Blood Biomarker Discovered for Autism Spectrum Disorder
By LabMedica International staff writers Posted on 11 Mar 2021 |
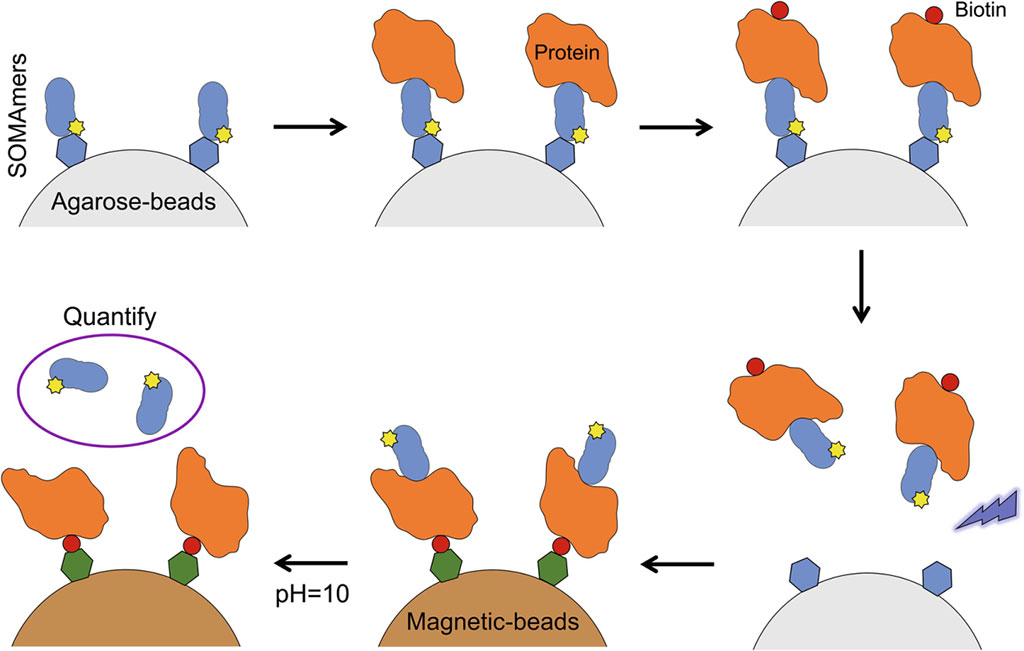
Image: The principle of SOMAscan Assay that allows for the simultaneous detection of 1,300 human proteins using SOMAmer (Slow Off-rate Modified Aptamer) protein-binding reagents (Photo courtesy of SomaLogic).
Autism spectrum disorder (ASD), a heterogeneous neurodevelopmental disorder, is characterized by deficits in social communication and social interaction, with restricted, repetitive patterns of behavior, interests, or activities. ASD impacts at least one out of every 59 children in the USA, although this is likely an underestimation.
Many blood-based biomarker candidates have been investigated, including neurotransmitters, cytokines, and markers of mitochondrial dysfunction, oxidative stress, and impaired methylation. However, given the prevalence of ASD, the use of machine learning to incorporate demographic and clinical data into the analysis could more powerfully examine disease status and symptom severity.
Medical Scientists at the Johnson Center for Child Health and Development (Austin, TX, USA) enrolled a total of 154 male pediatric subjects. The ASD group was comprised of 76 subjects with a mean age of 5.6 years ± 1.7 years. The typically developing (TD) group was comprised of 78 subjects with a mean age of 5.7 years ± 2.0 years. The ethnic breakdown was as follows: 73 White/Caucasian, 32 Hispanic/Latino, 17 African American/Black, five Asian or Pacific Islander, 23 multiple ethnicities or other, and four not reported.
A fasting blood draw was performed on ASD and TD subjects between the hours of 8–10 AM in a 3.5 mL Serum Separation Tube using standard venipuncture technique. The SOMAScan platform 1.3k was used for analysis, and assays were run (SomaLogic, Boulder, CO, USA). SOMAmer aptamer reagents consist of short single-stranded DNA sequences with ‘protein-like’ appendages that allow tight and specific binding to protein targets. More than 1,100 proteins were examined using the SomaLogic protein analysis platform. A panel of nine proteins was identified as optimal for predicting ASD using three computational methods. The scientists then evaluated the biomarker panel for quality using machine learning methods.
The team reported that five proteins were common to all three prediction models used: mitogen-activated protein kinase 14 (MAPK14), immunoglobulin D (IgD), dermatopontin (DERM), ephrin type-B receptor 2 (EPHB2), and soluble urokinase-type plasminogen activator receptor (suPAR). These five proteins were defined as core proteins. Four proteins providing additive power were combined with the five core proteins, and together, the nine proteins resulted in an AUC of 86% (sensitivity 83%; specificity 84%). These proteins have pathway significance related to a number of processes, including negative regulation of CD8+, alpha-beta T cell proliferation, immune response, neuron projection retraction, MAPK14 activity, and glutamate receptor signaling, all of which have previously been associated with ASD.
Dwight German, PhD, a Professor of Psychiatry and senior author of the study, said, “The more significantly affected the child is, the higher or lower than normal the blood biomarker is. Ideally, there will be a day when a child is identified using blood biomarkers as being at risk for developing ASD and therapies can be started immediately. That would help the child develop skills to optimize their communication and learning.”
The authors concluded that all nine proteins were significantly different in ASD compared with TD boys, and were significantly correlated with ASD severity as measured by Autism Diagnostic Observation Schedule (ADOS) total scores. Using machine learning methods, a panel of serum proteins was identified that may be useful as a blood biomarker for ASD in boys. The study was published on February 24, 2021 in the journal PLOS ONE.
Related Links:
Johnson Center for Child Health and Development
SomaLogic
Many blood-based biomarker candidates have been investigated, including neurotransmitters, cytokines, and markers of mitochondrial dysfunction, oxidative stress, and impaired methylation. However, given the prevalence of ASD, the use of machine learning to incorporate demographic and clinical data into the analysis could more powerfully examine disease status and symptom severity.
Medical Scientists at the Johnson Center for Child Health and Development (Austin, TX, USA) enrolled a total of 154 male pediatric subjects. The ASD group was comprised of 76 subjects with a mean age of 5.6 years ± 1.7 years. The typically developing (TD) group was comprised of 78 subjects with a mean age of 5.7 years ± 2.0 years. The ethnic breakdown was as follows: 73 White/Caucasian, 32 Hispanic/Latino, 17 African American/Black, five Asian or Pacific Islander, 23 multiple ethnicities or other, and four not reported.
A fasting blood draw was performed on ASD and TD subjects between the hours of 8–10 AM in a 3.5 mL Serum Separation Tube using standard venipuncture technique. The SOMAScan platform 1.3k was used for analysis, and assays were run (SomaLogic, Boulder, CO, USA). SOMAmer aptamer reagents consist of short single-stranded DNA sequences with ‘protein-like’ appendages that allow tight and specific binding to protein targets. More than 1,100 proteins were examined using the SomaLogic protein analysis platform. A panel of nine proteins was identified as optimal for predicting ASD using three computational methods. The scientists then evaluated the biomarker panel for quality using machine learning methods.
The team reported that five proteins were common to all three prediction models used: mitogen-activated protein kinase 14 (MAPK14), immunoglobulin D (IgD), dermatopontin (DERM), ephrin type-B receptor 2 (EPHB2), and soluble urokinase-type plasminogen activator receptor (suPAR). These five proteins were defined as core proteins. Four proteins providing additive power were combined with the five core proteins, and together, the nine proteins resulted in an AUC of 86% (sensitivity 83%; specificity 84%). These proteins have pathway significance related to a number of processes, including negative regulation of CD8+, alpha-beta T cell proliferation, immune response, neuron projection retraction, MAPK14 activity, and glutamate receptor signaling, all of which have previously been associated with ASD.
Dwight German, PhD, a Professor of Psychiatry and senior author of the study, said, “The more significantly affected the child is, the higher or lower than normal the blood biomarker is. Ideally, there will be a day when a child is identified using blood biomarkers as being at risk for developing ASD and therapies can be started immediately. That would help the child develop skills to optimize their communication and learning.”
The authors concluded that all nine proteins were significantly different in ASD compared with TD boys, and were significantly correlated with ASD severity as measured by Autism Diagnostic Observation Schedule (ADOS) total scores. Using machine learning methods, a panel of serum proteins was identified that may be useful as a blood biomarker for ASD in boys. The study was published on February 24, 2021 in the journal PLOS ONE.
Related Links:
Johnson Center for Child Health and Development
SomaLogic
Latest Clinical Chem. News
- Carbon Nanotubes Help Build Highly Accurate Sensors for Continuous Health Monitoring
- Paper-Based Device Boosts HIV Test Accuracy from Dried Blood Samples
- AI-Powered Raman Spectroscopy Method Enables Rapid Drug Detection in Blood
- Novel LC-MS/MS Assay Detects Low Creatinine in Sweat and Saliva
- Biosensing Technology Breakthrough Paves Way for New Methods of Early Disease Detection
- New Saliva Test Rapidly Identifies Paracetamol Overdose
- POC Saliva Testing Device Predicts Heart Failure in 15 Minutes
- Screening Tool Detects Multiple Health Conditions from Single Blood Drop
- Integrated Chemistry and Immunoassay Analyzer with Extensive Assay Menu Offers Flexibility, Scalability and Data Commutability
- Rapid Drug Test to Improve Treatment for Patients Presenting to Hospital
- AI Model Detects Cancer at Lightning Speed through Sugar Analyses
- First-Ever Blood-Powered Chip Offers Real-Time Health Monitoring
- New ADLM Guidance Provides Expert Recommendations on Clinical Testing For Respiratory Viral Infections
- 3D Printed Point-Of-Care Mass Spectrometer Outperforms State-Of-The-Art Models
- POC Biomedical Test Spins Water Droplet Using Sound Waves for Cancer Detection
- Highly Reliable Cell-Based Assay Enables Accurate Diagnosis of Endocrine Diseases
Channels
Molecular Diagnostics
view channel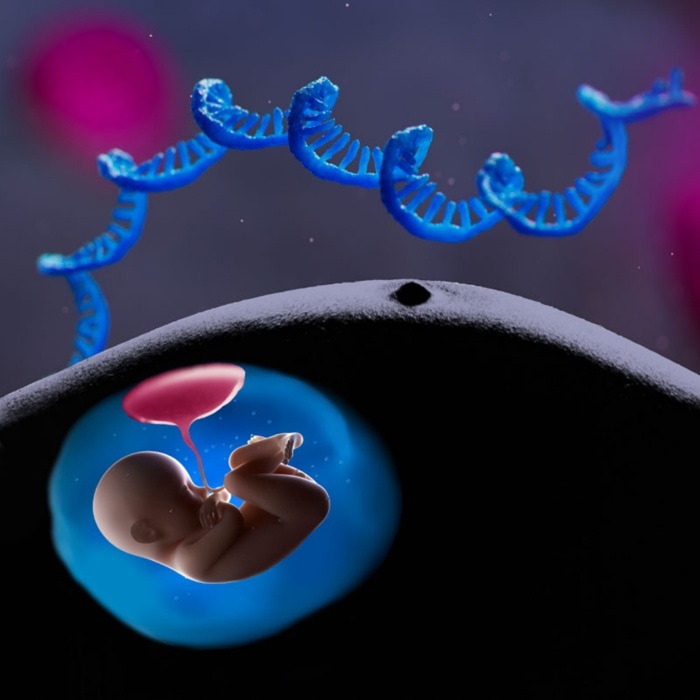
RNA-Based Blood Test Detects Preeclampsia Risk Months Before Symptoms
Preeclampsia remains a major cause of maternal morbidity and mortality, as well as preterm births. Despite current guidelines that aim to identify pregnant women at increased risk of preeclampsia using... Read more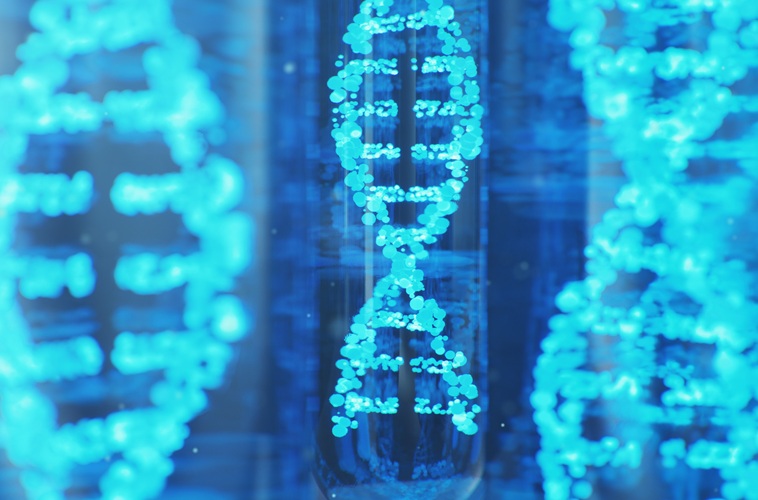
First Of Its Kind Test Uses microRNAs to Predict Toxicity from Cancer Therapy
Many men with early-stage prostate cancer receive stereotactic body radiotherapy (SBRT), a highly precise form of radiation treatment that is completed in just five sessions. Compared to traditional radiation,... Read more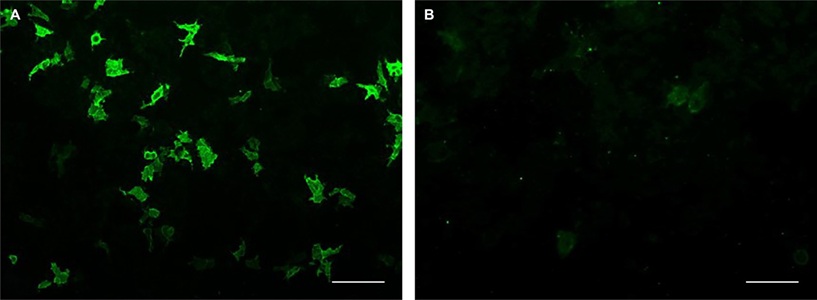
Novel Cell-Based Assay Provides Sensitive and Specific Autoantibody Detection in Demyelination
Anti-myelin-associated glycoprotein (MAG) antibodies serve as markers for an autoimmune demyelinating disorder that affects the peripheral nervous system, leading to sensory impairment. Anti-MAG-IgM antibodies... Read moreHematology
view channel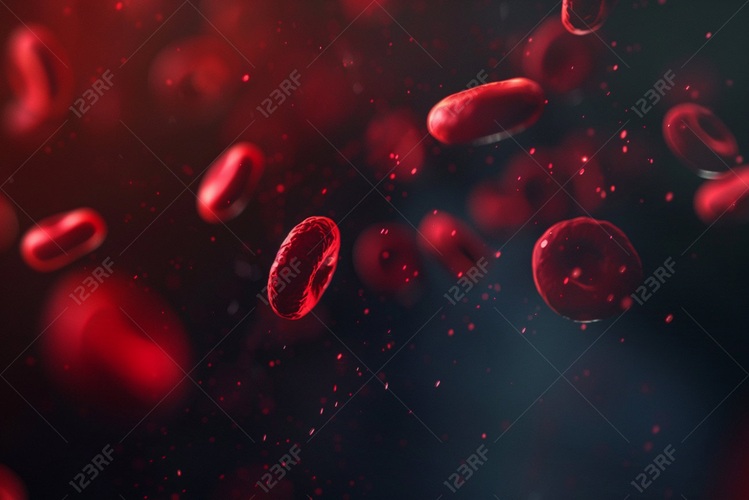
New Scoring System Predicts Risk of Developing Cancer from Common Blood Disorder
Clonal cytopenia of undetermined significance (CCUS) is a blood disorder commonly found in older adults, characterized by mutations in blood cells and a low blood count, but without any obvious cause or... Read more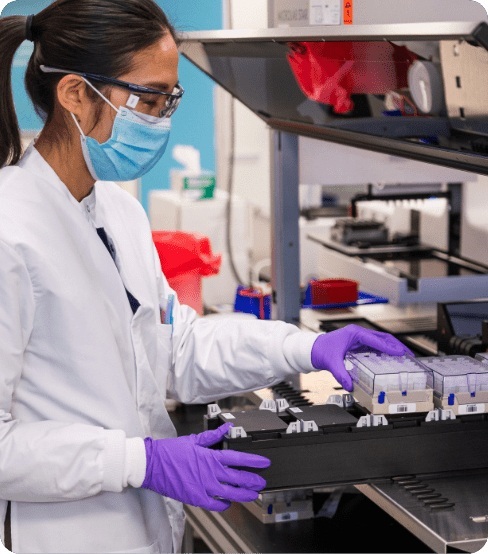
Non-Invasive Prenatal Test for Fetal RhD Status Demonstrates 100% Accuracy
In the United States, approximately 15% of pregnant individuals are RhD-negative. However, in about 40% of these cases, the fetus is also RhD-negative, making the administration of RhoGAM unnecessary.... Read moreImmunology
view channel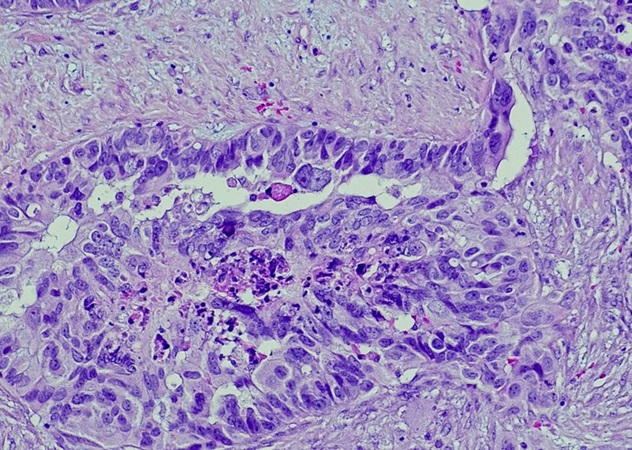
Stem Cell Test Predicts Treatment Outcome for Patients with Platinum-Resistant Ovarian Cancer
Epithelial ovarian cancer frequently responds to chemotherapy initially, but eventually, the tumor develops resistance to the therapy, leading to regrowth. This resistance is partially due to the activation... Read more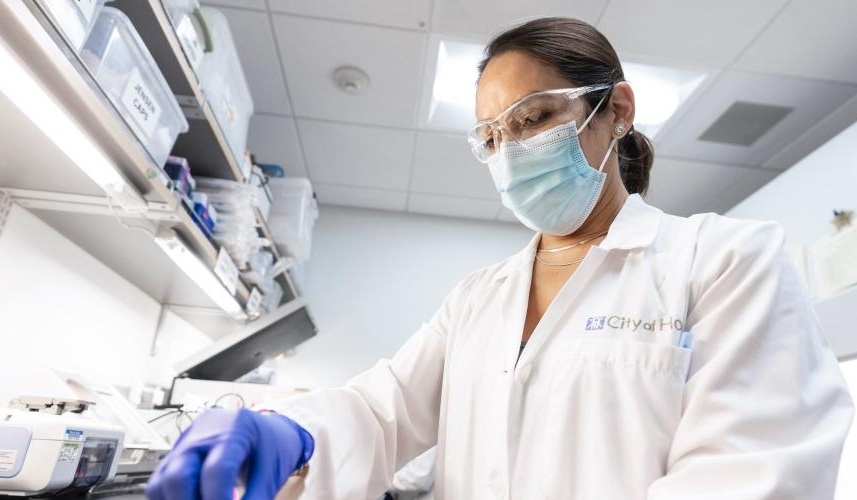
Machine Learning-Enabled Blood Test Predicts Immunotherapy Response in Lymphoma Patients
Chimeric antigen receptor (CAR) T-cell therapy has emerged as one of the most promising recent developments in the treatment of blood cancers. However, over half of non-Hodgkin lymphoma (NHL) patients... Read moreMicrobiology
view channel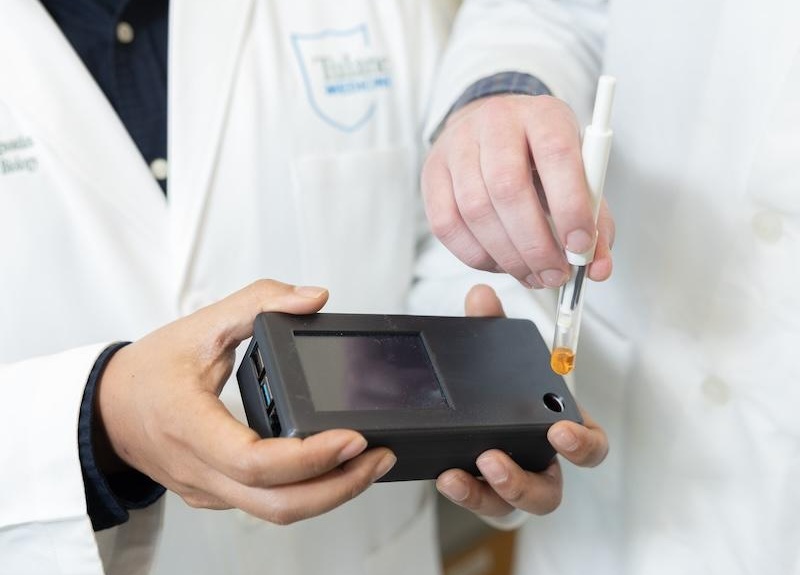
Handheld Device Deliver Low-Cost TB Results in Less Than One Hour
Tuberculosis (TB) remains the deadliest infectious disease globally, affecting an estimated 10 million people annually. In 2021, about 4.2 million TB cases went undiagnosed or unreported, mainly due to... Read more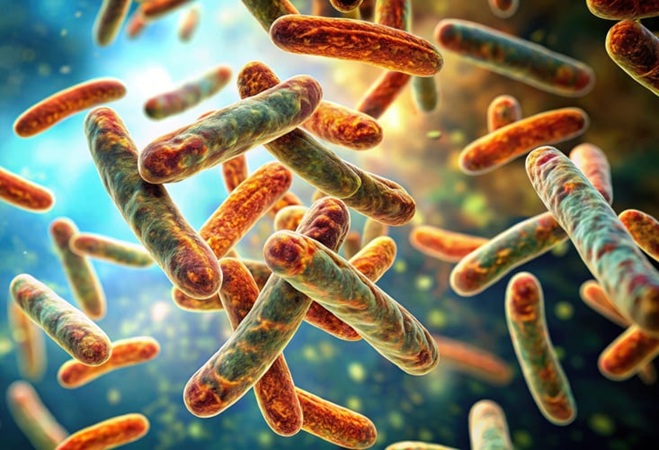
New AI-Based Method Improves Diagnosis of Drug-Resistant Infections
Drug-resistant infections, particularly those caused by deadly bacteria like tuberculosis and staphylococcus, are rapidly emerging as a global health emergency. These infections are more difficult to treat,... Read more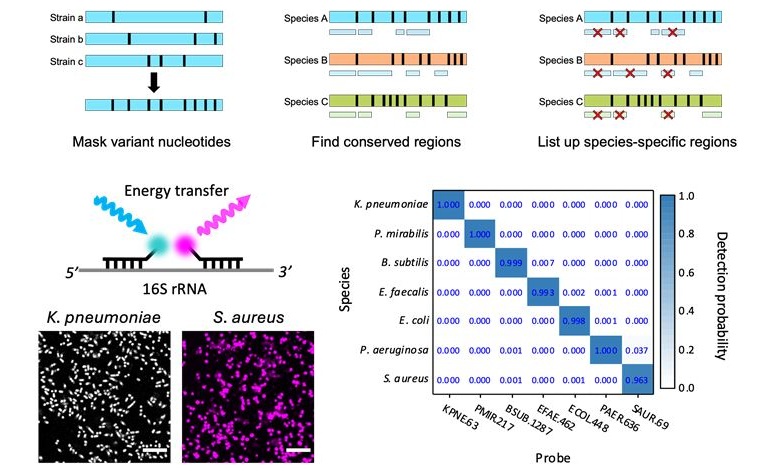
Breakthrough Diagnostic Technology Identifies Bacterial Infections with Almost 100% Accuracy within Three Hours
Rapid and precise identification of pathogenic microbes in patient samples is essential for the effective treatment of acute infectious diseases, such as sepsis. The fluorescence in situ hybridization... Read morePathology
view channel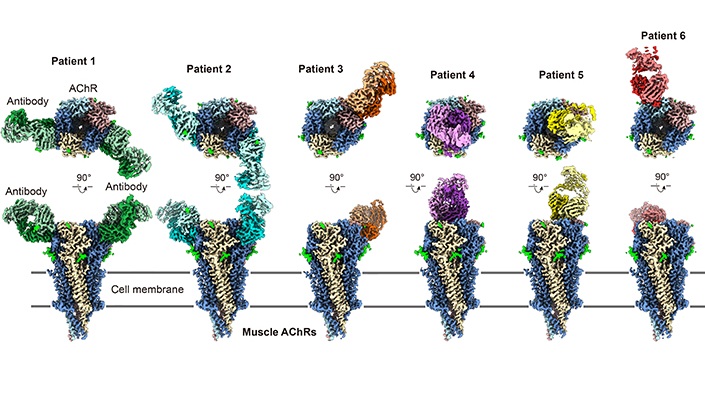
Advanced Imaging Reveals Mechanisms Causing Autoimmune Disease
Myasthenia gravis, an autoimmune disease, leads to muscle weakness that can affect a range of muscles, including those needed for basic actions like blinking, smiling, or moving. Researchers have long... Read more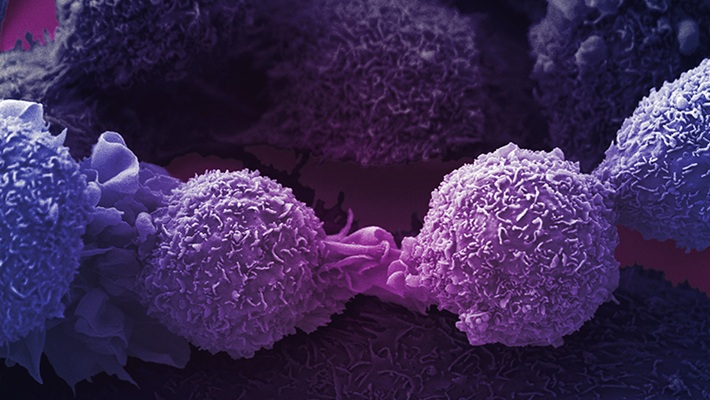
AI Model Effectively Predicts Patient Outcomes in Common Lung Cancer Type
Lung adenocarcinoma, the most common form of non-small cell lung cancer (NSCLC), typically adopts one of six distinct growth patterns, often combining multiple patterns within a single tumor.... Read moreTechnology
view channel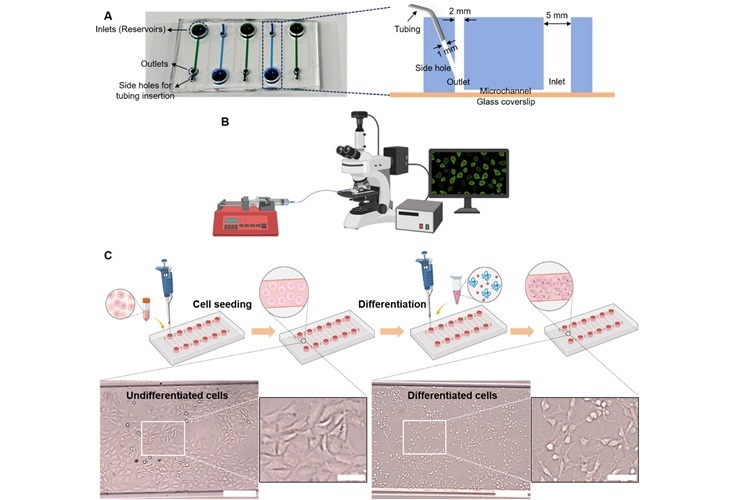
Pain-On-A-Chip Microfluidic Device Determines Types of Chronic Pain from Blood Samples
Chronic pain is a widespread condition that remains difficult to manage, and existing clinical methods for its treatment rely largely on self-reporting, which can be subjective and especially problematic... Read more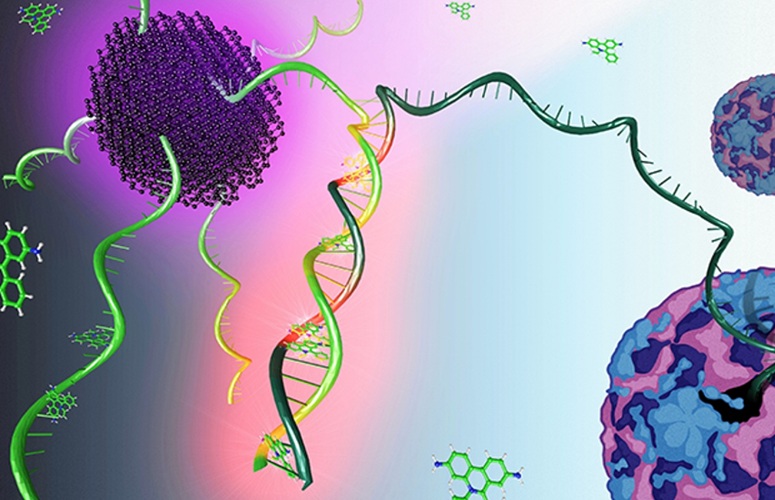
Innovative, Label-Free Ratiometric Fluorosensor Enables More Sensitive Viral RNA Detection
Viruses present a major global health risk, as demonstrated by recent pandemics, making early detection and identification essential for preventing new outbreaks. While traditional detection methods are... Read moreIndustry
view channel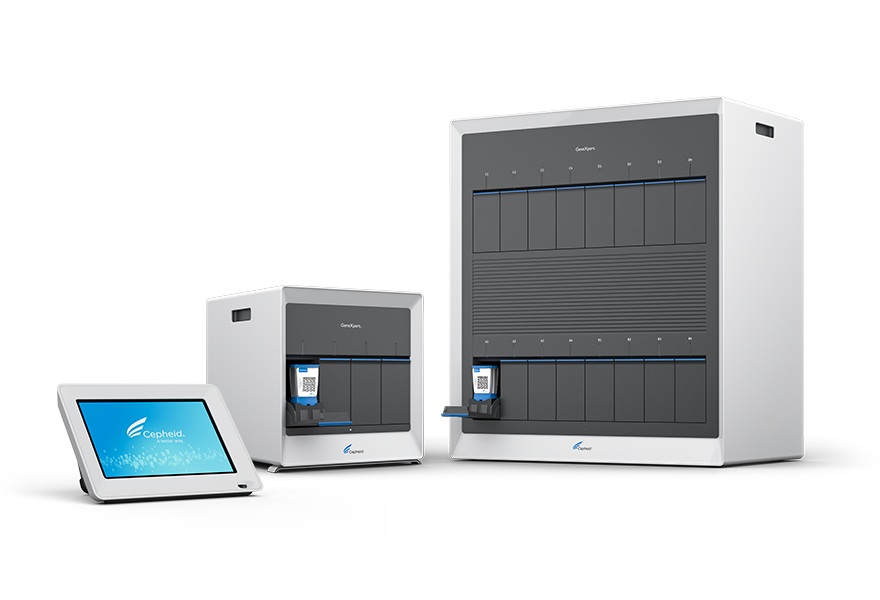
Cepheid and Oxford Nanopore Technologies Partner on Advancing Automated Sequencing-Based Solutions
Cepheid (Sunnyvale, CA, USA), a leading molecular diagnostics company, and Oxford Nanopore Technologies (Oxford, UK), the company behind a new generation of sequencing-based molecular analysis technologies,... Read more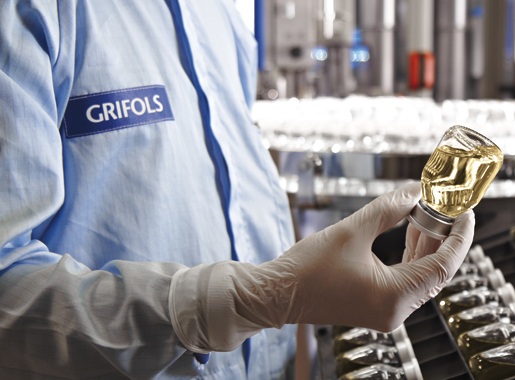
Grifols and Tecan’s IBL Collaborate on Advanced Biomarker Panels
Grifols (Barcelona, Spain), one of the world’s leading producers of plasma-derived medicines and innovative diagnostic solutions, is expanding its offer in clinical diagnostics through a strategic partnership... Read more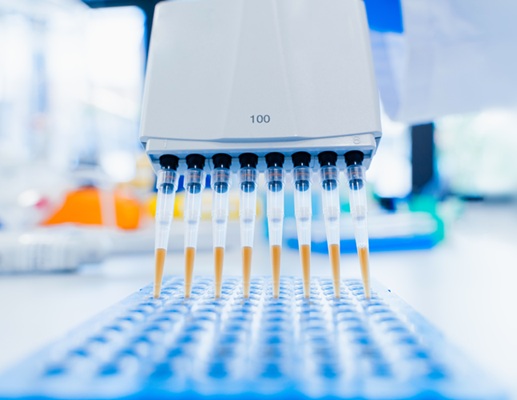