Machine Learning Radically Reduces Workload of Cell Counting for Disease Diagnosis
By LabMedica International staff writers Posted on 24 May 2022 |
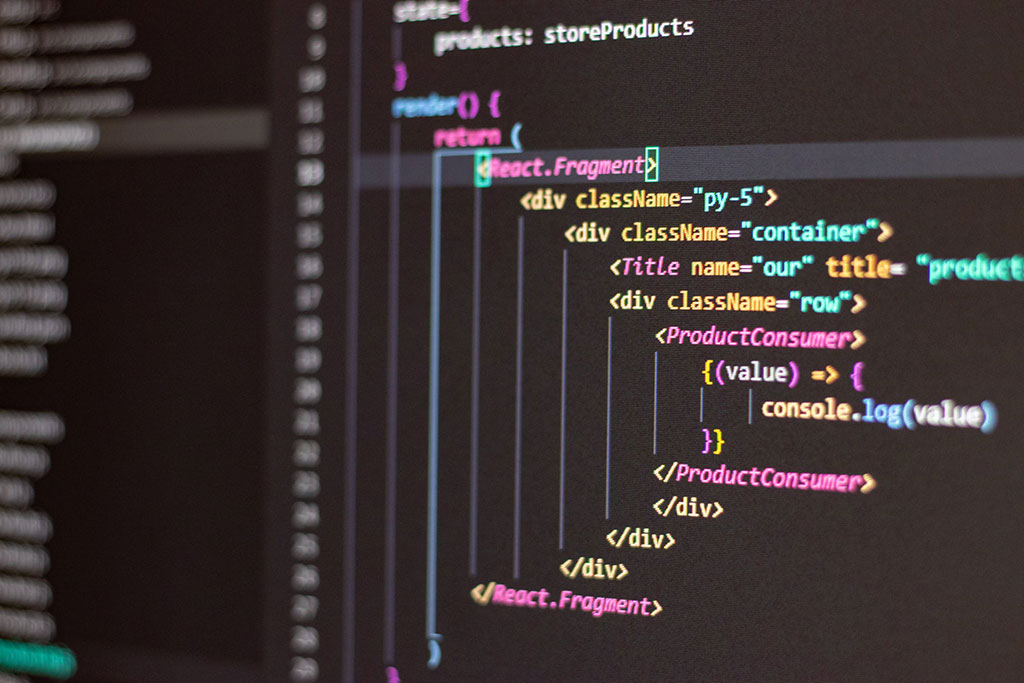
Machine learning methods are increasingly spreading into the area of blood cell counting, until recently dominated by expensive and often less accurate traditional cell analyzers. However, due to the enormous amount of manual annotation work required, training the machine learning model has so far proven highly labor-intensive. Now, researchers have developed an innovative training method that automates much of this activity.
Researchers at Benihang University (Beijing, China) have developed the new scheme for training a convolutional neural network (CNN) - a type of machine learning that mirrors the connection structure of the human visual cortex. The number and type of cells in the blood often play a crucial role in disease diagnosis, but the cell analysis techniques commonly used to perform such counting of blood cells - involving the detection and measurement of physical and chemical characteristics of cells suspended in fluid - are expensive and require complex preparations. Worse still, the accuracy of cell analyzer machines is only about 90% due to various influences such as temperature, pH, voltage, and magnetic field that can confuse the equipment.
In order to improve accuracy, reduce complexity and lower costs, much research into alternatives has lately focused on the use of computer programs to perform “segmentation” on photographs of the blood taken by a high-definition camera connected to a microscope. Segmentation involves algorithms that perform pixel-by-pixel labeling of what appears in a photo, in this case, what parts of the image are cells and which are not - in essence, counting the number of cells in an image. For images in which only a single type of cell appears, such methods achieve a decent level of accuracy, but they perform poorly when confronting images with multiple types of cells. So in recent years, in attempts to solve the problem, researchers have turned to CNNs.
For the CNN to perform this task, it must first be “trained” to understand what is and is not a cell on many thousands of images of cells that humans have manually labeled. Then, when fed a novel, unlabelled image, it recognizes and can count the cells in it. The researchers at Beihang University developed a new scheme for training the CNN, in this case, U-Net, a fully convolutional network segmentation model that has been widely used in medical image segmentation since it was first developed in 2015. In the new training scheme, the CNN is first trained on a set of many thousands of images with only one type of cell (taken from the blood of mice).
These single-cell-type images are “preprocessed” automatically by conventional algorithms that reduce noise in the images, enhance their quality, and detect the contours of objects in the image. They then perform adaptive image segmentation. This latter algorithm calculates the various levels of gray in a black and white image, and if a part of the image lies beyond a certain threshold of gray, the algorithm segments that out as a distinct object. What makes the process adaptive is that rather than segmenting out parts of the image segments according to a fixed gray threshold, it does this according to the local features of the image.
After the single-cell-type training set is presented to the U-Net model, the model is fine-tuned using a small set of manually annotated images of multiple cell types. In comparison, a certain amount of manual annotation remains, and the number of images needed to be labeled by humans drops from what was previously many thousands to just 600. To test their training scheme, the researchers first used a traditional cell analyzer on the same mouse blood samples to do an independent cell count against which they could compare their new approach. They found that the accuracy of their training scheme on segmentation of multiple-cell-type images was 94.85%, which is the same level achieved by training with manually annotated multiple-cell-type images. The technique can also be applied to more advanced models to consider more complex segmentation problems. As the new training technique still involves some level of manual annotation, the researchers hope to go on to develop a fully automatic algorithm for annotating and training models.
Related Links:
Benihang University
Latest Hematology News
- New Scoring System Predicts Risk of Developing Cancer from Common Blood Disorder
- Non-Invasive Prenatal Test for Fetal RhD Status Demonstrates 100% Accuracy
- WBC Count Could Predict Severity of COVID-19 Symptoms
- New Platelet Counting Technology to Help Labs Prevent Diagnosis Errors
- Streamlined Approach to Testing for Heparin-Induced Thrombocytopenia Improves Diagnostic Accuracy
- POC Hemostasis System Could Help Prevent Maternal Deaths
- New Test Assesses Oxygen Delivering Ability of Red Blood Cells by Measuring Their Shape
- Personalized CBC Testing Could Help Diagnose Early-Stage Diseases in Healthy Individuals
- Non-Invasive Test Solution Determines Fetal RhD Status from Maternal Plasma
- First-Of-Its-Kind Smartphone Technology Noninvasively Measures Blood Hemoglobin Levels at POC
- Next Gen CBC and Sepsis Diagnostic System Targets Faster, Earlier, Easier Results
- Newly Discovered Blood Group System to Help Identify and Treat Rare Patients
- Blood Platelet Score Detects Previously Unmeasured Risk of Heart Attack and Stroke
- Automated Benchtop System to Bring Blood Testing To Anyone, Anywhere
- New Hematology Analyzers Deliver Combined ESR and CBC/DIFF Results in 60 Seconds
- Next Generation Instrument Screens for Hemoglobin Disorders in Newborns
Channels
Clinical Chemistry
view channel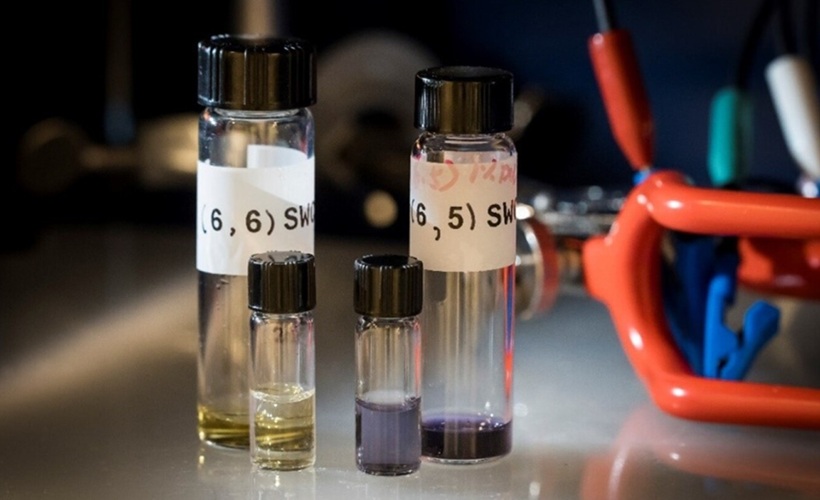
Carbon Nanotubes Help Build Highly Accurate Sensors for Continuous Health Monitoring
Current sensors can measure various health indicators, such as blood glucose levels, in the body. However, there is a need to develop more accurate and sensitive sensor materials that can detect lower... Read more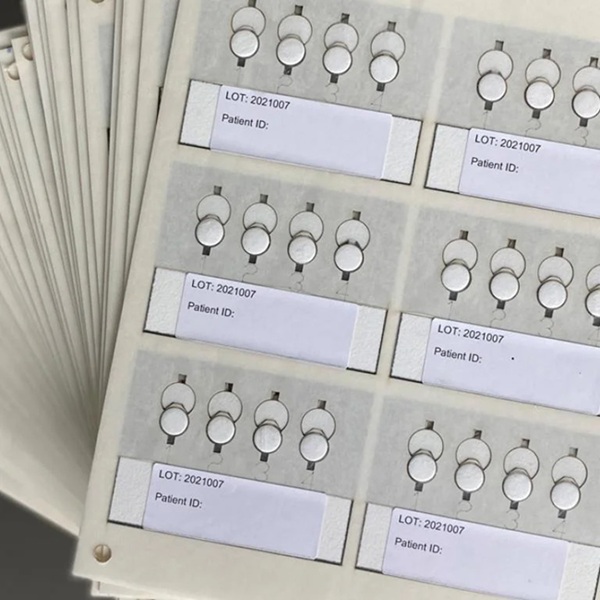
Paper-Based Device Boosts HIV Test Accuracy from Dried Blood Samples
In regions where access to clinics for routine blood tests presents financial and logistical obstacles, HIV patients are increasingly able to collect and send a drop of blood using paper-based devices... Read moreMolecular Diagnostics
view channel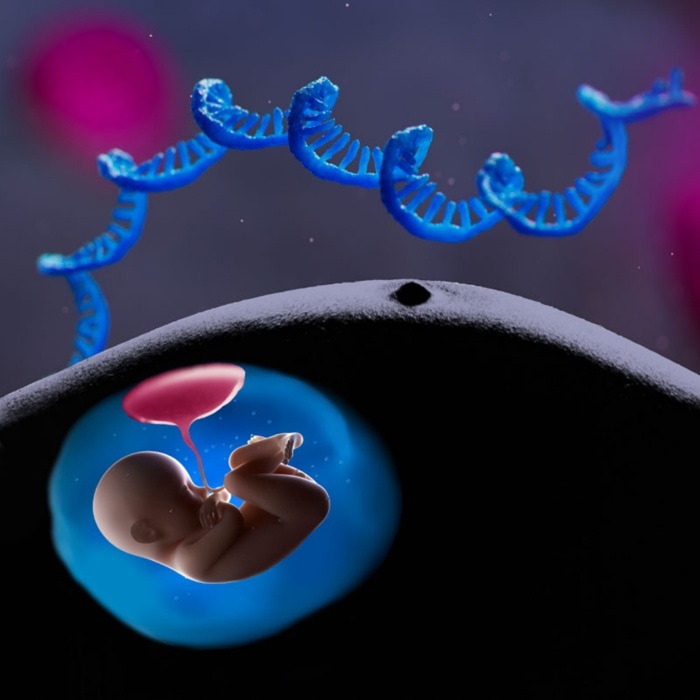
RNA-Based Blood Test Detects Preeclampsia Risk Months Before Symptoms
Preeclampsia remains a major cause of maternal morbidity and mortality, as well as preterm births. Despite current guidelines that aim to identify pregnant women at increased risk of preeclampsia using... Read more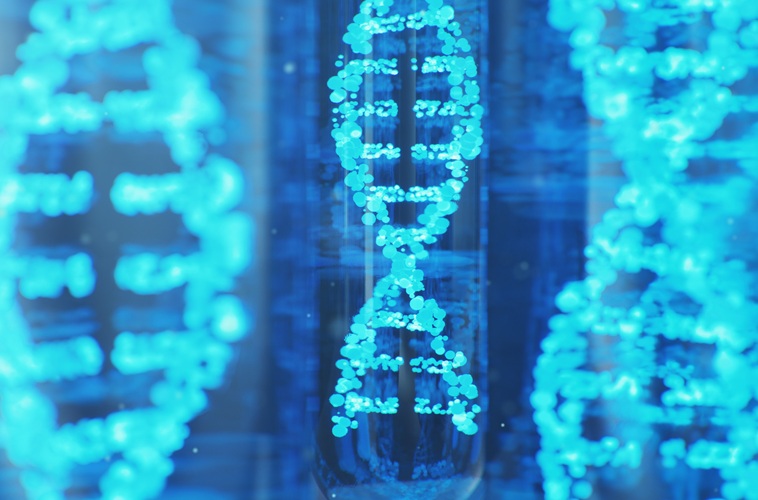
First Of Its Kind Test Uses microRNAs to Predict Toxicity from Cancer Therapy
Many men with early-stage prostate cancer receive stereotactic body radiotherapy (SBRT), a highly precise form of radiation treatment that is completed in just five sessions. Compared to traditional radiation,... Read more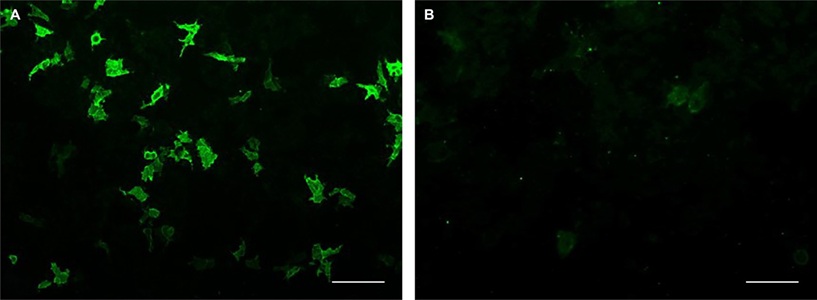
Novel Cell-Based Assay Provides Sensitive and Specific Autoantibody Detection in Demyelination
Anti-myelin-associated glycoprotein (MAG) antibodies serve as markers for an autoimmune demyelinating disorder that affects the peripheral nervous system, leading to sensory impairment. Anti-MAG-IgM antibodies... Read moreImmunology
view channel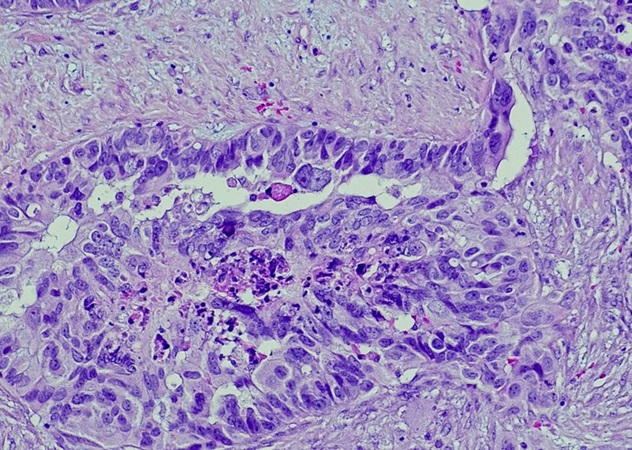
Stem Cell Test Predicts Treatment Outcome for Patients with Platinum-Resistant Ovarian Cancer
Epithelial ovarian cancer frequently responds to chemotherapy initially, but eventually, the tumor develops resistance to the therapy, leading to regrowth. This resistance is partially due to the activation... Read more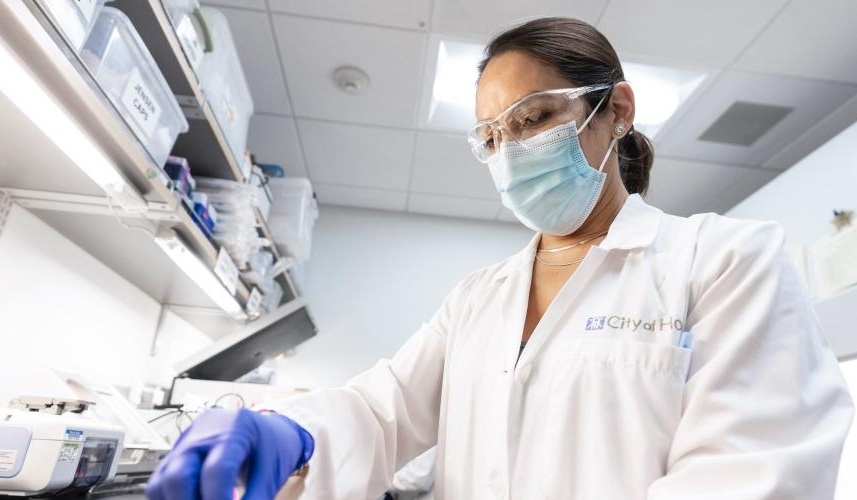
Machine Learning-Enabled Blood Test Predicts Immunotherapy Response in Lymphoma Patients
Chimeric antigen receptor (CAR) T-cell therapy has emerged as one of the most promising recent developments in the treatment of blood cancers. However, over half of non-Hodgkin lymphoma (NHL) patients... Read moreMicrobiology
view channel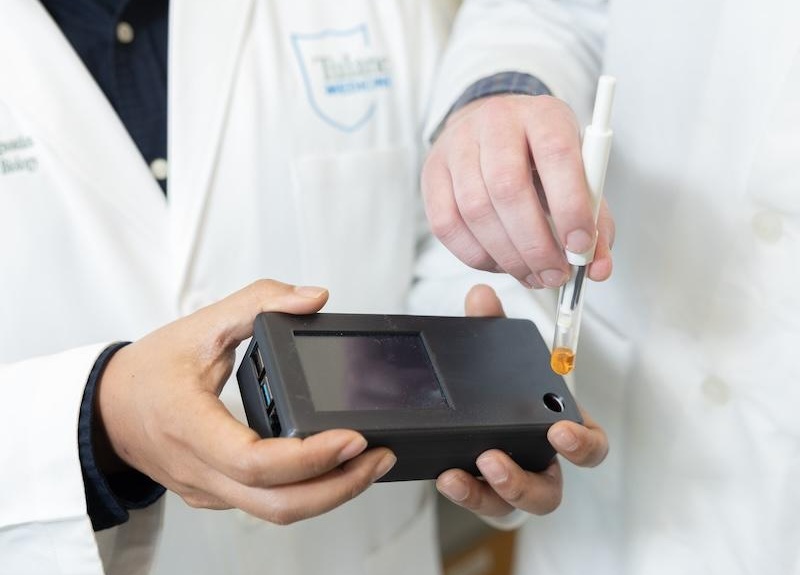
Handheld Device Deliver Low-Cost TB Results in Less Than One Hour
Tuberculosis (TB) remains the deadliest infectious disease globally, affecting an estimated 10 million people annually. In 2021, about 4.2 million TB cases went undiagnosed or unreported, mainly due to... Read more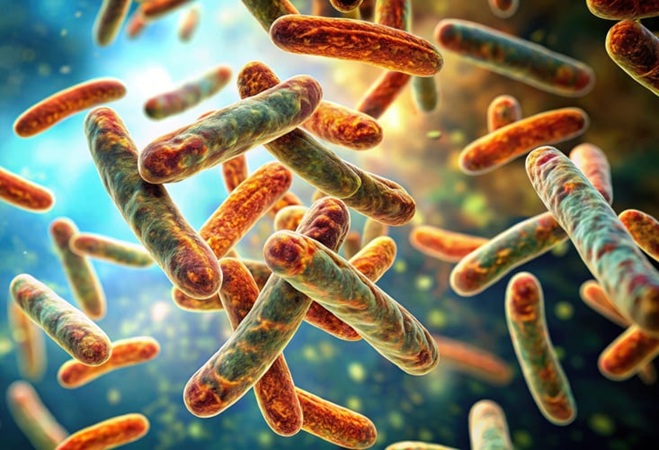
New AI-Based Method Improves Diagnosis of Drug-Resistant Infections
Drug-resistant infections, particularly those caused by deadly bacteria like tuberculosis and staphylococcus, are rapidly emerging as a global health emergency. These infections are more difficult to treat,... Read more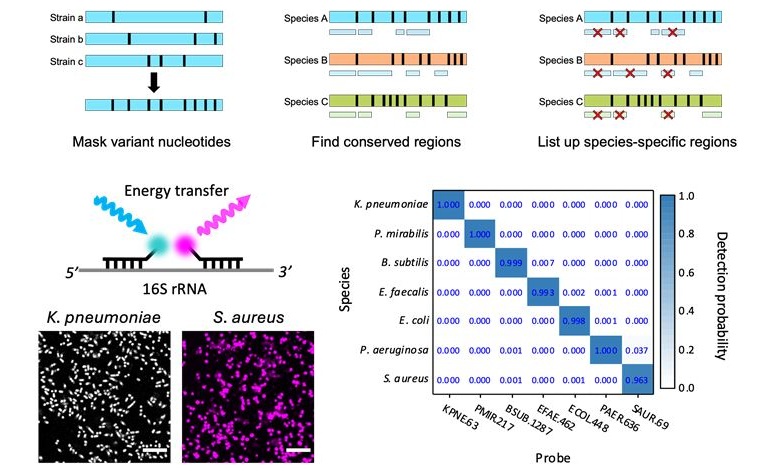
Breakthrough Diagnostic Technology Identifies Bacterial Infections with Almost 100% Accuracy within Three Hours
Rapid and precise identification of pathogenic microbes in patient samples is essential for the effective treatment of acute infectious diseases, such as sepsis. The fluorescence in situ hybridization... Read morePathology
view channel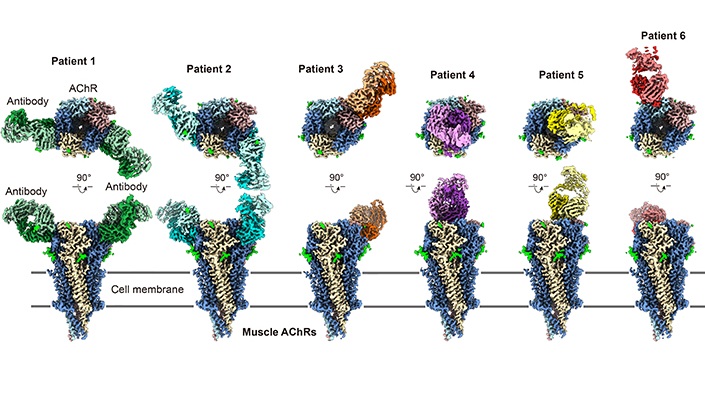
Advanced Imaging Reveals Mechanisms Causing Autoimmune Disease
Myasthenia gravis, an autoimmune disease, leads to muscle weakness that can affect a range of muscles, including those needed for basic actions like blinking, smiling, or moving. Researchers have long... Read more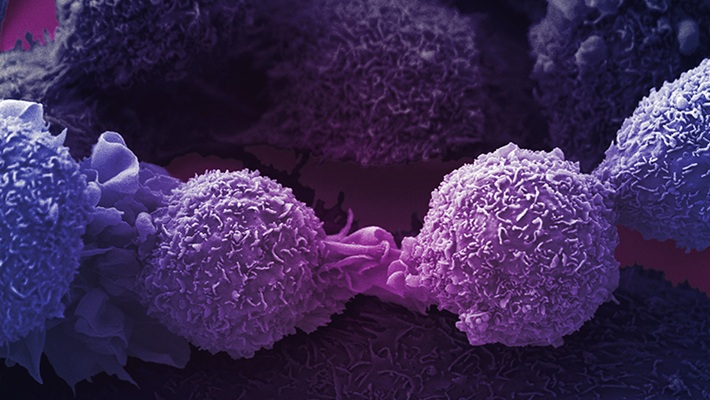
AI Model Effectively Predicts Patient Outcomes in Common Lung Cancer Type
Lung adenocarcinoma, the most common form of non-small cell lung cancer (NSCLC), typically adopts one of six distinct growth patterns, often combining multiple patterns within a single tumor.... Read moreTechnology
view channel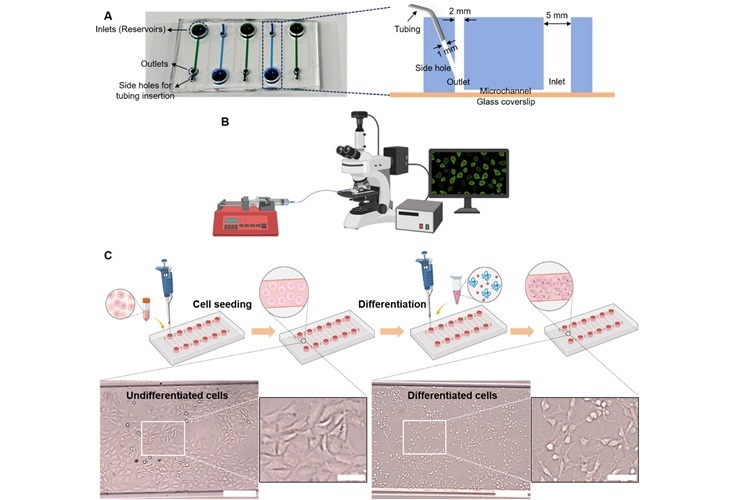
Pain-On-A-Chip Microfluidic Device Determines Types of Chronic Pain from Blood Samples
Chronic pain is a widespread condition that remains difficult to manage, and existing clinical methods for its treatment rely largely on self-reporting, which can be subjective and especially problematic... Read more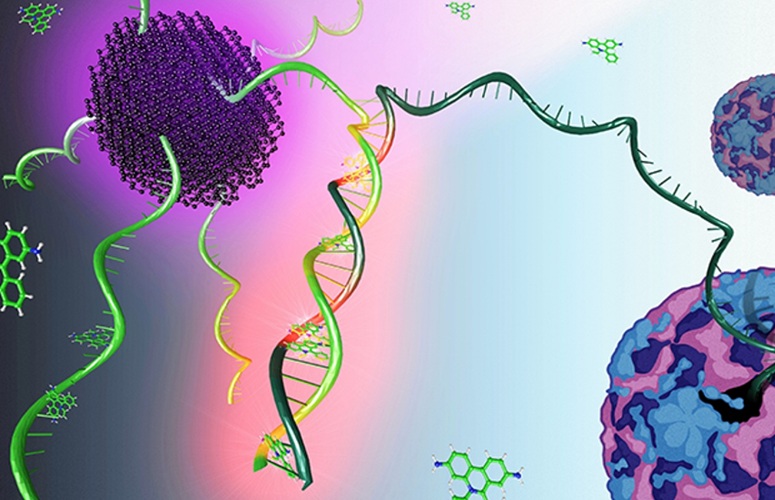
Innovative, Label-Free Ratiometric Fluorosensor Enables More Sensitive Viral RNA Detection
Viruses present a major global health risk, as demonstrated by recent pandemics, making early detection and identification essential for preventing new outbreaks. While traditional detection methods are... Read moreIndustry
view channel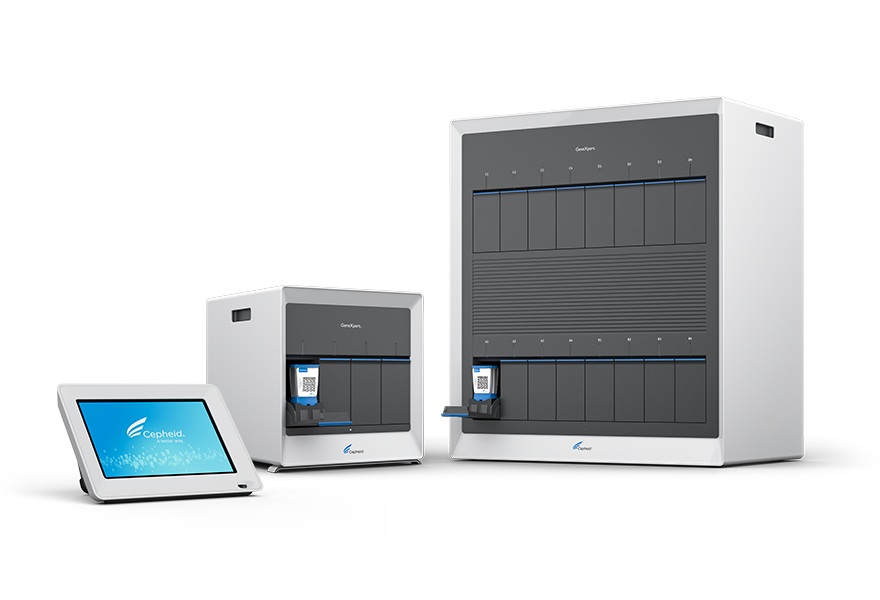
Cepheid and Oxford Nanopore Technologies Partner on Advancing Automated Sequencing-Based Solutions
Cepheid (Sunnyvale, CA, USA), a leading molecular diagnostics company, and Oxford Nanopore Technologies (Oxford, UK), the company behind a new generation of sequencing-based molecular analysis technologies,... Read more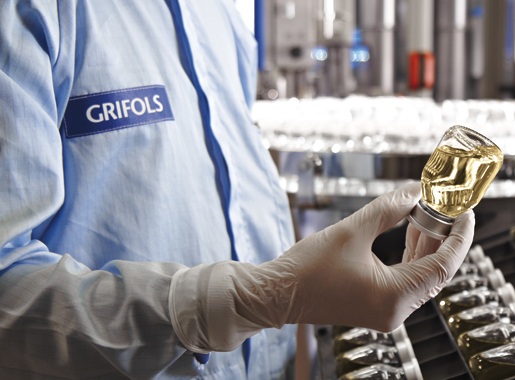
Grifols and Tecan’s IBL Collaborate on Advanced Biomarker Panels
Grifols (Barcelona, Spain), one of the world’s leading producers of plasma-derived medicines and innovative diagnostic solutions, is expanding its offer in clinical diagnostics through a strategic partnership... Read more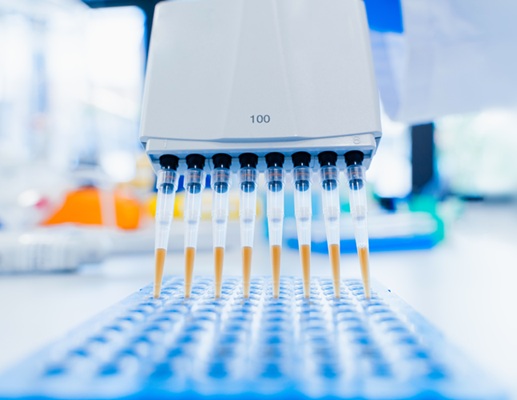