Acute Myeloid Leukemia Diagnosed by Convolutional Neural Networks
By LabMedica International staff writers Posted on 27 Nov 2019 |
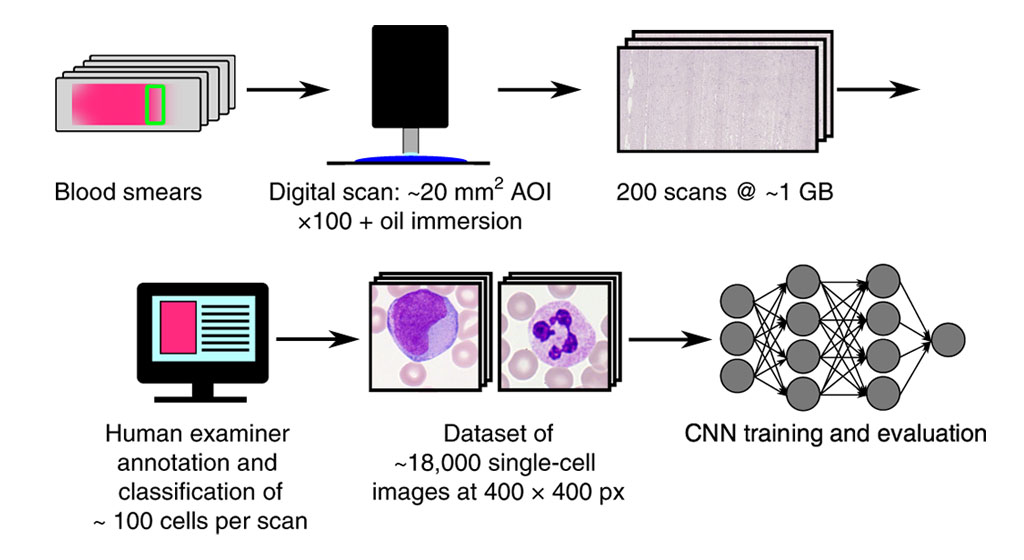
Image: Schematic diagram of how the deep learning algorithm classifies leukocytes in a blood smear in an automated and standardized way (Photo courtesy of Helmholtz Zentrum München / Dr. Carsten Marr)
Every day, millions of single blood cells are evaluated for disease diagnostics in medical laboratories and clinics. Most of this repetitive task is still done manually by trained cytologists who inspect cells in stained blood smears and classify them into roughly 15 different categories.
Scientists have now shown that deep learning algorithms perform similar to human experts when classifying blood samples from patients suffering from acute myeloid leukemia (AML). Their proof of concept study paves the way for an automated, standardized and on-hand sample analysis in the near future.
Scientists from the Helmholtz Zentrum München (Neuherberg, Germany) and their colleagues compiled an annotated image dataset of over 18,000 white blood cells, use it to train a convolutional neural network for leukocyte classification and evaluate the network’s performance by comparing to inter- and intra-expert variability. They used images which were extracted from blood smears of 100 patients suffering from the aggressive blood disease AML and 100 controls. The new AI-driven approach was then evaluated by comparing its performance with the accuracy of human experts.
The network classifies the most important cell types with high accuracy. It also allowed the investigators to decide two clinically relevant questions with human-level performance: (1) if a given cell has blast character and (2) if it belongs to the cell types normally present in non-pathological blood smears. The result showed that the AI-driven solution is able to identify diagnostic blast cells at least as good as a trained cytologist expert.
Carsten Marr, PhD, a computational stem cell biologists and the senior author of the study, said, “To bring our approach to clinics, digitization of patients' blood samples has to become routine. Algorithms have to be trained with samples from different sources to cope with the inherent heterogeneity in sample preparation and staining. Together with our partners we could prove that deep learning algorithms show a similar performance as human cytologists. In a next step, we will evaluate how well other disease characteristics, such as genetic mutations or translocations, can be predicted with this new AI-driven method.”
The authors concluded that their approach holds the potential to be used as a classification aid for examining much larger numbers of cells in a smear than can usually is done by a human expert. This will allow clinicians to recognize malignant cell populations with lower prevalence at an earlier stage of the disease. The study was published on November 12, 2019 in the journal Nature Machine Intelligence.
Related Links:
Helmholtz Zentrum München
Scientists have now shown that deep learning algorithms perform similar to human experts when classifying blood samples from patients suffering from acute myeloid leukemia (AML). Their proof of concept study paves the way for an automated, standardized and on-hand sample analysis in the near future.
Scientists from the Helmholtz Zentrum München (Neuherberg, Germany) and their colleagues compiled an annotated image dataset of over 18,000 white blood cells, use it to train a convolutional neural network for leukocyte classification and evaluate the network’s performance by comparing to inter- and intra-expert variability. They used images which were extracted from blood smears of 100 patients suffering from the aggressive blood disease AML and 100 controls. The new AI-driven approach was then evaluated by comparing its performance with the accuracy of human experts.
The network classifies the most important cell types with high accuracy. It also allowed the investigators to decide two clinically relevant questions with human-level performance: (1) if a given cell has blast character and (2) if it belongs to the cell types normally present in non-pathological blood smears. The result showed that the AI-driven solution is able to identify diagnostic blast cells at least as good as a trained cytologist expert.
Carsten Marr, PhD, a computational stem cell biologists and the senior author of the study, said, “To bring our approach to clinics, digitization of patients' blood samples has to become routine. Algorithms have to be trained with samples from different sources to cope with the inherent heterogeneity in sample preparation and staining. Together with our partners we could prove that deep learning algorithms show a similar performance as human cytologists. In a next step, we will evaluate how well other disease characteristics, such as genetic mutations or translocations, can be predicted with this new AI-driven method.”
The authors concluded that their approach holds the potential to be used as a classification aid for examining much larger numbers of cells in a smear than can usually is done by a human expert. This will allow clinicians to recognize malignant cell populations with lower prevalence at an earlier stage of the disease. The study was published on November 12, 2019 in the journal Nature Machine Intelligence.
Related Links:
Helmholtz Zentrum München
Latest Hematology News
- New Scoring System Predicts Risk of Developing Cancer from Common Blood Disorder
- Non-Invasive Prenatal Test for Fetal RhD Status Demonstrates 100% Accuracy
- WBC Count Could Predict Severity of COVID-19 Symptoms
- New Platelet Counting Technology to Help Labs Prevent Diagnosis Errors
- Streamlined Approach to Testing for Heparin-Induced Thrombocytopenia Improves Diagnostic Accuracy
- POC Hemostasis System Could Help Prevent Maternal Deaths
- New Test Assesses Oxygen Delivering Ability of Red Blood Cells by Measuring Their Shape
- Personalized CBC Testing Could Help Diagnose Early-Stage Diseases in Healthy Individuals
- Non-Invasive Test Solution Determines Fetal RhD Status from Maternal Plasma
- First-Of-Its-Kind Smartphone Technology Noninvasively Measures Blood Hemoglobin Levels at POC
- Next Gen CBC and Sepsis Diagnostic System Targets Faster, Earlier, Easier Results
- Newly Discovered Blood Group System to Help Identify and Treat Rare Patients
- Blood Platelet Score Detects Previously Unmeasured Risk of Heart Attack and Stroke
- Automated Benchtop System to Bring Blood Testing To Anyone, Anywhere
- New Hematology Analyzers Deliver Combined ESR and CBC/DIFF Results in 60 Seconds
- Next Generation Instrument Screens for Hemoglobin Disorders in Newborns
Channels
Clinical Chemistry
view channel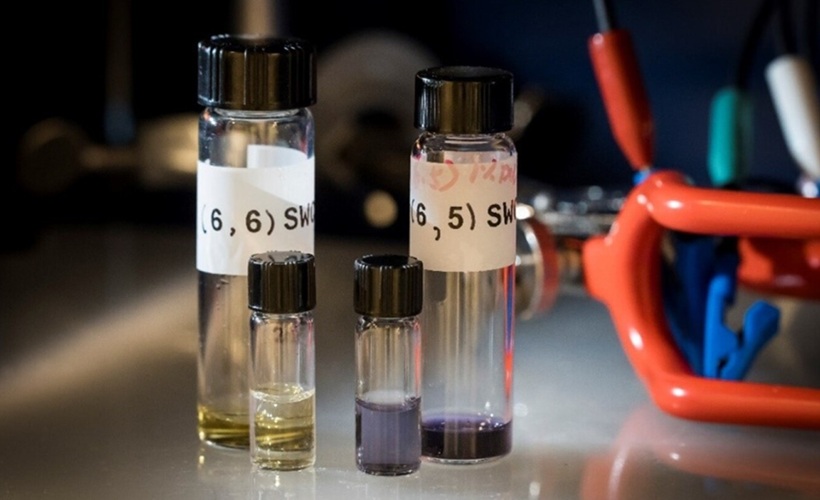
Carbon Nanotubes Help Build Highly Accurate Sensors for Continuous Health Monitoring
Current sensors can measure various health indicators, such as blood glucose levels, in the body. However, there is a need to develop more accurate and sensitive sensor materials that can detect lower... Read more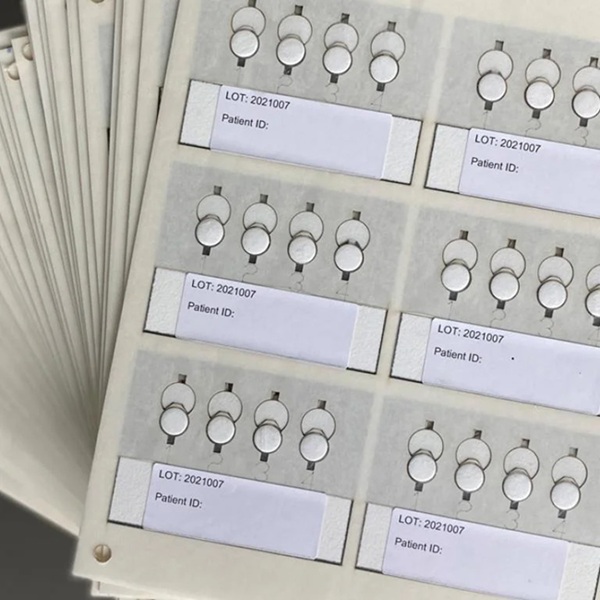
Paper-Based Device Boosts HIV Test Accuracy from Dried Blood Samples
In regions where access to clinics for routine blood tests presents financial and logistical obstacles, HIV patients are increasingly able to collect and send a drop of blood using paper-based devices... Read moreMolecular Diagnostics
view channel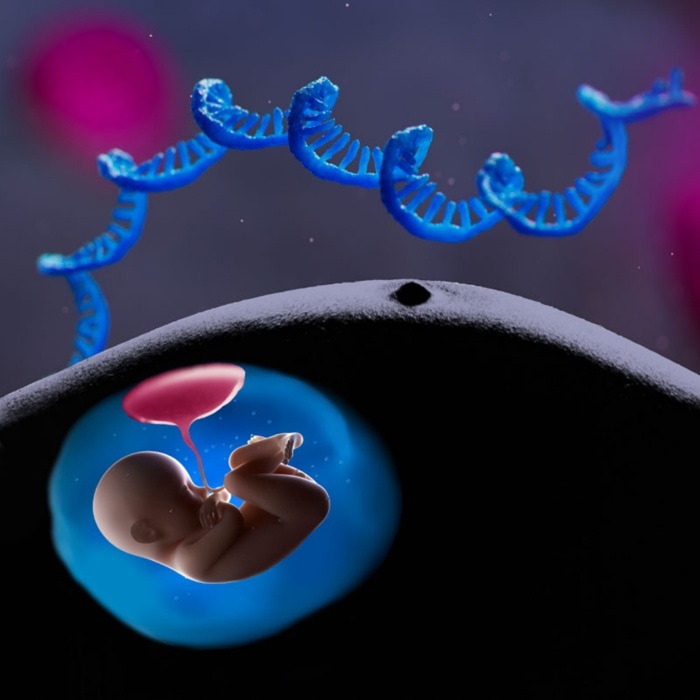
RNA-Based Blood Test Detects Preeclampsia Risk Months Before Symptoms
Preeclampsia remains a major cause of maternal morbidity and mortality, as well as preterm births. Despite current guidelines that aim to identify pregnant women at increased risk of preeclampsia using... Read more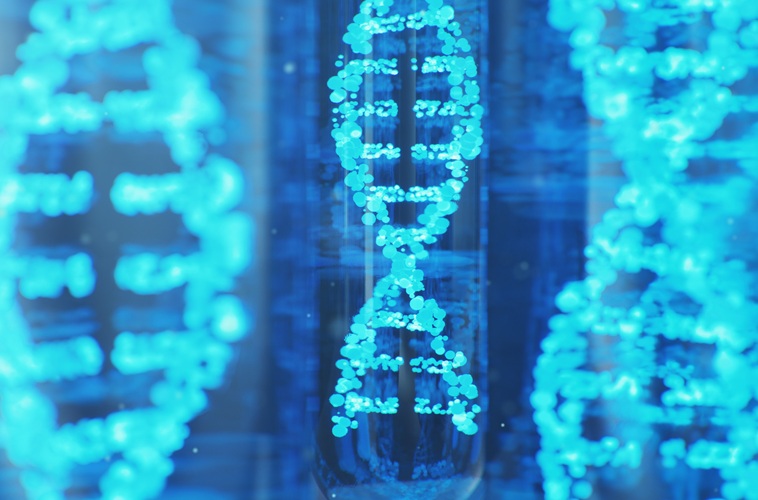
First Of Its Kind Test Uses microRNAs to Predict Toxicity from Cancer Therapy
Many men with early-stage prostate cancer receive stereotactic body radiotherapy (SBRT), a highly precise form of radiation treatment that is completed in just five sessions. Compared to traditional radiation,... Read more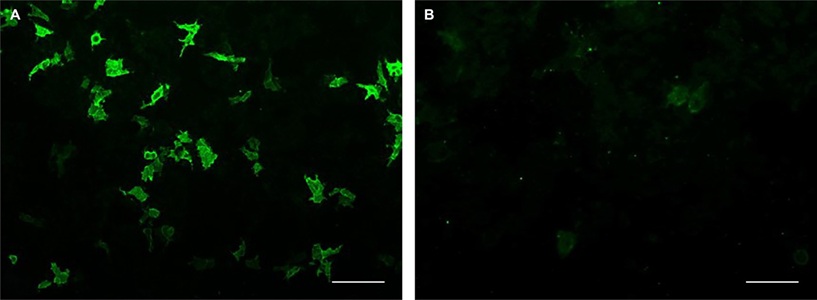
Novel Cell-Based Assay Provides Sensitive and Specific Autoantibody Detection in Demyelination
Anti-myelin-associated glycoprotein (MAG) antibodies serve as markers for an autoimmune demyelinating disorder that affects the peripheral nervous system, leading to sensory impairment. Anti-MAG-IgM antibodies... Read moreImmunology
view channel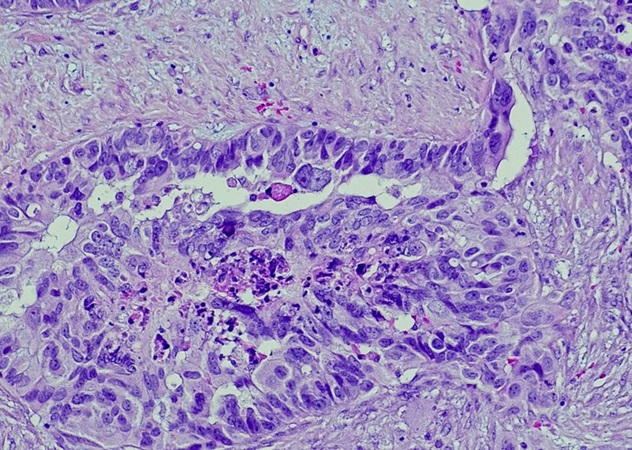
Stem Cell Test Predicts Treatment Outcome for Patients with Platinum-Resistant Ovarian Cancer
Epithelial ovarian cancer frequently responds to chemotherapy initially, but eventually, the tumor develops resistance to the therapy, leading to regrowth. This resistance is partially due to the activation... Read more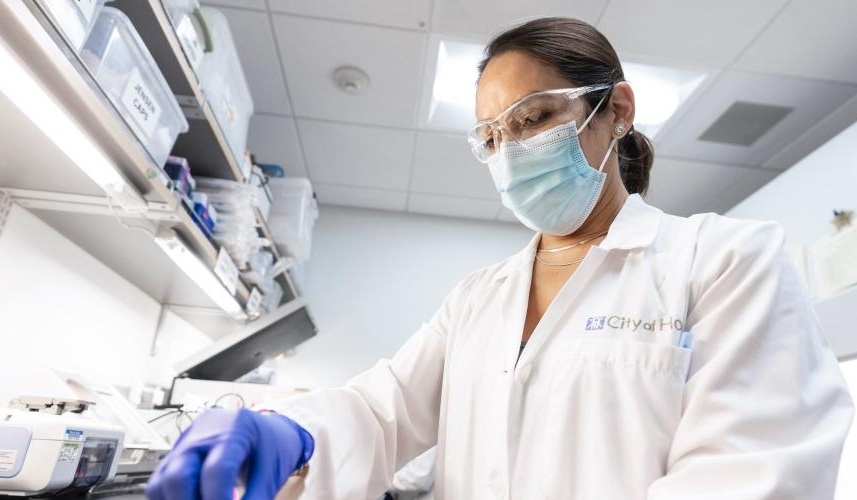
Machine Learning-Enabled Blood Test Predicts Immunotherapy Response in Lymphoma Patients
Chimeric antigen receptor (CAR) T-cell therapy has emerged as one of the most promising recent developments in the treatment of blood cancers. However, over half of non-Hodgkin lymphoma (NHL) patients... Read moreMicrobiology
view channel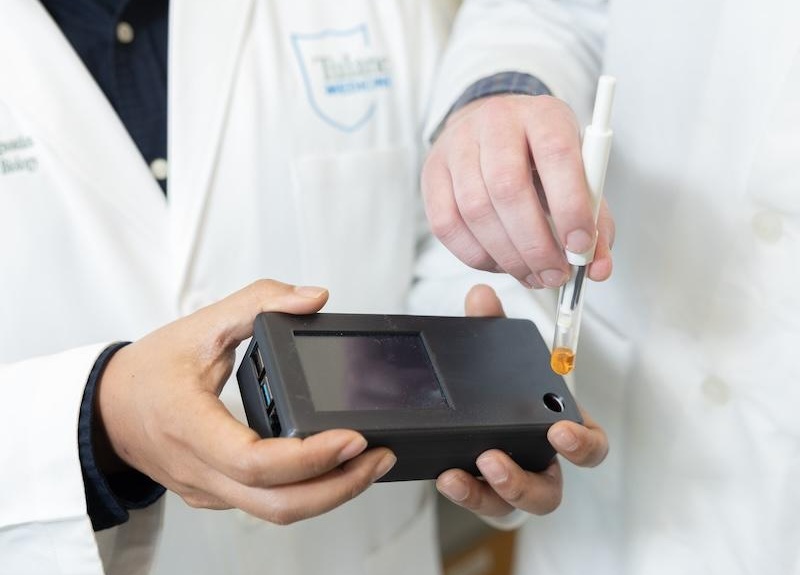
Handheld Device Deliver Low-Cost TB Results in Less Than One Hour
Tuberculosis (TB) remains the deadliest infectious disease globally, affecting an estimated 10 million people annually. In 2021, about 4.2 million TB cases went undiagnosed or unreported, mainly due to... Read more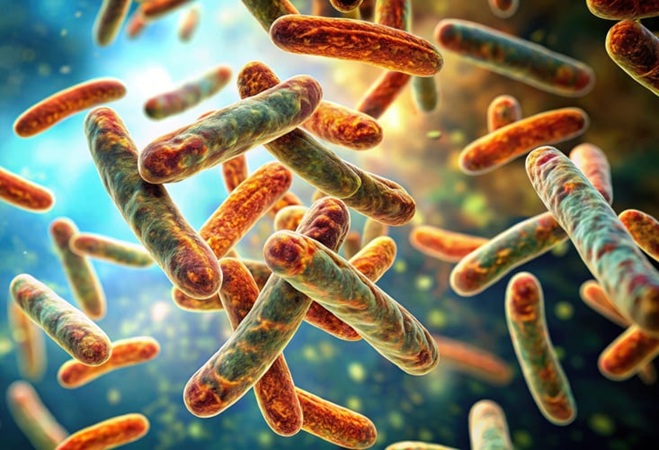
New AI-Based Method Improves Diagnosis of Drug-Resistant Infections
Drug-resistant infections, particularly those caused by deadly bacteria like tuberculosis and staphylococcus, are rapidly emerging as a global health emergency. These infections are more difficult to treat,... Read more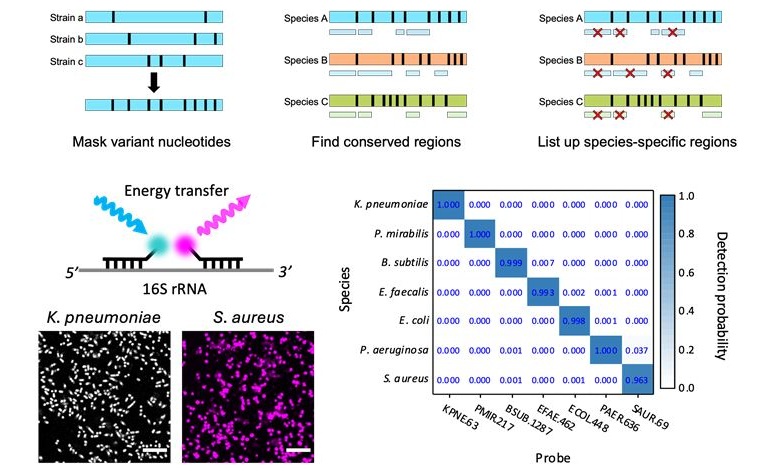
Breakthrough Diagnostic Technology Identifies Bacterial Infections with Almost 100% Accuracy within Three Hours
Rapid and precise identification of pathogenic microbes in patient samples is essential for the effective treatment of acute infectious diseases, such as sepsis. The fluorescence in situ hybridization... Read morePathology
view channel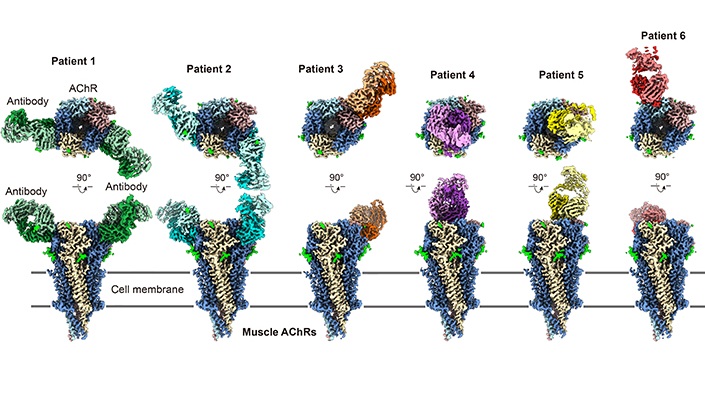
Advanced Imaging Reveals Mechanisms Causing Autoimmune Disease
Myasthenia gravis, an autoimmune disease, leads to muscle weakness that can affect a range of muscles, including those needed for basic actions like blinking, smiling, or moving. Researchers have long... Read more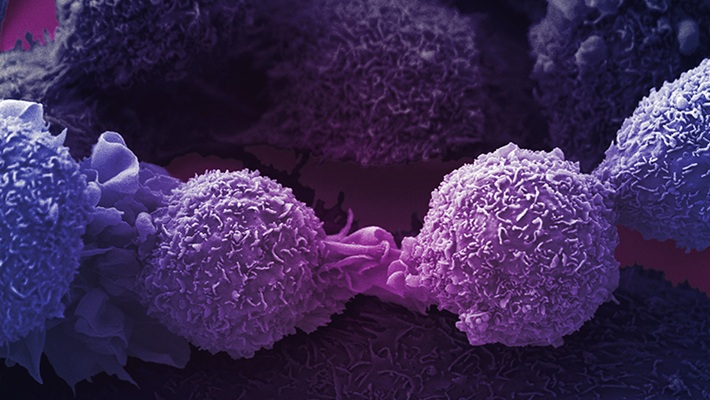
AI Model Effectively Predicts Patient Outcomes in Common Lung Cancer Type
Lung adenocarcinoma, the most common form of non-small cell lung cancer (NSCLC), typically adopts one of six distinct growth patterns, often combining multiple patterns within a single tumor.... Read moreTechnology
view channel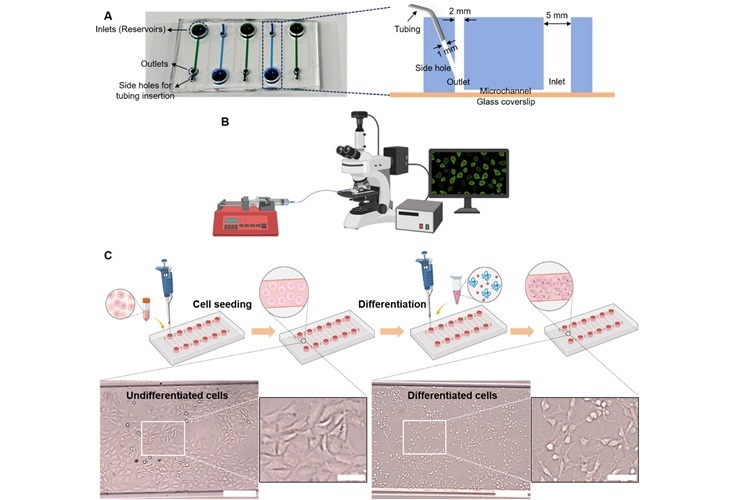
Pain-On-A-Chip Microfluidic Device Determines Types of Chronic Pain from Blood Samples
Chronic pain is a widespread condition that remains difficult to manage, and existing clinical methods for its treatment rely largely on self-reporting, which can be subjective and especially problematic... Read more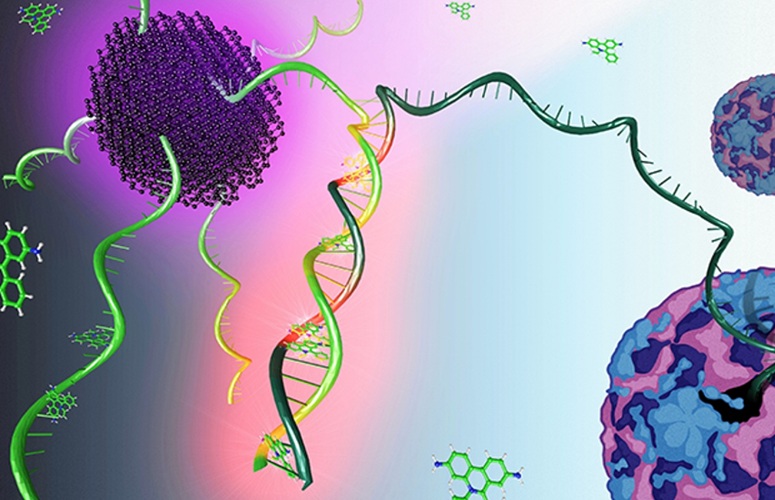
Innovative, Label-Free Ratiometric Fluorosensor Enables More Sensitive Viral RNA Detection
Viruses present a major global health risk, as demonstrated by recent pandemics, making early detection and identification essential for preventing new outbreaks. While traditional detection methods are... Read moreIndustry
view channel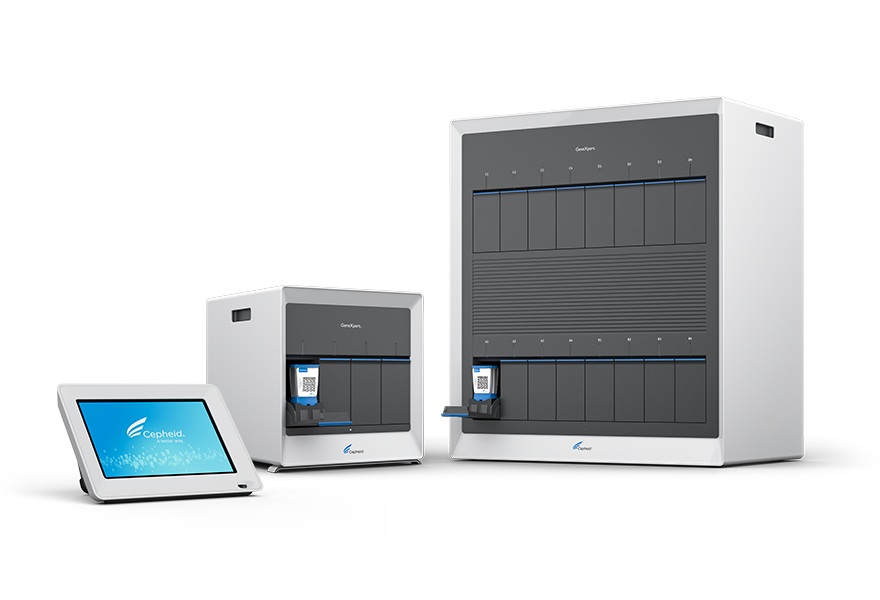
Cepheid and Oxford Nanopore Technologies Partner on Advancing Automated Sequencing-Based Solutions
Cepheid (Sunnyvale, CA, USA), a leading molecular diagnostics company, and Oxford Nanopore Technologies (Oxford, UK), the company behind a new generation of sequencing-based molecular analysis technologies,... Read more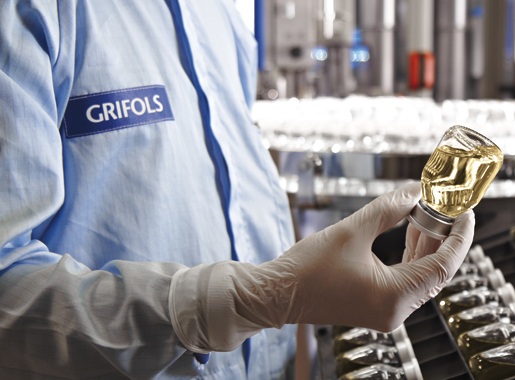
Grifols and Tecan’s IBL Collaborate on Advanced Biomarker Panels
Grifols (Barcelona, Spain), one of the world’s leading producers of plasma-derived medicines and innovative diagnostic solutions, is expanding its offer in clinical diagnostics through a strategic partnership... Read more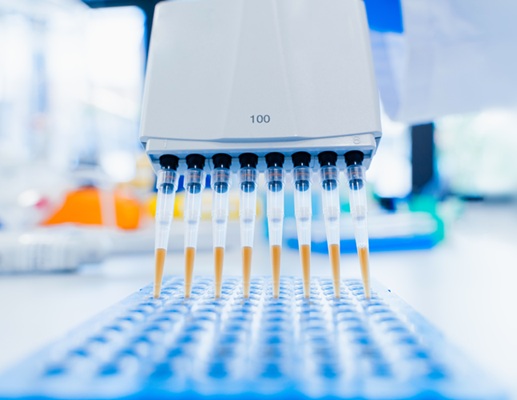