Artificial Intelligence Helps Diagnose Leukemia
By LabMedica International staff writers Posted on 29 Sep 2021 |
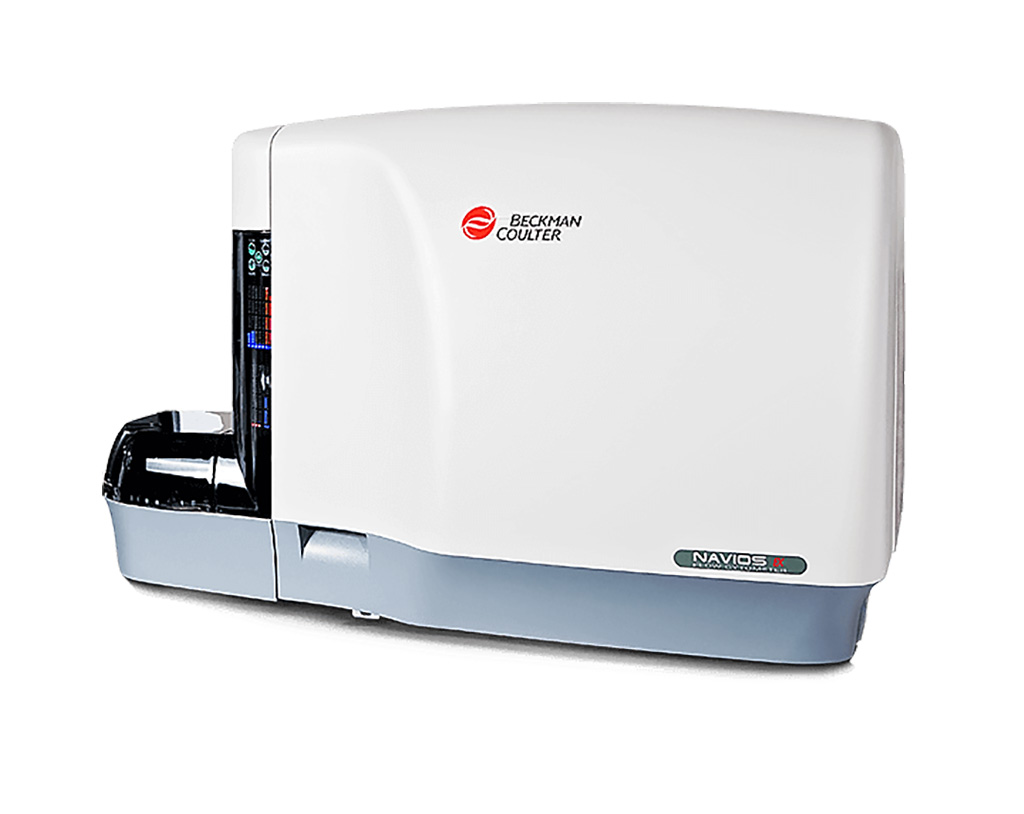
Image: The Navios EX flow cytometer offers a solution for advanced cytometry applications with workflows for high throughput laboratories (Photo courtesy of Beckman Coulter)
Multi-parameter flow cytometry (MFC) is a cornerstone in clinical decision making for leukemia and lymphoma. MFC data analysis requires manual gating of cell populations, which is time-consuming, subjective, and often limited to a two-dimensional space.
Typical symptoms of malignant B-cell lymphomas and related leukemias are that the lymph nodes become swollen, there is weight loss and fatigue, as well as fevers and infections. If such a cancer of the lymphatic system is suspected, the physician takes a blood or bone marrow sample and sends it to specialized laboratories for flow cytometry analysis.
Clinical Scientists and Bioinformaticians associated with University of Bonn (Bonn, Germany) and colleagues from other institutions present a workflow that allows existing artificial intelligence (AI) to adapt to multiple MFC protocols. They combined transfer learning (TL) with MFC data merging to increase the robustness of AI. The base dataset consists of around 18,000 training samples acquired using a 9-color MFC panel at Munich Leukemia Laboratory (MLL, Munich, Germany), between 2017 and 2018. Four additional MFC target datasets were obtained with different MFC panel compositions. All samples were analyzed on Navios flow cytometers (Beckman Coulter, Miami, FL, USA).
Flow cytometry is a method in which the blood cells flow past measurement sensors at high speed. The properties of the cells can be detected depending on their shape, structure or coloring. Detection and accurate characterization of pathological cells is important when making a diagnosis. The laboratories use "antibodies" that dock to the surface of the cells and are coupled to fluorescent dyes. Such markers can also be used to detect small differences between cancer cells and healthy blood cells. Flow cytometry generates large amounts of data. On average, more than 50,000 cells are measured per sample. These data are then typically analyzed on screen by plotting the expression of the markers used against each other.
The great new feature of the AI presented in the study lies in the possibility of knowledge transfer. Particularly smaller laboratories that cannot afford their own bioinformatics expertise and may also have too few samples to develop their own AI from scratch can benefit from this study. After a short training phase, during which the AI learns the specifics of the new laboratory, it can then draw on knowledge derived from many thousands of data sets.
Peter M. Krawitz, MD, PhD, a Professor at the Institute for Genomic Statistics and Bioinformatics and a senior author of the study, said, “The gold standard is diagnosis by hematologists, which can also take into account results of additional tests. The point of using AI is not to replace physicians, but to make the best use of the information contained in the data.”
The authors concluded their workflow extended deep learning models to multiple MFC panels and achieve high accuracy for multi-label classification across datasets. They addressed some of the previous challenges for automated flow cytometry classification by allowing models to be trained with smaller training sizes and generalizing models to work with multiple MFC panels. The workflow is a step toward making deep learning models robust so that AI for diagnostic MFC can move from the “proof of concept” stage into routine diagnostics. The study was published on September 17, 2021 in the journal Patterns.
Related Links:
University of Bonn
Munich Leukemia Laboratory
Beckman Coulter
Typical symptoms of malignant B-cell lymphomas and related leukemias are that the lymph nodes become swollen, there is weight loss and fatigue, as well as fevers and infections. If such a cancer of the lymphatic system is suspected, the physician takes a blood or bone marrow sample and sends it to specialized laboratories for flow cytometry analysis.
Clinical Scientists and Bioinformaticians associated with University of Bonn (Bonn, Germany) and colleagues from other institutions present a workflow that allows existing artificial intelligence (AI) to adapt to multiple MFC protocols. They combined transfer learning (TL) with MFC data merging to increase the robustness of AI. The base dataset consists of around 18,000 training samples acquired using a 9-color MFC panel at Munich Leukemia Laboratory (MLL, Munich, Germany), between 2017 and 2018. Four additional MFC target datasets were obtained with different MFC panel compositions. All samples were analyzed on Navios flow cytometers (Beckman Coulter, Miami, FL, USA).
Flow cytometry is a method in which the blood cells flow past measurement sensors at high speed. The properties of the cells can be detected depending on their shape, structure or coloring. Detection and accurate characterization of pathological cells is important when making a diagnosis. The laboratories use "antibodies" that dock to the surface of the cells and are coupled to fluorescent dyes. Such markers can also be used to detect small differences between cancer cells and healthy blood cells. Flow cytometry generates large amounts of data. On average, more than 50,000 cells are measured per sample. These data are then typically analyzed on screen by plotting the expression of the markers used against each other.
The great new feature of the AI presented in the study lies in the possibility of knowledge transfer. Particularly smaller laboratories that cannot afford their own bioinformatics expertise and may also have too few samples to develop their own AI from scratch can benefit from this study. After a short training phase, during which the AI learns the specifics of the new laboratory, it can then draw on knowledge derived from many thousands of data sets.
Peter M. Krawitz, MD, PhD, a Professor at the Institute for Genomic Statistics and Bioinformatics and a senior author of the study, said, “The gold standard is diagnosis by hematologists, which can also take into account results of additional tests. The point of using AI is not to replace physicians, but to make the best use of the information contained in the data.”
The authors concluded their workflow extended deep learning models to multiple MFC panels and achieve high accuracy for multi-label classification across datasets. They addressed some of the previous challenges for automated flow cytometry classification by allowing models to be trained with smaller training sizes and generalizing models to work with multiple MFC panels. The workflow is a step toward making deep learning models robust so that AI for diagnostic MFC can move from the “proof of concept” stage into routine diagnostics. The study was published on September 17, 2021 in the journal Patterns.
Related Links:
University of Bonn
Munich Leukemia Laboratory
Beckman Coulter
Latest Hematology News
- New Scoring System Predicts Risk of Developing Cancer from Common Blood Disorder
- Non-Invasive Prenatal Test for Fetal RhD Status Demonstrates 100% Accuracy
- WBC Count Could Predict Severity of COVID-19 Symptoms
- New Platelet Counting Technology to Help Labs Prevent Diagnosis Errors
- Streamlined Approach to Testing for Heparin-Induced Thrombocytopenia Improves Diagnostic Accuracy
- POC Hemostasis System Could Help Prevent Maternal Deaths
- New Test Assesses Oxygen Delivering Ability of Red Blood Cells by Measuring Their Shape
- Personalized CBC Testing Could Help Diagnose Early-Stage Diseases in Healthy Individuals
- Non-Invasive Test Solution Determines Fetal RhD Status from Maternal Plasma
- First-Of-Its-Kind Smartphone Technology Noninvasively Measures Blood Hemoglobin Levels at POC
- Next Gen CBC and Sepsis Diagnostic System Targets Faster, Earlier, Easier Results
- Newly Discovered Blood Group System to Help Identify and Treat Rare Patients
- Blood Platelet Score Detects Previously Unmeasured Risk of Heart Attack and Stroke
- Automated Benchtop System to Bring Blood Testing To Anyone, Anywhere
- New Hematology Analyzers Deliver Combined ESR and CBC/DIFF Results in 60 Seconds
- Next Generation Instrument Screens for Hemoglobin Disorders in Newborns
Channels
Clinical Chemistry
view channel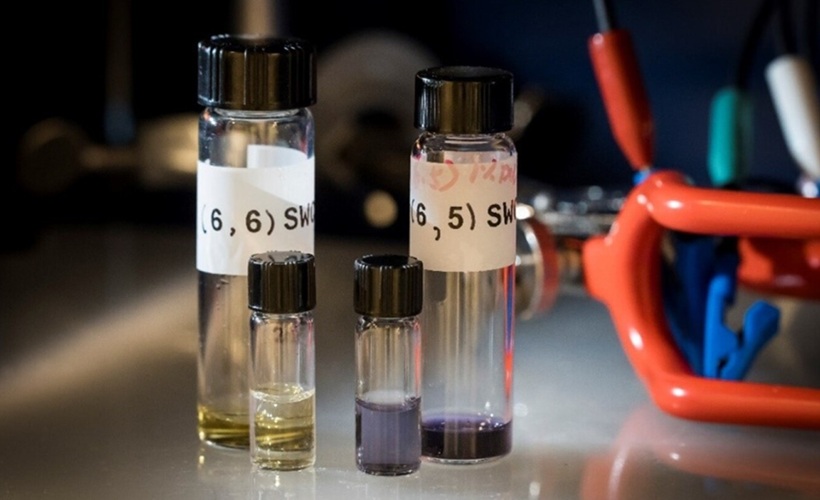
Carbon Nanotubes Help Build Highly Accurate Sensors for Continuous Health Monitoring
Current sensors can measure various health indicators, such as blood glucose levels, in the body. However, there is a need to develop more accurate and sensitive sensor materials that can detect lower... Read more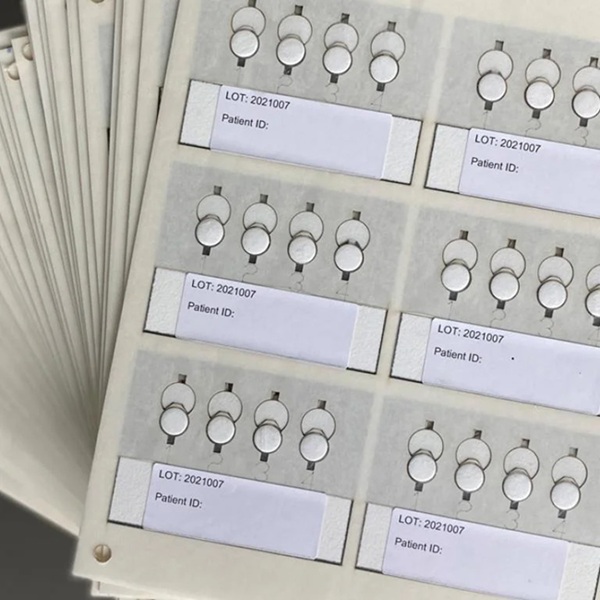
Paper-Based Device Boosts HIV Test Accuracy from Dried Blood Samples
In regions where access to clinics for routine blood tests presents financial and logistical obstacles, HIV patients are increasingly able to collect and send a drop of blood using paper-based devices... Read moreMolecular Diagnostics
view channel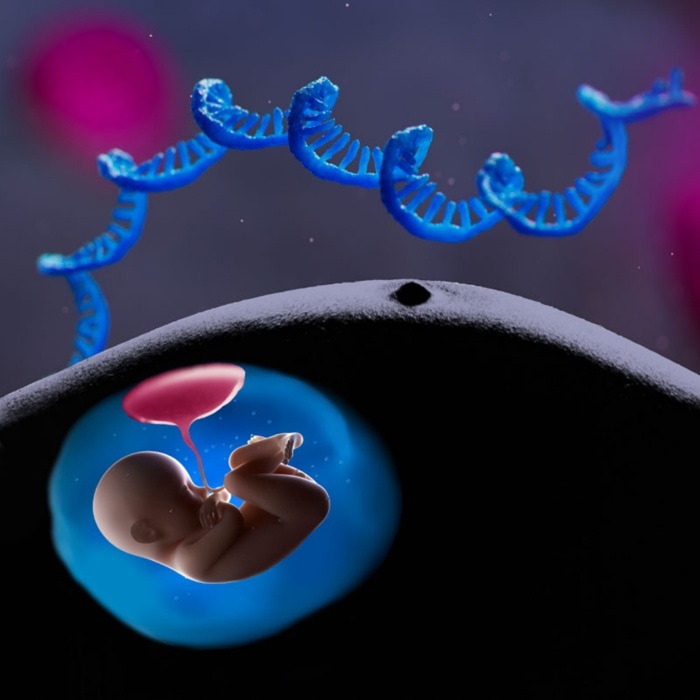
RNA-Based Blood Test Detects Preeclampsia Risk Months Before Symptoms
Preeclampsia remains a major cause of maternal morbidity and mortality, as well as preterm births. Despite current guidelines that aim to identify pregnant women at increased risk of preeclampsia using... Read more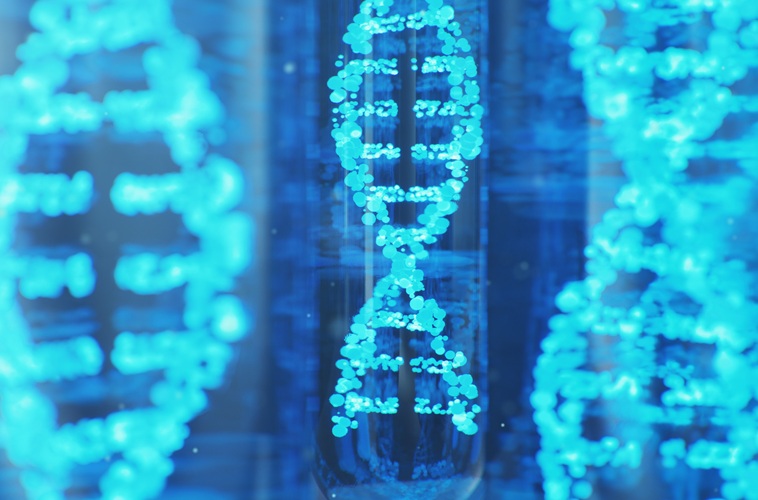
First Of Its Kind Test Uses microRNAs to Predict Toxicity from Cancer Therapy
Many men with early-stage prostate cancer receive stereotactic body radiotherapy (SBRT), a highly precise form of radiation treatment that is completed in just five sessions. Compared to traditional radiation,... Read more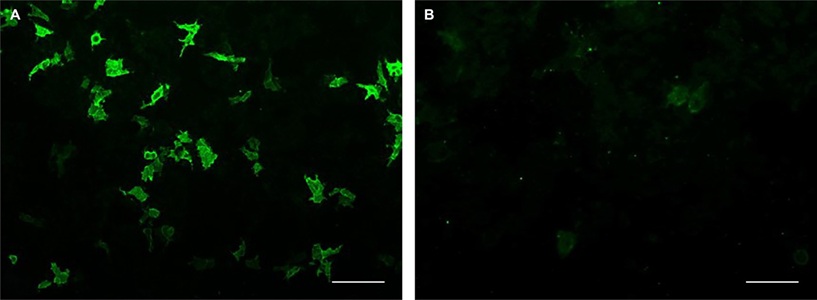
Novel Cell-Based Assay Provides Sensitive and Specific Autoantibody Detection in Demyelination
Anti-myelin-associated glycoprotein (MAG) antibodies serve as markers for an autoimmune demyelinating disorder that affects the peripheral nervous system, leading to sensory impairment. Anti-MAG-IgM antibodies... Read moreImmunology
view channel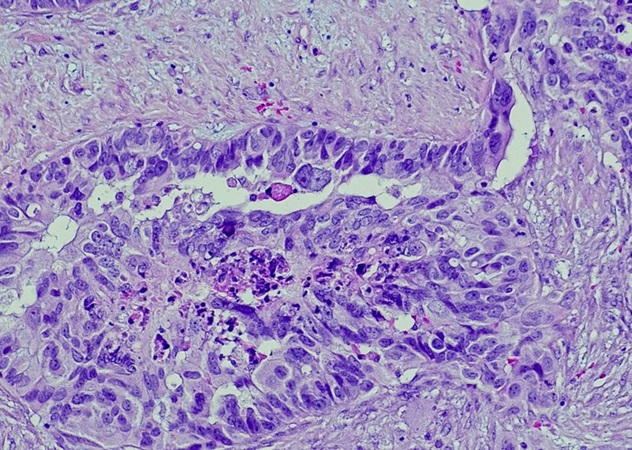
Stem Cell Test Predicts Treatment Outcome for Patients with Platinum-Resistant Ovarian Cancer
Epithelial ovarian cancer frequently responds to chemotherapy initially, but eventually, the tumor develops resistance to the therapy, leading to regrowth. This resistance is partially due to the activation... Read more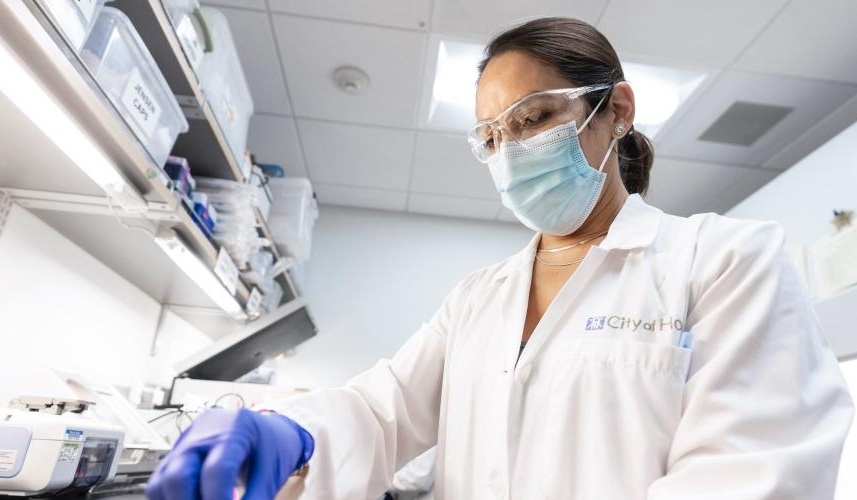
Machine Learning-Enabled Blood Test Predicts Immunotherapy Response in Lymphoma Patients
Chimeric antigen receptor (CAR) T-cell therapy has emerged as one of the most promising recent developments in the treatment of blood cancers. However, over half of non-Hodgkin lymphoma (NHL) patients... Read moreMicrobiology
view channel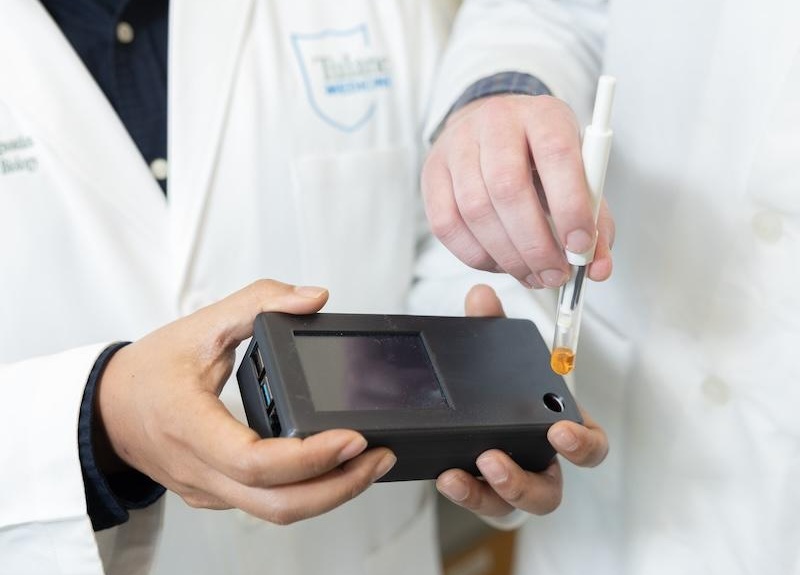
Handheld Device Deliver Low-Cost TB Results in Less Than One Hour
Tuberculosis (TB) remains the deadliest infectious disease globally, affecting an estimated 10 million people annually. In 2021, about 4.2 million TB cases went undiagnosed or unreported, mainly due to... Read more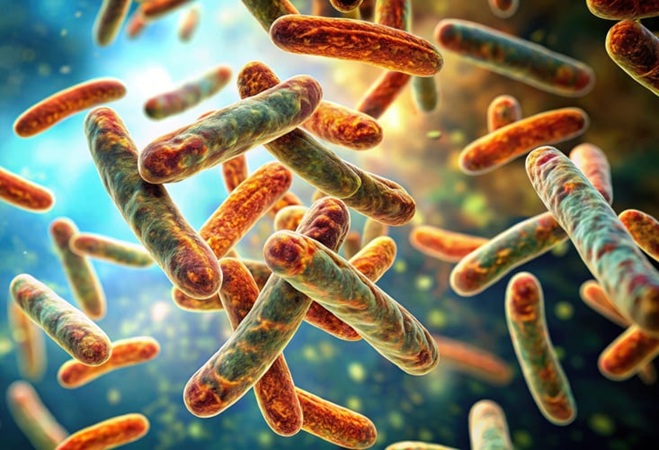
New AI-Based Method Improves Diagnosis of Drug-Resistant Infections
Drug-resistant infections, particularly those caused by deadly bacteria like tuberculosis and staphylococcus, are rapidly emerging as a global health emergency. These infections are more difficult to treat,... Read more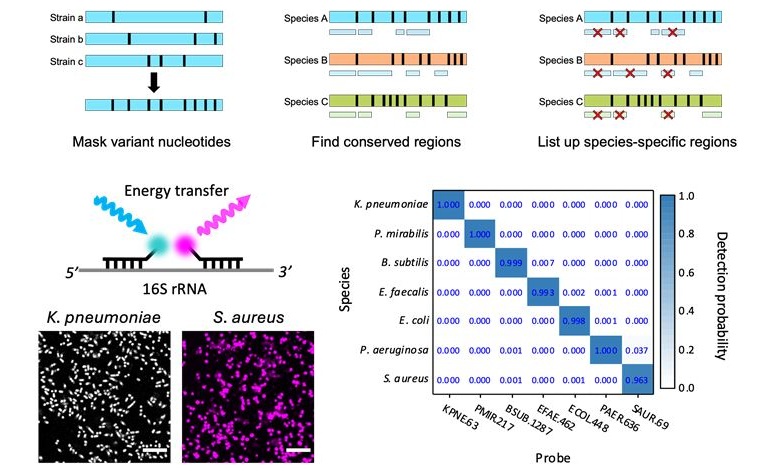
Breakthrough Diagnostic Technology Identifies Bacterial Infections with Almost 100% Accuracy within Three Hours
Rapid and precise identification of pathogenic microbes in patient samples is essential for the effective treatment of acute infectious diseases, such as sepsis. The fluorescence in situ hybridization... Read morePathology
view channel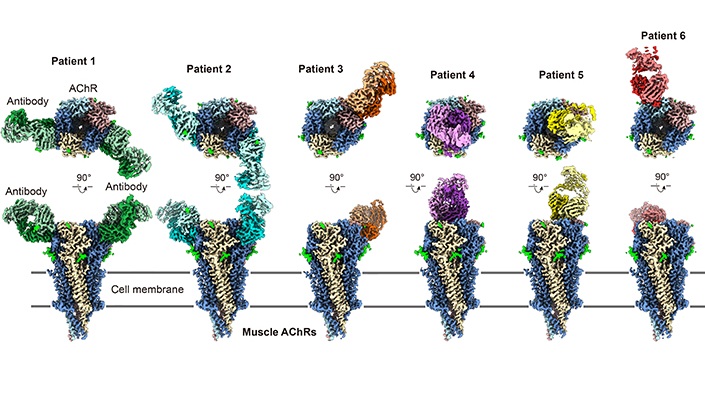
Advanced Imaging Reveals Mechanisms Causing Autoimmune Disease
Myasthenia gravis, an autoimmune disease, leads to muscle weakness that can affect a range of muscles, including those needed for basic actions like blinking, smiling, or moving. Researchers have long... Read more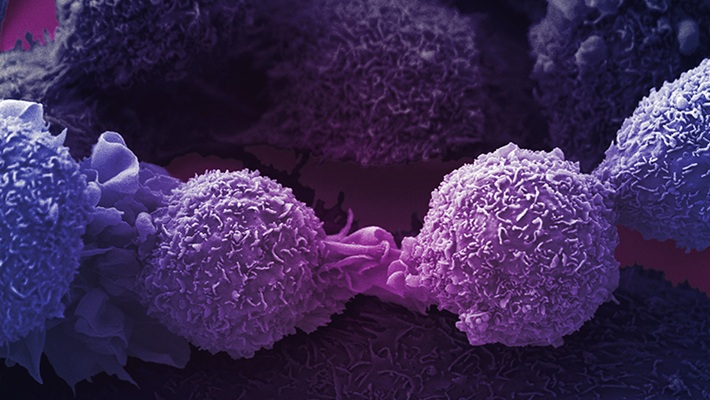
AI Model Effectively Predicts Patient Outcomes in Common Lung Cancer Type
Lung adenocarcinoma, the most common form of non-small cell lung cancer (NSCLC), typically adopts one of six distinct growth patterns, often combining multiple patterns within a single tumor.... Read moreTechnology
view channel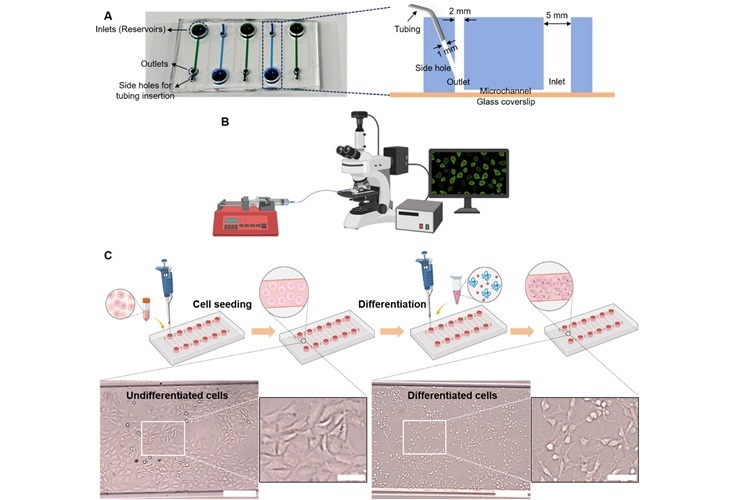
Pain-On-A-Chip Microfluidic Device Determines Types of Chronic Pain from Blood Samples
Chronic pain is a widespread condition that remains difficult to manage, and existing clinical methods for its treatment rely largely on self-reporting, which can be subjective and especially problematic... Read more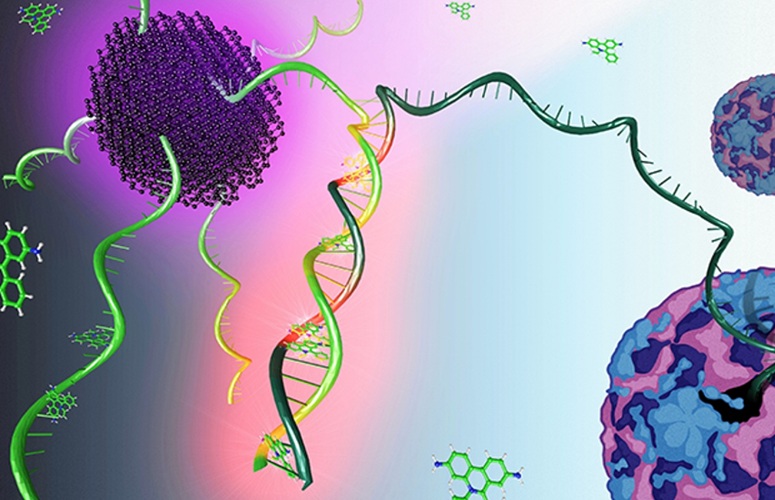
Innovative, Label-Free Ratiometric Fluorosensor Enables More Sensitive Viral RNA Detection
Viruses present a major global health risk, as demonstrated by recent pandemics, making early detection and identification essential for preventing new outbreaks. While traditional detection methods are... Read moreIndustry
view channel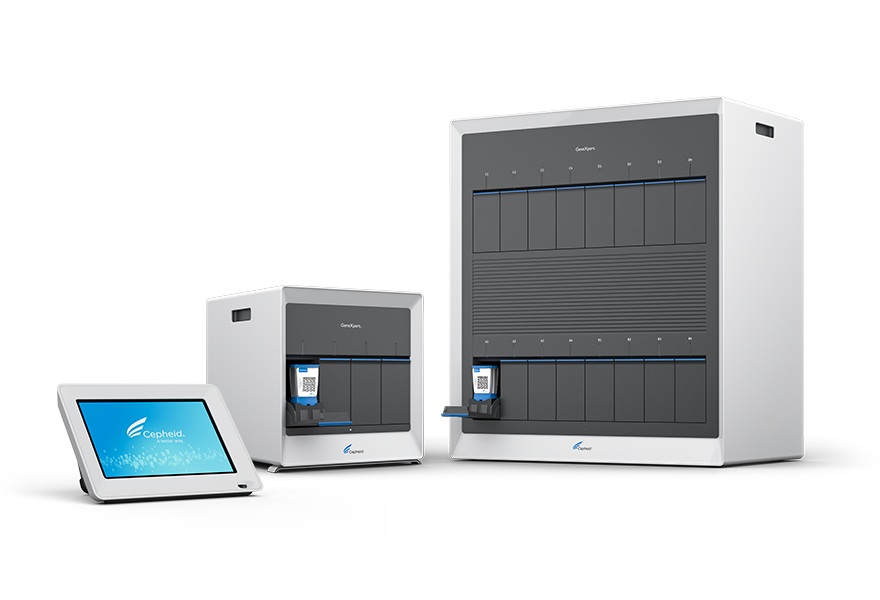
Cepheid and Oxford Nanopore Technologies Partner on Advancing Automated Sequencing-Based Solutions
Cepheid (Sunnyvale, CA, USA), a leading molecular diagnostics company, and Oxford Nanopore Technologies (Oxford, UK), the company behind a new generation of sequencing-based molecular analysis technologies,... Read more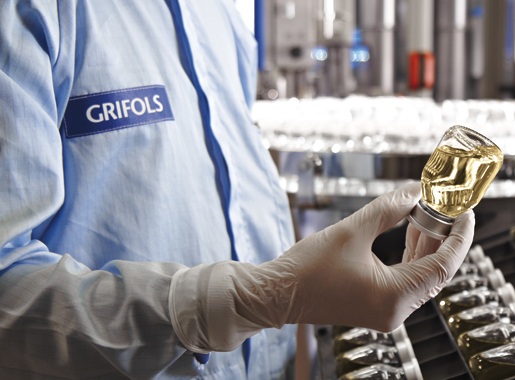
Grifols and Tecan’s IBL Collaborate on Advanced Biomarker Panels
Grifols (Barcelona, Spain), one of the world’s leading producers of plasma-derived medicines and innovative diagnostic solutions, is expanding its offer in clinical diagnostics through a strategic partnership... Read more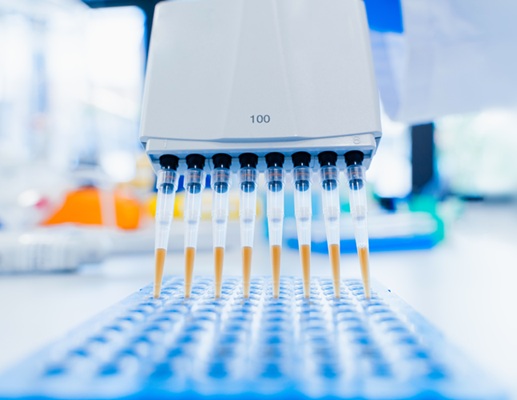