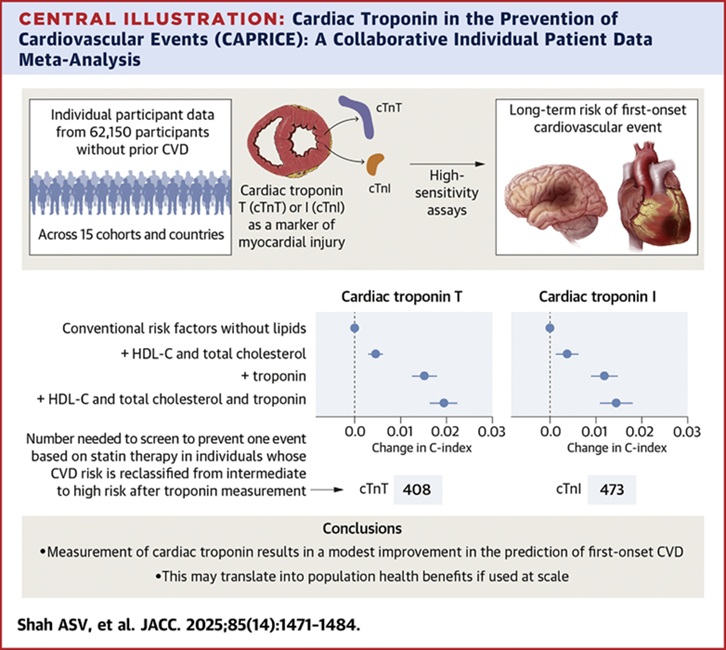
Simple Blood Test Improves Heart Attack and Stroke Risk Prediction
Troponin is a protein found in heart muscle cells that is released into the bloodstream when the heart is damaged. High-sensitivity troponin blood tests are commonly used in hospitals to diagnose heart... Read more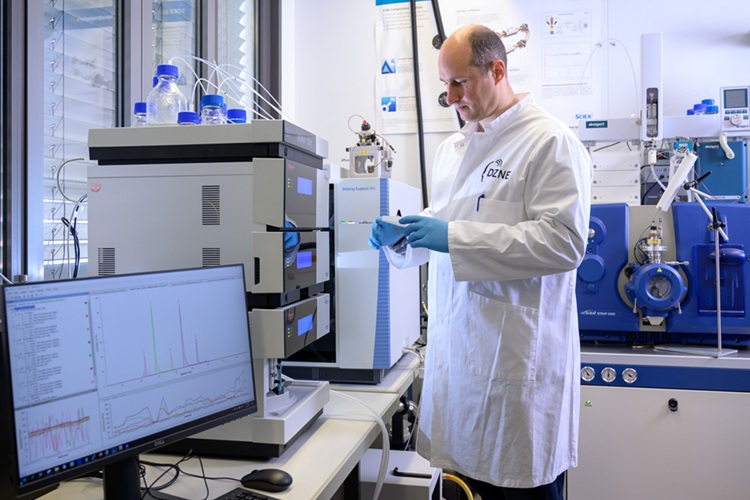
Blood Biomarker Test Could Detect Genetic Predisposition to Alzheimer’s
New medications for Alzheimer’s disease, the most common form of dementia, are now becoming available. These treatments, known as “amyloid antibodies,” work by promoting the removal of small deposits from... Read more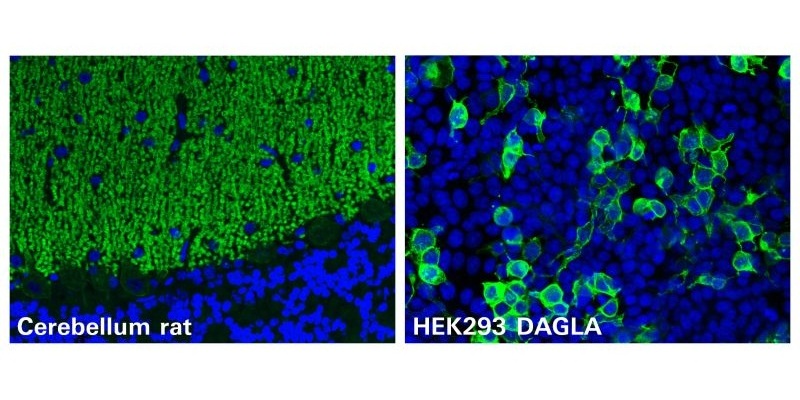
Novel Autoantibody Against DAGLA Discovered in Cerebellitis
Autoimmune cerebellar ataxias are strongly disabling disorders characterized by an impaired ability to coordinate muscle movement. Cerebellar autoantibodies serve as useful biomarkers to support rapid... Read more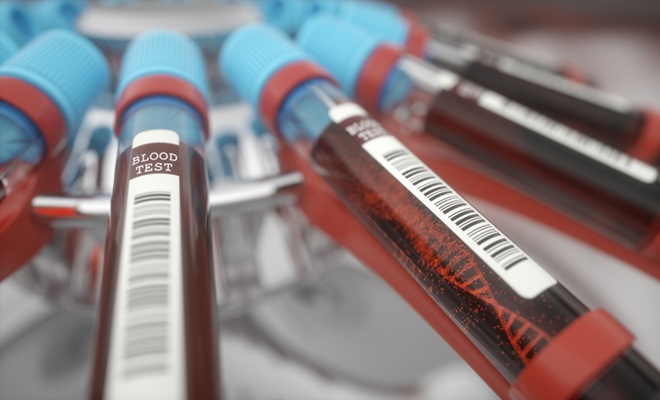
Gene-Based Blood Test Accurately Predicts Tumor Recurrence of Advanced Skin Cancer
Melanoma, an aggressive form of skin cancer, becomes extremely difficult to treat once it spreads to other parts of the body. For patients with metastatic melanoma tumors that cannot be surgically removed... Read moreHematology
view channel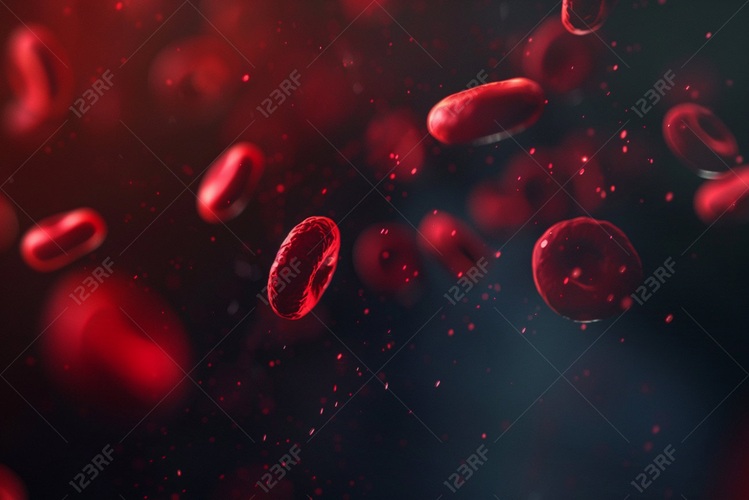
New Scoring System Predicts Risk of Developing Cancer from Common Blood Disorder
Clonal cytopenia of undetermined significance (CCUS) is a blood disorder commonly found in older adults, characterized by mutations in blood cells and a low blood count, but without any obvious cause or... Read more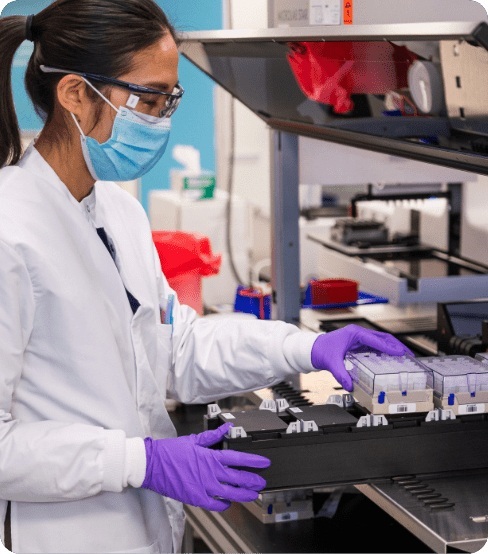
Non-Invasive Prenatal Test for Fetal RhD Status Demonstrates 100% Accuracy
In the United States, approximately 15% of pregnant individuals are RhD-negative. However, in about 40% of these cases, the fetus is also RhD-negative, making the administration of RhoGAM unnecessary.... Read moreImmunology
view channel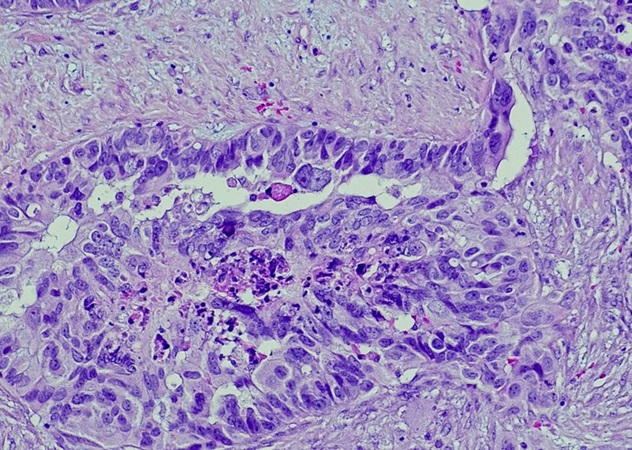
Stem Cell Test Predicts Treatment Outcome for Patients with Platinum-Resistant Ovarian Cancer
Epithelial ovarian cancer frequently responds to chemotherapy initially, but eventually, the tumor develops resistance to the therapy, leading to regrowth. This resistance is partially due to the activation... Read more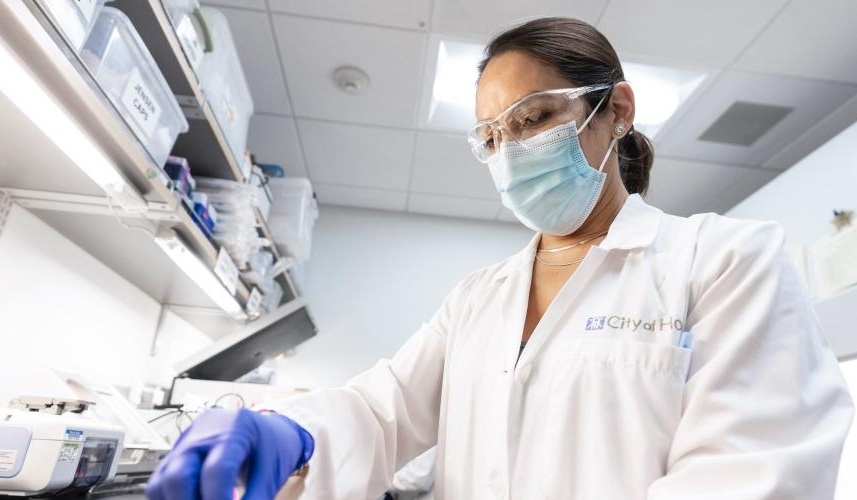
Machine Learning-Enabled Blood Test Predicts Immunotherapy Response in Lymphoma Patients
Chimeric antigen receptor (CAR) T-cell therapy has emerged as one of the most promising recent developments in the treatment of blood cancers. However, over half of non-Hodgkin lymphoma (NHL) patients... Read moreMicrobiology
view channel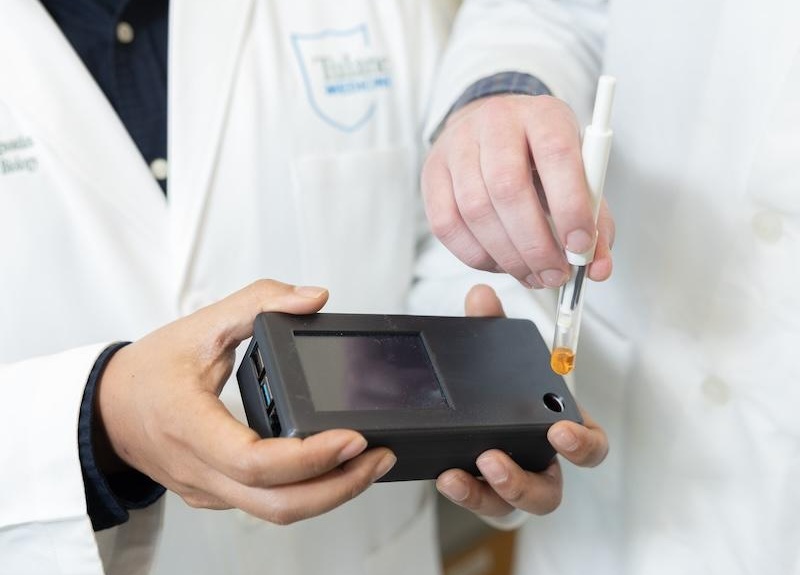
Handheld Device Delivers Low-Cost TB Results in Less Than One Hour
Tuberculosis (TB) remains the deadliest infectious disease globally, affecting an estimated 10 million people annually. In 2021, about 4.2 million TB cases went undiagnosed or unreported, mainly due to... Read more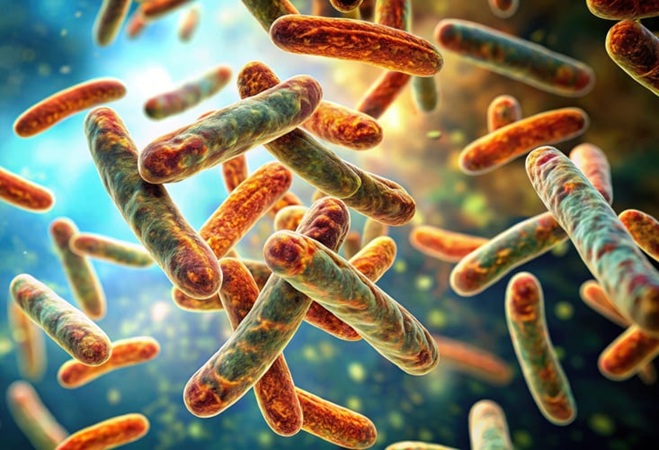
New AI-Based Method Improves Diagnosis of Drug-Resistant Infections
Drug-resistant infections, particularly those caused by deadly bacteria like tuberculosis and staphylococcus, are rapidly emerging as a global health emergency. These infections are more difficult to treat,... Read more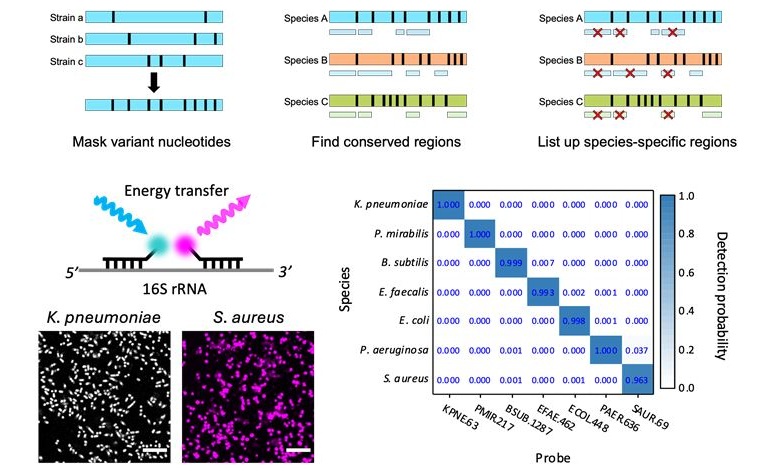
Breakthrough Diagnostic Technology Identifies Bacterial Infections with Almost 100% Accuracy within Three Hours
Rapid and precise identification of pathogenic microbes in patient samples is essential for the effective treatment of acute infectious diseases, such as sepsis. The fluorescence in situ hybridization... Read moreTechnology
view channel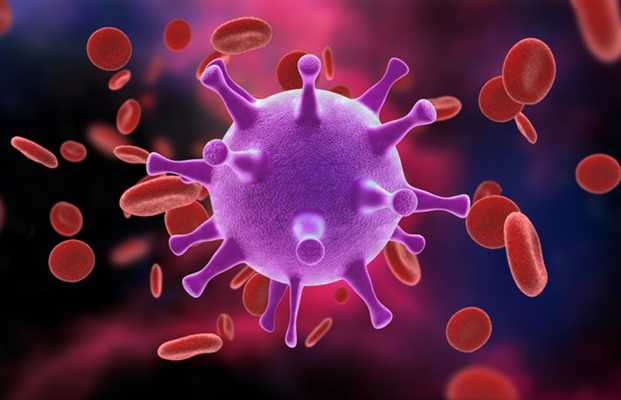
Disposable Microchip Technology Could Selectively Detect HIV in Whole Blood Samples
As of the end of 2023, approximately 40 million people globally were living with HIV, and around 630,000 individuals died from AIDS-related illnesses that same year. Despite a substantial decline in deaths... Read more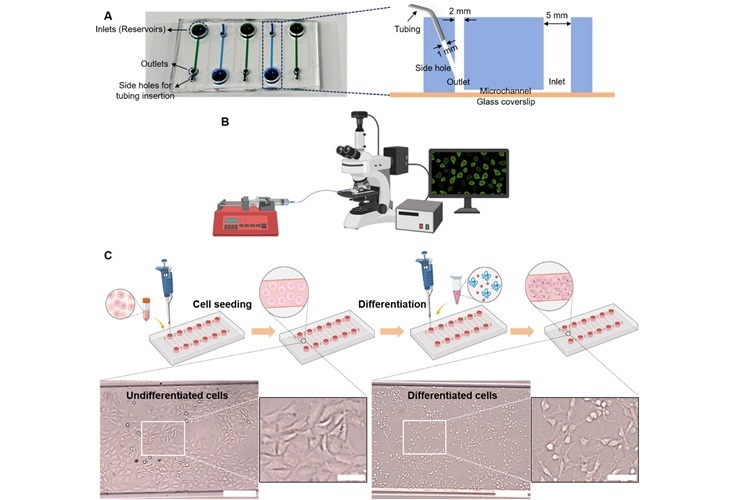
Pain-On-A-Chip Microfluidic Device Determines Types of Chronic Pain from Blood Samples
Chronic pain is a widespread condition that remains difficult to manage, and existing clinical methods for its treatment rely largely on self-reporting, which can be subjective and especially problematic... Read more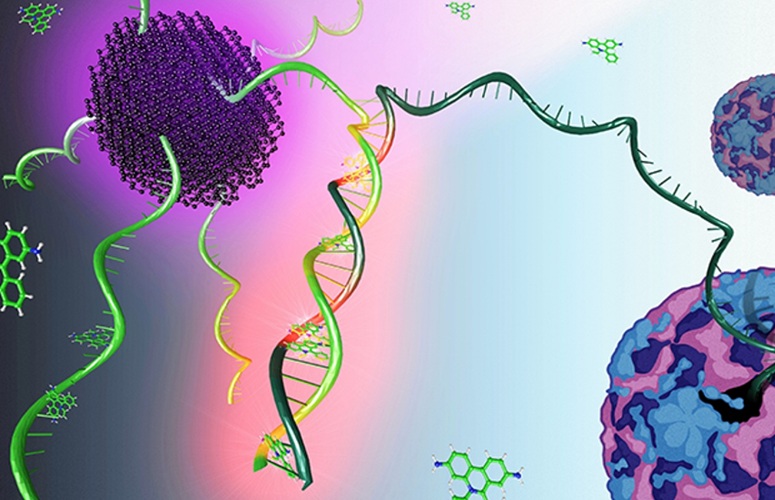
Innovative, Label-Free Ratiometric Fluorosensor Enables More Sensitive Viral RNA Detection
Viruses present a major global health risk, as demonstrated by recent pandemics, making early detection and identification essential for preventing new outbreaks. While traditional detection methods are... Read moreIndustry
view channel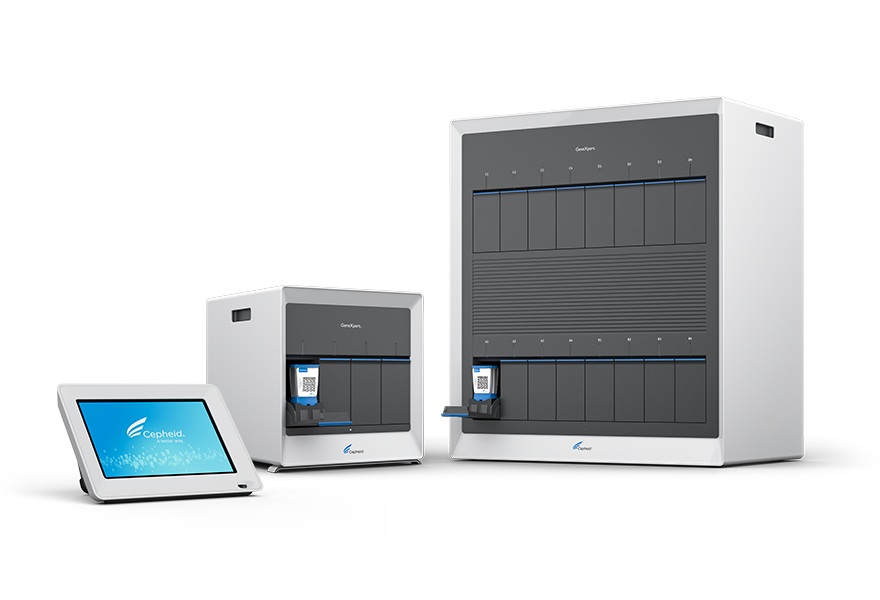
Cepheid and Oxford Nanopore Technologies Partner on Advancing Automated Sequencing-Based Solutions
Cepheid (Sunnyvale, CA, USA), a leading molecular diagnostics company, and Oxford Nanopore Technologies (Oxford, UK), the company behind a new generation of sequencing-based molecular analysis technologies,... Read more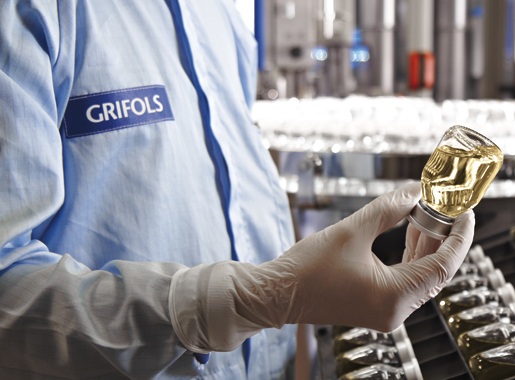
Grifols and Tecan’s IBL Collaborate on Advanced Biomarker Panels
Grifols (Barcelona, Spain), one of the world’s leading producers of plasma-derived medicines and innovative diagnostic solutions, is expanding its offer in clinical diagnostics through a strategic partnership... Read more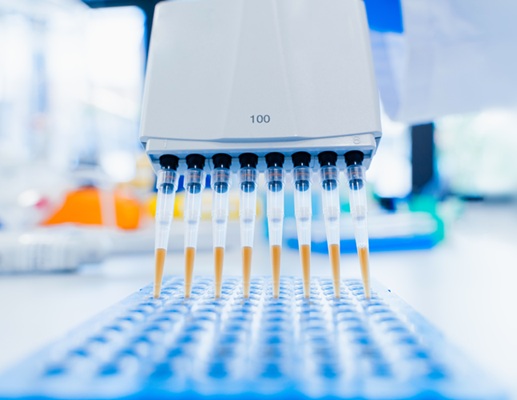