Gut Microbiome Data Helps Routine Screening of Cardiovascular Disease
By LabMedica International staff writers Posted on 22 Sep 2020 |
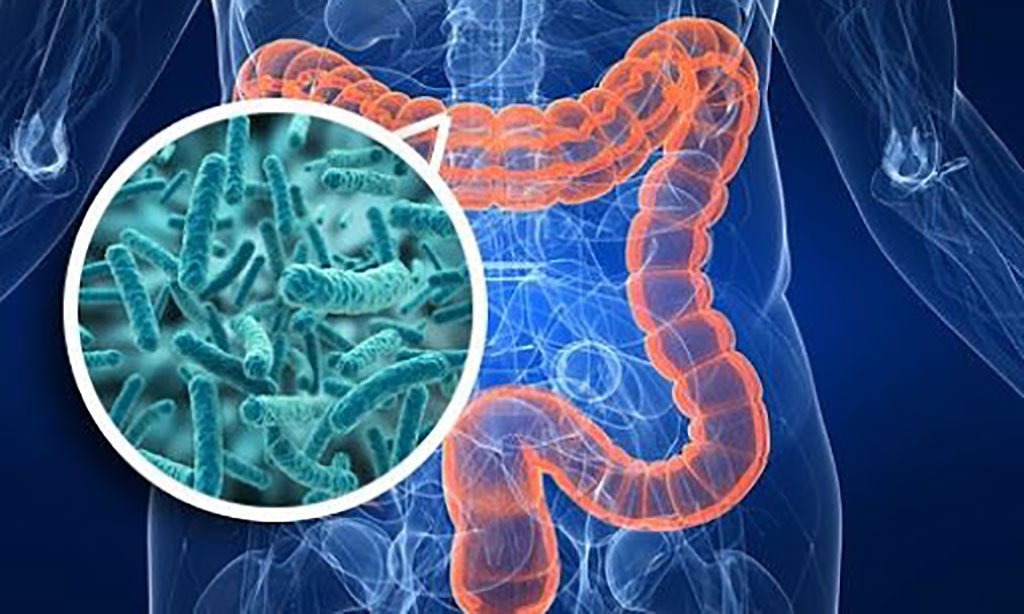
Image: Gut Microbiome Data Helps Routine Screening of Cardiovascular Disease (Photo courtesy of Nishant Mehta PhD).
Besides genetics and environmental factors, gut microbiota has emerged as a new factor influencing cardiovascular disease (CVD). Although cause-effect relationships are not clearly established, the reported associations between alterations in gut microbiota and CVD are prominent.
Recent studies have found a link between gut microbiota, the microorganisms in human digestive tracts, and, CVD which is the leading cause of mortality worldwide. Gut microbiota is highly variable between individuals, and differences in gut microbial compositions between people with and without CVD have been reported.
Scientists at the University of Toledo (Toledo, OH, USA) hypothesized that machine learning (ML) could be used for gut microbiome–based diagnostic screening of CVD. To test their hypothesis, fecal 16S ribosomal RNA sequencing data of 478 CVD and 473 non-CVD human subjects collected through the American Gut Project were analyzed using five supervised ML algorithms, including random forest, support vector machine, decision tree, elastic net, and neural networks.
The team identified 39 differential bacterial taxa between the CVD and non-CVD groups. ML modeling using these taxonomic features achieved a testing area under the receiver operating characteristic curve (0.0, perfect antidiscrimination; 0.5, random guessing; 1.0, perfect discrimination) of ≈0.58 (random forest and neural networks). Next, the ML models were trained with the top 500 high-variance features of operational taxonomic units, instead of bacterial taxa, and an improved testing area under the receiver operating characteristic curves of ≈0.65 (random forest) was achieved.
Further, by limiting the selection to only the top 25 highly contributing operational taxonomic unit features, the area under the receiver operating characteristic curves was further significantly enhanced to ≈0.70. Among the bacteria identified were Bacteroides, Subdoligranulum, Clostridium, Megasphaera, Eubacterium, Veillonella, Acidaminococcus and Listeria were more abundant in the CVD group. Faecalibacterium, Ruminococcus, Proteus, Lachnospira, Brevundimonas, Alistipes and Neisseria were more abundant in the non-CVD group.
Bina Joe, PhD, FAHA, Distinguished University Professor and Chairwoman of the department of Physiology and Pharmacology, said, “Despite the fact that gut microbiomes are highly variable among individuals, we were surprised by the promising level of accuracy obtained from these preliminary results, which indicate fecal microbiota composition could potentially serve as a convenient diagnostic screening method for CVD.”
The authors concluded that overall, the study was the first to identify dysbiosis of gut microbiota in CVD patients as a group and apply this knowledge to develop a gut microbiome–based ML approach for diagnostic screening of CVD. The study was published on September 10, 2020 in the journal Hypertension.
Related Links:
University of Toledo
Recent studies have found a link between gut microbiota, the microorganisms in human digestive tracts, and, CVD which is the leading cause of mortality worldwide. Gut microbiota is highly variable between individuals, and differences in gut microbial compositions between people with and without CVD have been reported.
Scientists at the University of Toledo (Toledo, OH, USA) hypothesized that machine learning (ML) could be used for gut microbiome–based diagnostic screening of CVD. To test their hypothesis, fecal 16S ribosomal RNA sequencing data of 478 CVD and 473 non-CVD human subjects collected through the American Gut Project were analyzed using five supervised ML algorithms, including random forest, support vector machine, decision tree, elastic net, and neural networks.
The team identified 39 differential bacterial taxa between the CVD and non-CVD groups. ML modeling using these taxonomic features achieved a testing area under the receiver operating characteristic curve (0.0, perfect antidiscrimination; 0.5, random guessing; 1.0, perfect discrimination) of ≈0.58 (random forest and neural networks). Next, the ML models were trained with the top 500 high-variance features of operational taxonomic units, instead of bacterial taxa, and an improved testing area under the receiver operating characteristic curves of ≈0.65 (random forest) was achieved.
Further, by limiting the selection to only the top 25 highly contributing operational taxonomic unit features, the area under the receiver operating characteristic curves was further significantly enhanced to ≈0.70. Among the bacteria identified were Bacteroides, Subdoligranulum, Clostridium, Megasphaera, Eubacterium, Veillonella, Acidaminococcus and Listeria were more abundant in the CVD group. Faecalibacterium, Ruminococcus, Proteus, Lachnospira, Brevundimonas, Alistipes and Neisseria were more abundant in the non-CVD group.
Bina Joe, PhD, FAHA, Distinguished University Professor and Chairwoman of the department of Physiology and Pharmacology, said, “Despite the fact that gut microbiomes are highly variable among individuals, we were surprised by the promising level of accuracy obtained from these preliminary results, which indicate fecal microbiota composition could potentially serve as a convenient diagnostic screening method for CVD.”
The authors concluded that overall, the study was the first to identify dysbiosis of gut microbiota in CVD patients as a group and apply this knowledge to develop a gut microbiome–based ML approach for diagnostic screening of CVD. The study was published on September 10, 2020 in the journal Hypertension.
Related Links:
University of Toledo
Latest Microbiology News
- Handheld Device Delivers Low-Cost TB Results in Less Than One Hour
- New AI-Based Method Improves Diagnosis of Drug-Resistant Infections
- Breakthrough Diagnostic Technology Identifies Bacterial Infections with Almost 100% Accuracy within Three Hours
- Innovative ID/AST System to Help Diagnose Infectious Diseases and Combat AMR
- Gastrointestinal Panel Delivers Rapid Detection of Five Common Bacterial Pathogens for Outpatient Use
- Rapid PCR Testing in ICU Improves Antibiotic Stewardship
- Unique Genetic Signature Predicts Drug Resistance in Bacteria
- Unique Barcoding System Tracks Pneumonia-Causing Bacteria as They Infect Blood Stream
- Rapid Sepsis Diagnostic Test Demonstrates Improved Patient Care and Cost Savings in Hospital Application
- Rapid Diagnostic System to Detect Neonatal Sepsis Within Hours
- Novel Test to Diagnose Bacterial Pneumonia Directly from Whole Blood
- Interferon-γ Release Assay Effective in Patients with COPD Complicated with Pulmonary Tuberculosis
- New Point of Care Tests to Help Reduce Overuse of Antibiotics
- 30-Minute Sepsis Test Differentiates Bacterial Infections, Viral Infections, and Noninfectious Disease
- CRISPR-TB Blood Test to Enable Early Disease Diagnosis and Public Screening
- Syndromic Panel Provides Fast Answers for Outpatient Diagnosis of Gastrointestinal Conditions
Channels
Clinical Chemistry
view channel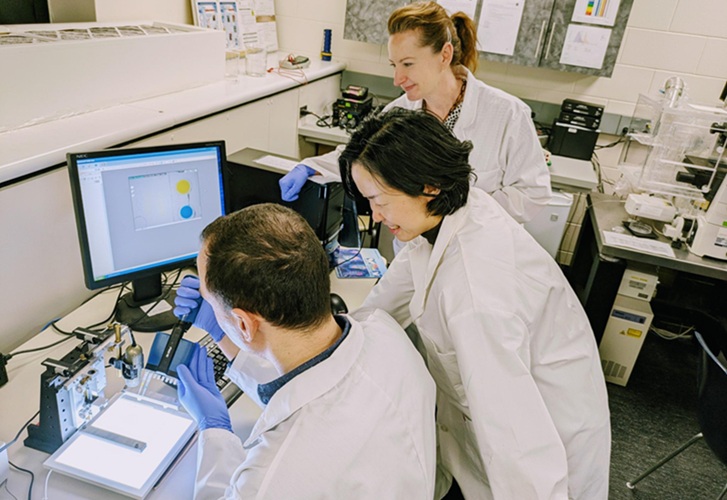
Low-Cost Portable Screening Test to Transform Kidney Disease Detection
Millions of individuals suffer from kidney disease, which often remains undiagnosed until it has reached a critical stage. This silent epidemic not only diminishes the quality of life for those affected... Read more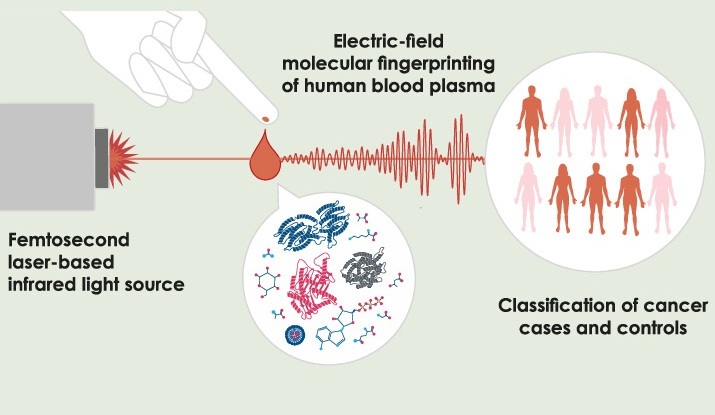
New Method Uses Pulsed Infrared Light to Find Cancer's 'Fingerprints' In Blood Plasma
Cancer diagnoses have traditionally relied on invasive or time-consuming procedures like tissue biopsies. Now, new research published in ACS Central Science introduces a method that utilizes pulsed infrared... Read moreMolecular Diagnostics
view channel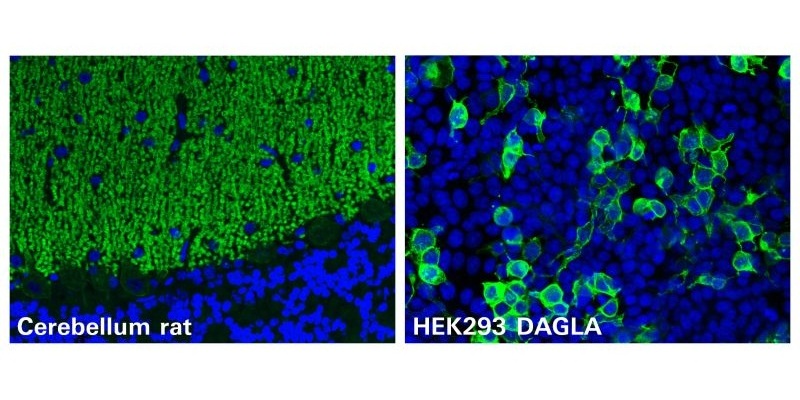
Novel Autoantibody Against DAGLA Discovered in Cerebellitis
Autoimmune cerebellar ataxias are strongly disabling disorders characterized by an impaired ability to coordinate muscle movement. Cerebellar autoantibodies serve as useful biomarkers to support rapid... Read more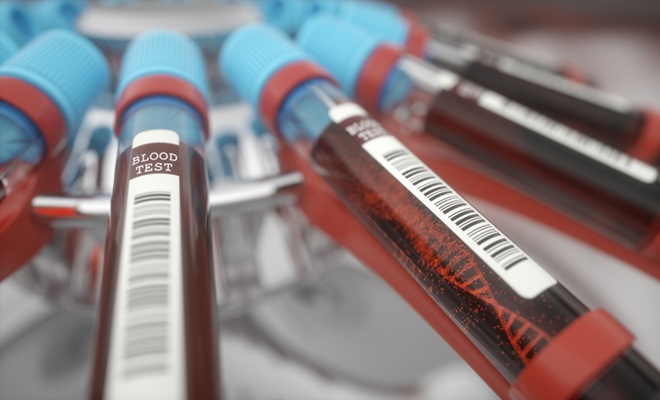
Gene-Based Blood Test Accurately Predicts Tumor Recurrence of Advanced Skin Cancer
Melanoma, an aggressive form of skin cancer, becomes extremely difficult to treat once it spreads to other parts of the body. For patients with metastatic melanoma tumors that cannot be surgically removed... Read moreHematology
view channel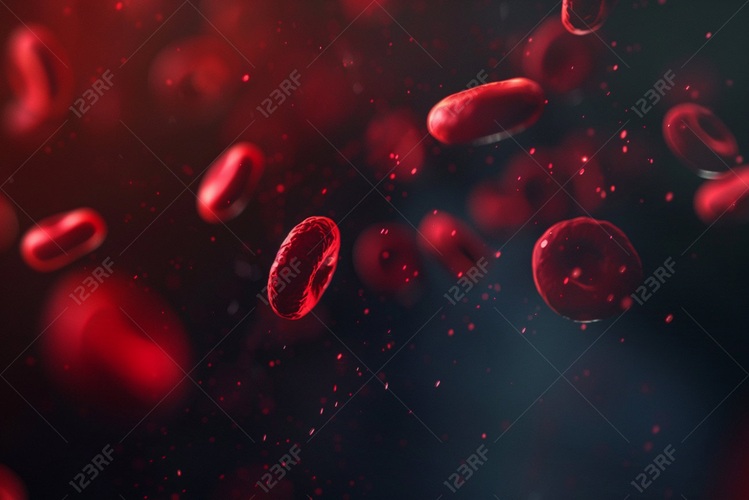
New Scoring System Predicts Risk of Developing Cancer from Common Blood Disorder
Clonal cytopenia of undetermined significance (CCUS) is a blood disorder commonly found in older adults, characterized by mutations in blood cells and a low blood count, but without any obvious cause or... Read more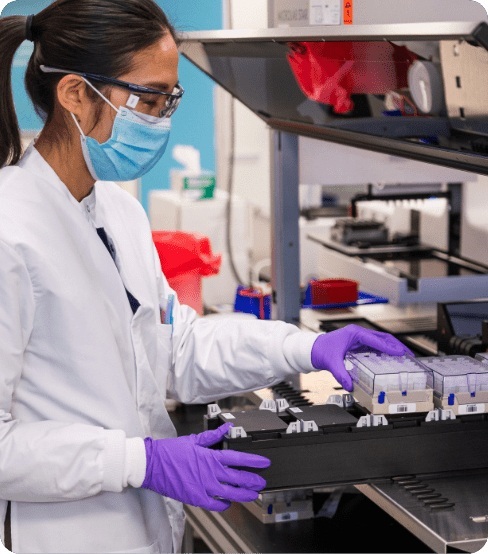
Non-Invasive Prenatal Test for Fetal RhD Status Demonstrates 100% Accuracy
In the United States, approximately 15% of pregnant individuals are RhD-negative. However, in about 40% of these cases, the fetus is also RhD-negative, making the administration of RhoGAM unnecessary.... Read moreImmunology
view channel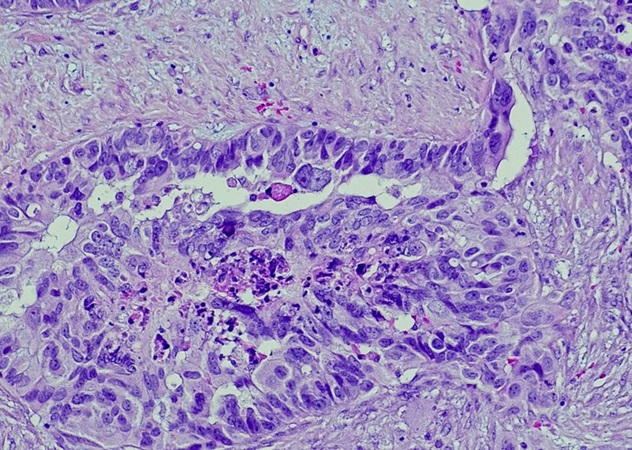
Stem Cell Test Predicts Treatment Outcome for Patients with Platinum-Resistant Ovarian Cancer
Epithelial ovarian cancer frequently responds to chemotherapy initially, but eventually, the tumor develops resistance to the therapy, leading to regrowth. This resistance is partially due to the activation... Read more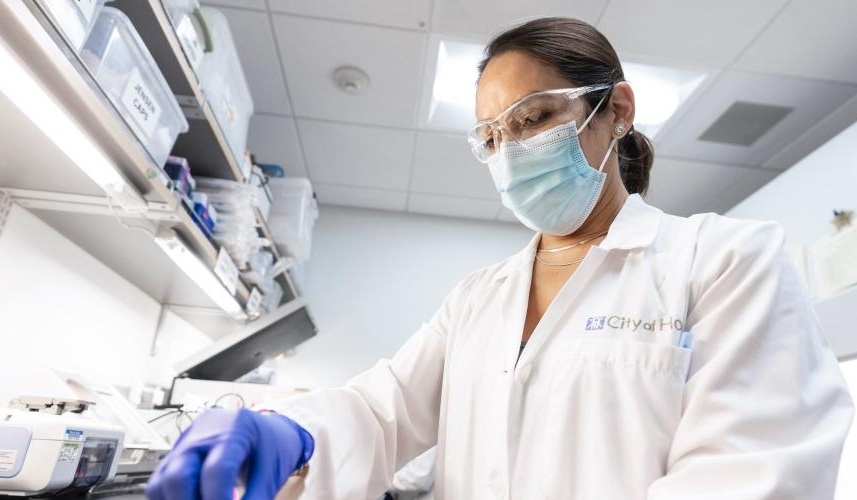
Machine Learning-Enabled Blood Test Predicts Immunotherapy Response in Lymphoma Patients
Chimeric antigen receptor (CAR) T-cell therapy has emerged as one of the most promising recent developments in the treatment of blood cancers. However, over half of non-Hodgkin lymphoma (NHL) patients... Read morePathology
view channel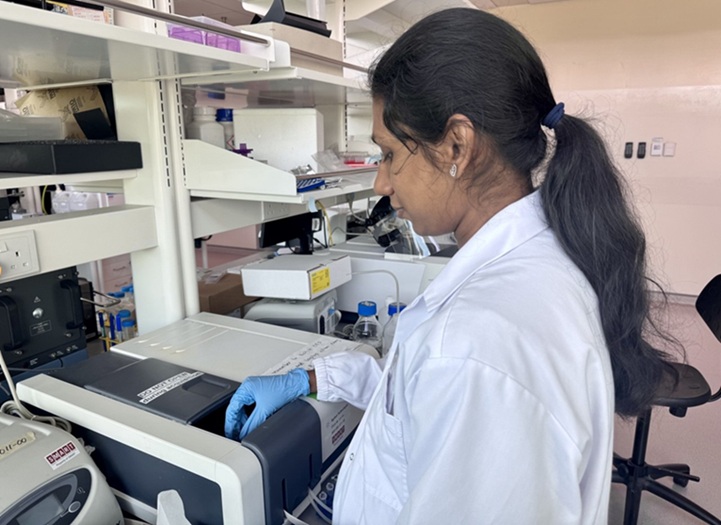
Novel UV and Machine Learning-Aided Method Detects Microbial Contamination in Cell Cultures
Cell therapy holds great potential in treating diseases such as cancers, inflammatory conditions, and chronic degenerative disorders by manipulating or replacing cells to restore function or combat disease.... Read more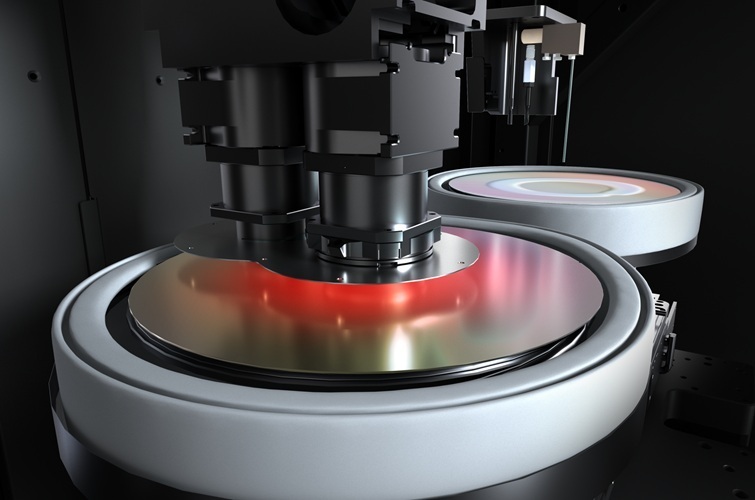
New Error-Corrected Method to Help Detect Cancer from Blood Samples Alone
"Liquid biopsy" technology, which relies on blood tests for early cancer detection and monitoring cancer burden in patients, has the potential to transform cancer care. However, detecting the mutational... Read more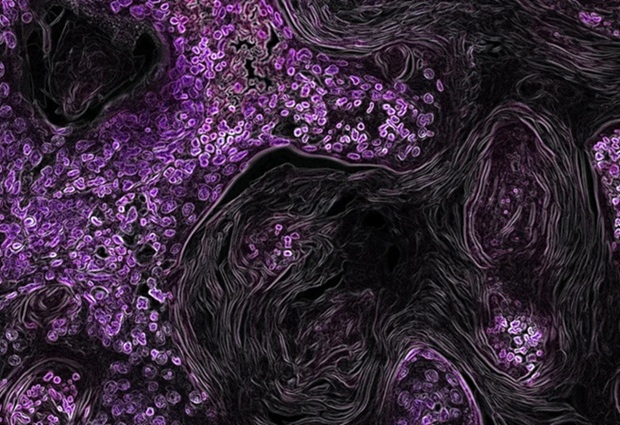
"Metal Detector" Algorithm Hunts Down Vulnerable Tumors
Scientists have developed an algorithm capable of functioning as a "metal detector" to identify vulnerable tumors, marking a significant advancement in personalized cancer treatment. This breakthrough... Read more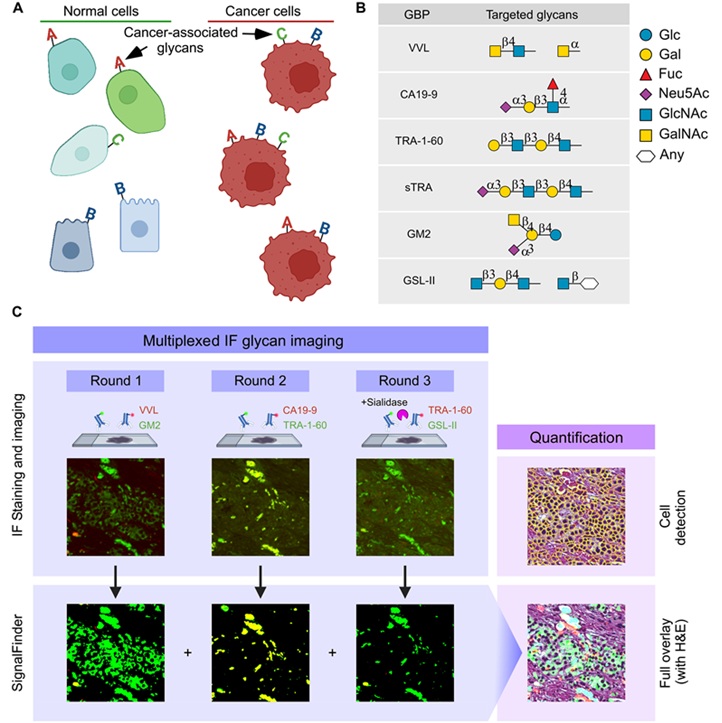
Novel Technique Uses ‘Sugar’ Signatures to Identify and Classify Pancreatic Cancer Cell Subtypes
Pancreatic cancer is often asymptomatic in its early stages, making it difficult to detect until it has progressed. Consequently, only 15% of pancreatic cancers are diagnosed early enough to allow for... Read moreTechnology
view channel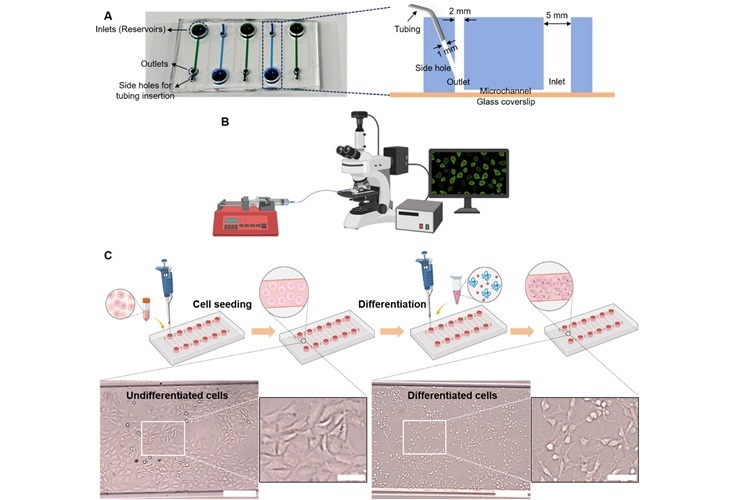
Pain-On-A-Chip Microfluidic Device Determines Types of Chronic Pain from Blood Samples
Chronic pain is a widespread condition that remains difficult to manage, and existing clinical methods for its treatment rely largely on self-reporting, which can be subjective and especially problematic... Read more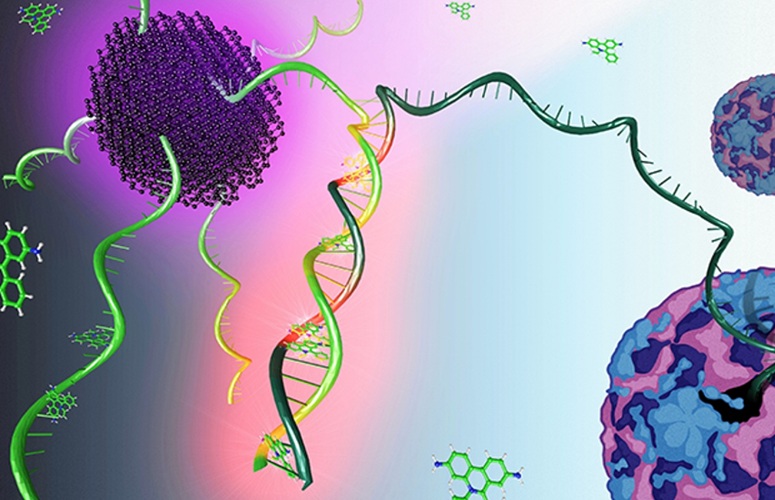
Innovative, Label-Free Ratiometric Fluorosensor Enables More Sensitive Viral RNA Detection
Viruses present a major global health risk, as demonstrated by recent pandemics, making early detection and identification essential for preventing new outbreaks. While traditional detection methods are... Read moreIndustry
view channel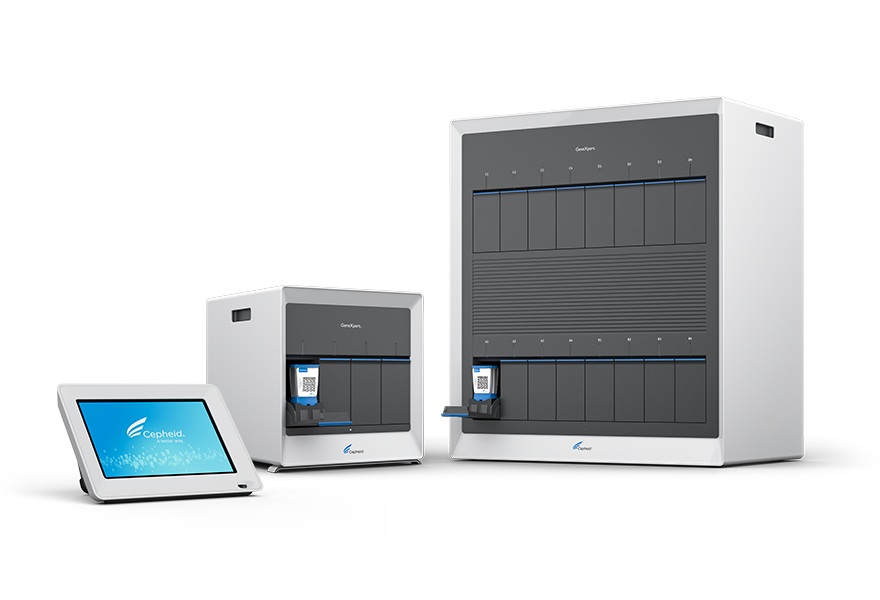
Cepheid and Oxford Nanopore Technologies Partner on Advancing Automated Sequencing-Based Solutions
Cepheid (Sunnyvale, CA, USA), a leading molecular diagnostics company, and Oxford Nanopore Technologies (Oxford, UK), the company behind a new generation of sequencing-based molecular analysis technologies,... Read more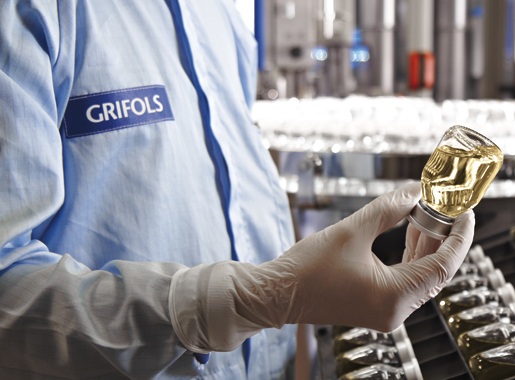
Grifols and Tecan’s IBL Collaborate on Advanced Biomarker Panels
Grifols (Barcelona, Spain), one of the world’s leading producers of plasma-derived medicines and innovative diagnostic solutions, is expanding its offer in clinical diagnostics through a strategic partnership... Read more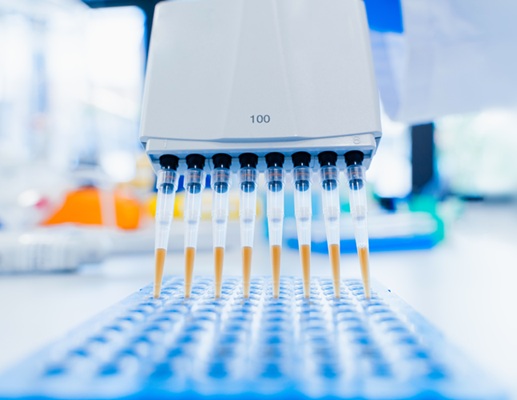