Prostate Cancer Identified By Profiling Immune Cell Subsets
By LabMedica International staff writers Posted on 19 Aug 2020 |
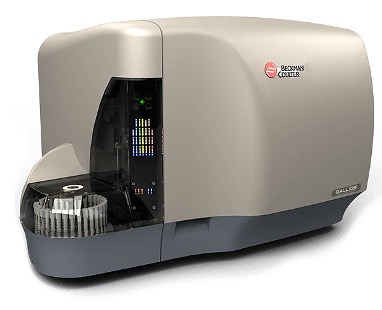
The Gallios 10-color/3-laser flow cytometer (Photo courtesy of Beckman Coulter).
With an estimated 1.8 million new cases in 2018 alone, prostate cancer is the fourth most common cancer in the world. Diagnosing the disease early increases the chances of survival, but this cancer remains difficult to detect.
The best diagnostic test currently available measures the blood level of a protein called the prostate-specific antigen (PSA for short). Heightened amounts of PSA may mean that the patient has cancer, but 15% of individuals with prostate cancer have normal levels of the protein.
A team of scientists at Nottingham Trent University (Nottingham, UK) and their colleagues obtained peripheral blood samples from individuals suspected of having prostate cancer that attended the Urology Clinic at Leicester General Hospital (Leicester, UK) between 24th October 2012 and 15th August 2014. Peripheral blood (60 mL) was collected from all patients using standard clinical procedures, and the peripheral blood mononuclear cell (PBMC) fraction was harvested. The PBMCs were processed and incubated with a monoclonal antibody (mAb) panel. Data on viable cells were acquired within 1 hour using a Gallios 10-color/3-laser flow cytometer (Beckman Coulter, Indianapolis, IN, USA).
The team collected and examined the natural killer cells of 72 participants with slightly elevated PSA levels and no other symptoms. Amongst these, 31 individuals had prostate cancer and 41 were healthy. These biological data were then used to produce computer models that could detect the presence of the disease, as well as assess its severity. The algorithms were developed using machine learning, where previous patient information is used to make prediction on new data.
Statistical and computational methods identified a panel of eight phenotypic features (CD56dimCD16high, CD56+DNAM−1−, CD56+LAIR−1+, CD56+LAIR−1−, CD56brightCD8+, CD56+NKp30+, CD56+NKp30−, CD56+NKp46+) that, when incorporated into an Ensemble machine learning prediction model, distinguished between the presence of benign prostate disease and prostate cancer. The machine learning model was then adapted to predict the D’Amico Risk Classification using data from 54 patients with prostate cancer and was shown to accurately differentiate between the presence of low-/intermediate-risk disease and high-risk disease without the need for additional clinical data.
The authors concluded that their study resulted in a new detection tool which was 12.5% more accurate than the PSA test in detecting prostate cancer; and in a detection tool that was 99% accurate in predicting the risk of the disease (in terms of clinical significance) in individuals with prostate cancer. This simple blood test has the potential to transform prostate cancer diagnostics. The study was published on July 28, 2020 in the journal eLife.
Related Links:
Nottingham Trent University
Leicester General Hospital
Beckman Coulter
The best diagnostic test currently available measures the blood level of a protein called the prostate-specific antigen (PSA for short). Heightened amounts of PSA may mean that the patient has cancer, but 15% of individuals with prostate cancer have normal levels of the protein.
A team of scientists at Nottingham Trent University (Nottingham, UK) and their colleagues obtained peripheral blood samples from individuals suspected of having prostate cancer that attended the Urology Clinic at Leicester General Hospital (Leicester, UK) between 24th October 2012 and 15th August 2014. Peripheral blood (60 mL) was collected from all patients using standard clinical procedures, and the peripheral blood mononuclear cell (PBMC) fraction was harvested. The PBMCs were processed and incubated with a monoclonal antibody (mAb) panel. Data on viable cells were acquired within 1 hour using a Gallios 10-color/3-laser flow cytometer (Beckman Coulter, Indianapolis, IN, USA).
The team collected and examined the natural killer cells of 72 participants with slightly elevated PSA levels and no other symptoms. Amongst these, 31 individuals had prostate cancer and 41 were healthy. These biological data were then used to produce computer models that could detect the presence of the disease, as well as assess its severity. The algorithms were developed using machine learning, where previous patient information is used to make prediction on new data.
Statistical and computational methods identified a panel of eight phenotypic features (CD56dimCD16high, CD56+DNAM−1−, CD56+LAIR−1+, CD56+LAIR−1−, CD56brightCD8+, CD56+NKp30+, CD56+NKp30−, CD56+NKp46+) that, when incorporated into an Ensemble machine learning prediction model, distinguished between the presence of benign prostate disease and prostate cancer. The machine learning model was then adapted to predict the D’Amico Risk Classification using data from 54 patients with prostate cancer and was shown to accurately differentiate between the presence of low-/intermediate-risk disease and high-risk disease without the need for additional clinical data.
The authors concluded that their study resulted in a new detection tool which was 12.5% more accurate than the PSA test in detecting prostate cancer; and in a detection tool that was 99% accurate in predicting the risk of the disease (in terms of clinical significance) in individuals with prostate cancer. This simple blood test has the potential to transform prostate cancer diagnostics. The study was published on July 28, 2020 in the journal eLife.
Related Links:
Nottingham Trent University
Leicester General Hospital
Beckman Coulter
Latest Immunology News
- Post-Treatment Blood Test Could Inform Future Cancer Therapy Decisions
- Cerebrospinal Fluid Test Predicts Dangerous Side Effect of Cancer Treatment
- New Test Measures Preterm Infant Immunity Using Only Two Drops of Blood
- Simple Blood Test Could Help Choose Better Treatments for Patients with Recurrent Endometrial Cancer
- Novel Analytical Method Tracks Progression of Autoimmune Diseases
- 3D Bioprinted Gastric Cancer Model Uses Patient-Derived Tissue Fragments to Predict Drug Response
- Blood Test for Fungal Infections Could End Invasive Tissue Biopsies
- Cutting-Edge Microscopy Technology Enables Tailored Rheumatology Therapies
- New Discovery in Blood Immune Cells Paves Way for Parkinson's Disease Diagnostic Test
- AI Tool Uses Routine Blood Tests to Predict Immunotherapy Response for Various Cancers
- Blood Test Can Predict How Long Vaccine Immunity Will Last
- Microfluidic Chip-Based Device to Measure Viral Immunity
- Simple Blood Test Could Detect Drug Resistance in Ovarian Cancer Patients
- Advanced Imaging Method Maps Immune Cell Connections to Predict Cancer Patients Survival
Channels
Clinical Chemistry
view channel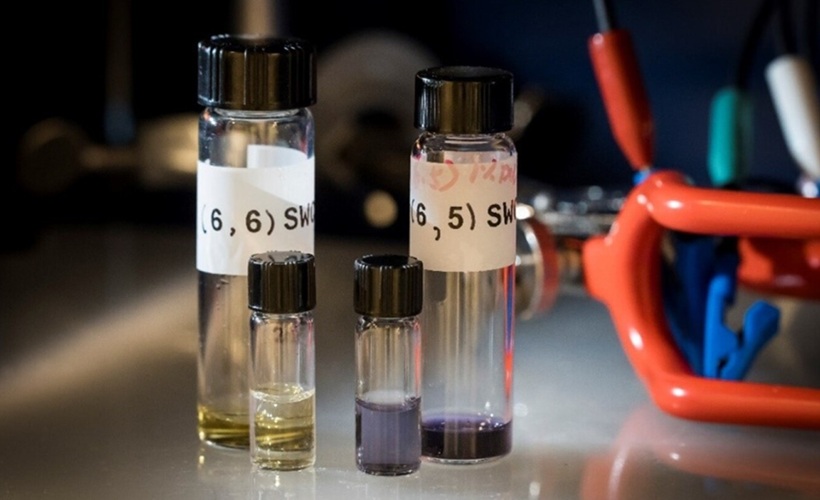
Carbon Nanotubes Help Build Highly Accurate Sensors for Continuous Health Monitoring
Current sensors can measure various health indicators, such as blood glucose levels, in the body. However, there is a need to develop more accurate and sensitive sensor materials that can detect lower... Read more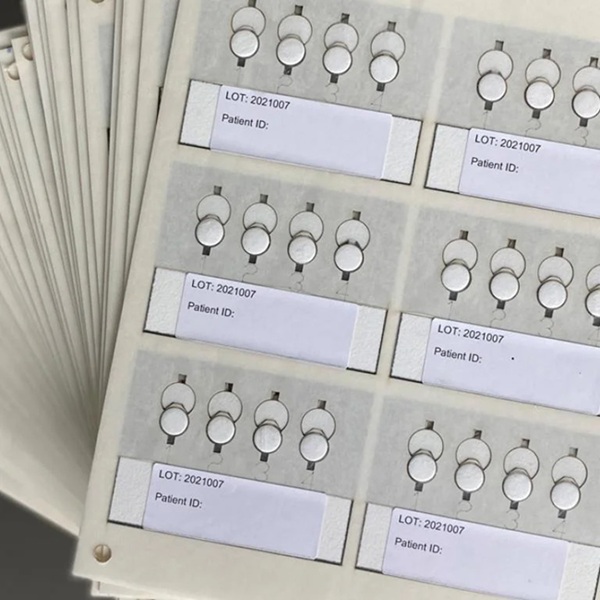
Paper-Based Device Boosts HIV Test Accuracy from Dried Blood Samples
In regions where access to clinics for routine blood tests presents financial and logistical obstacles, HIV patients are increasingly able to collect and send a drop of blood using paper-based devices... Read moreMolecular Diagnostics
view channel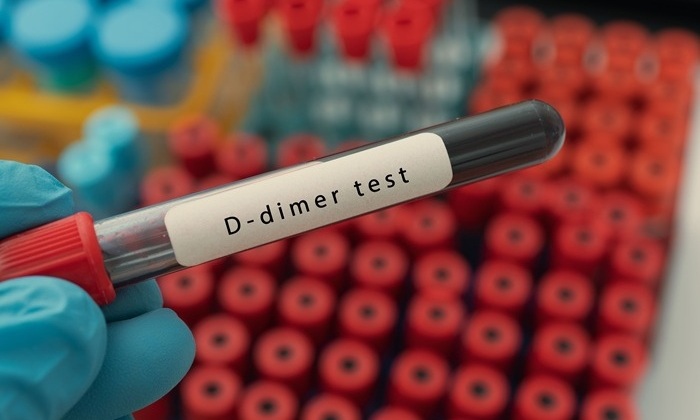
D-Dimer Testing Can Identify Patients at Higher Risk of Pulmonary Embolism
Pulmonary embolism (PE) is a commonly suspected condition in emergency departments (EDs) and can be life-threatening if not diagnosed correctly. Achieving an accurate diagnosis is vital for providing effective... Read more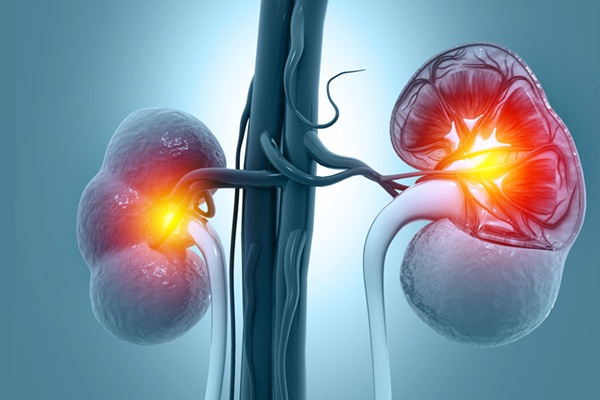
New Biomarkers to Improve Early Detection and Monitoring of Kidney Injury
Drug-induced kidney injury, also known as nephrotoxicity, is a prevalent issue in clinical practice, occurring when specific medications at certain doses cause damage to the kidneys. Nephrotoxicity can... Read moreHematology
view channel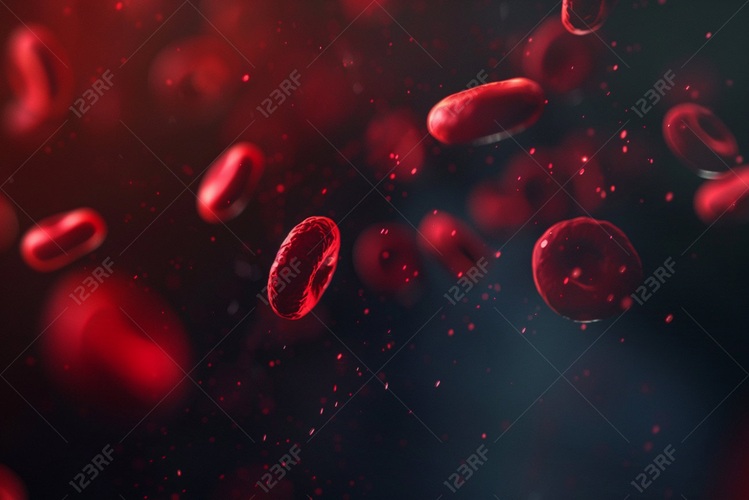
New Scoring System Predicts Risk of Developing Cancer from Common Blood Disorder
Clonal cytopenia of undetermined significance (CCUS) is a blood disorder commonly found in older adults, characterized by mutations in blood cells and a low blood count, but without any obvious cause or... Read more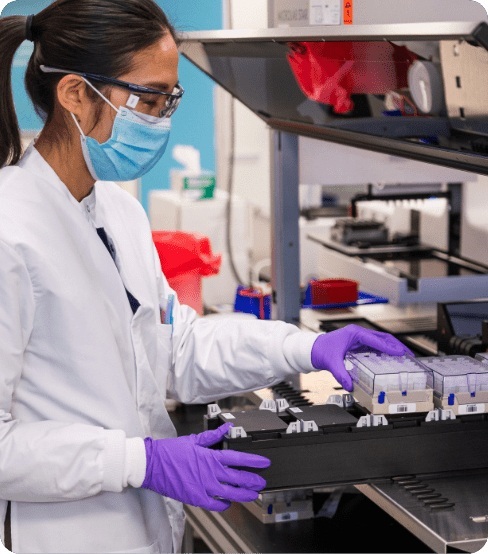
Non-Invasive Prenatal Test for Fetal RhD Status Demonstrates 100% Accuracy
In the United States, approximately 15% of pregnant individuals are RhD-negative. However, in about 40% of these cases, the fetus is also RhD-negative, making the administration of RhoGAM unnecessary.... Read moreMicrobiology
view channel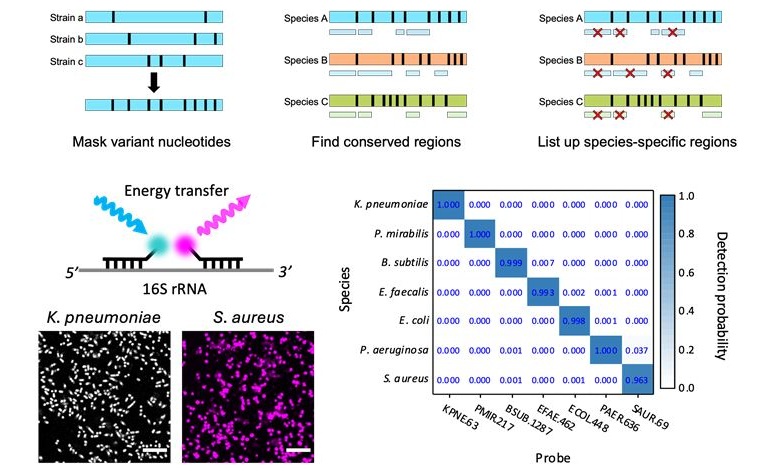
Breakthrough Diagnostic Technology Identifies Bacterial Infections with Almost 100% Accuracy within Three Hours
Rapid and precise identification of pathogenic microbes in patient samples is essential for the effective treatment of acute infectious diseases, such as sepsis. The fluorescence in situ hybridization... Read moreInnovative ID/AST System to Help Diagnose Infectious Diseases and Combat AMR
Each year, 11 million people across the world die of sepsis out of which 1.3 million deaths are due to antibiotic-resistant bacteria. The burden of antimicrobial resistance (AMR) continues to weigh heavily,... Read more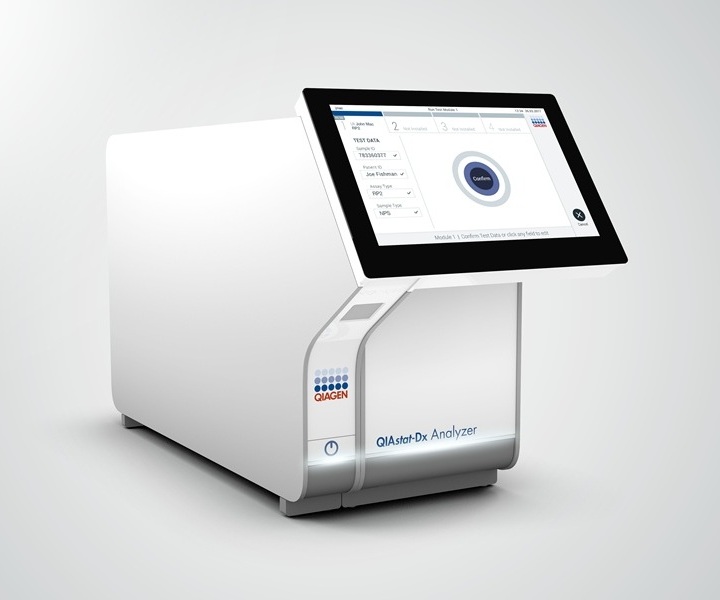
Gastrointestinal Panel Delivers Rapid Detection of Five Common Bacterial Pathogens for Outpatient Use
Acute infectious gastroenteritis results in approximately 179 million cases each year in the United States, leading to a significant number of outpatient visits and hospitalizations. To address this, a... Read morePathology
view channel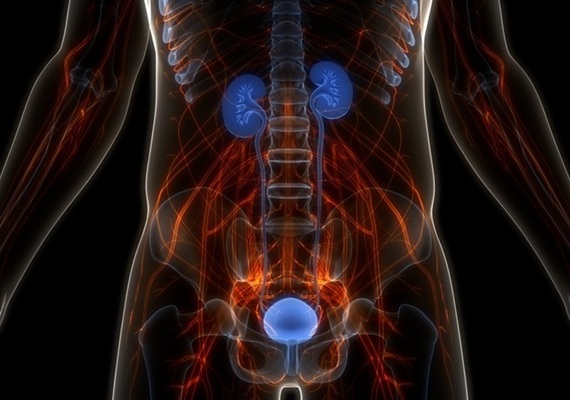
AI Model Predicts Patient Response to Bladder Cancer Treatment
Each year in the United States, around 81,000 new cases of bladder cancer are diagnosed, leading to approximately 17,000 deaths annually. Muscle-invasive bladder cancer (MIBC) is a severe form of bladder... Read more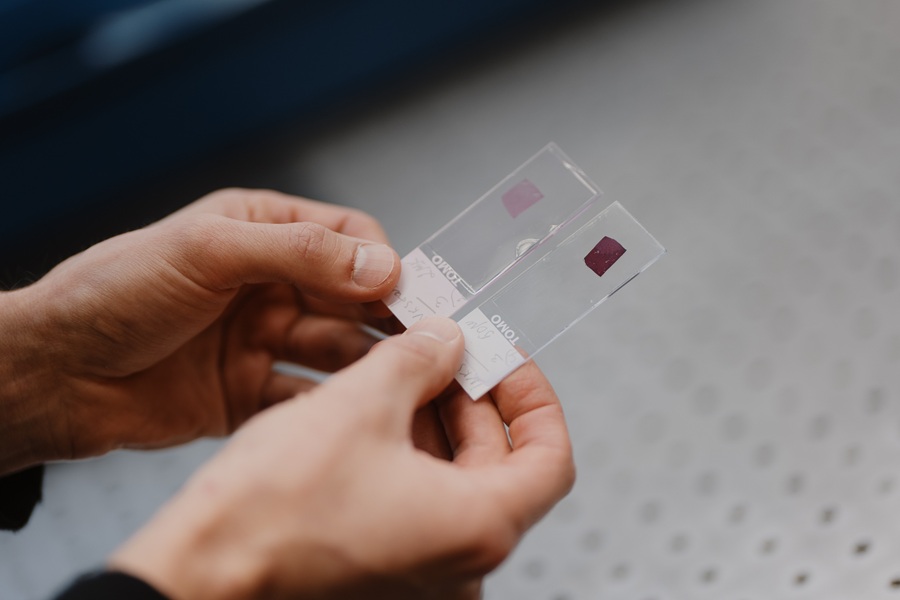
New Laser-Based Method to Accelerate Cancer Diagnosis
Researchers have developed a method to improve cancer diagnostics and other diseases. Collagen, a key structural protein, plays various roles in cell activity. A novel multidisciplinary study published... Read more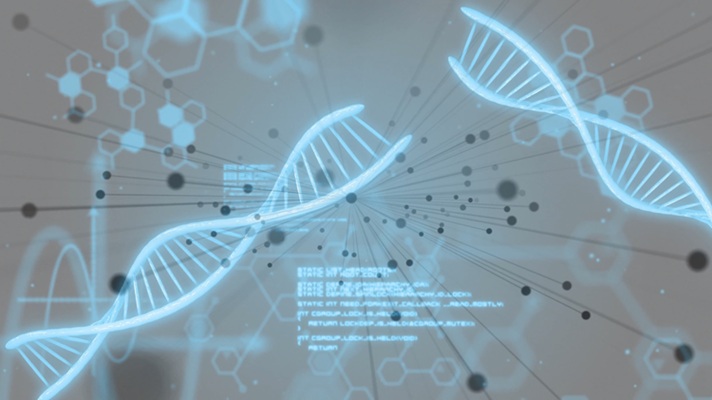
New AI Model Predicts Gene Variants’ Effects on Specific Diseases
In recent years, artificial intelligence (AI) has greatly enhanced our ability to identify a vast number of genetic variants in increasingly larger populations. However, up to half of these variants are... Read more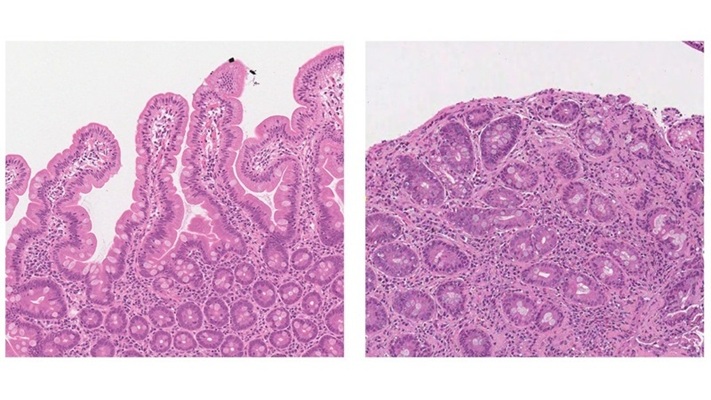
Powerful AI Tool Diagnoses Coeliac Disease from Biopsy Images with Over 97% Accuracy
Coeliac disease is an autoimmune disorder triggered by the consumption of gluten, causing symptoms such as stomach cramps, diarrhea, skin rashes, weight loss, fatigue, and anemia. Due to the wide variation... Read moreTechnology
view channel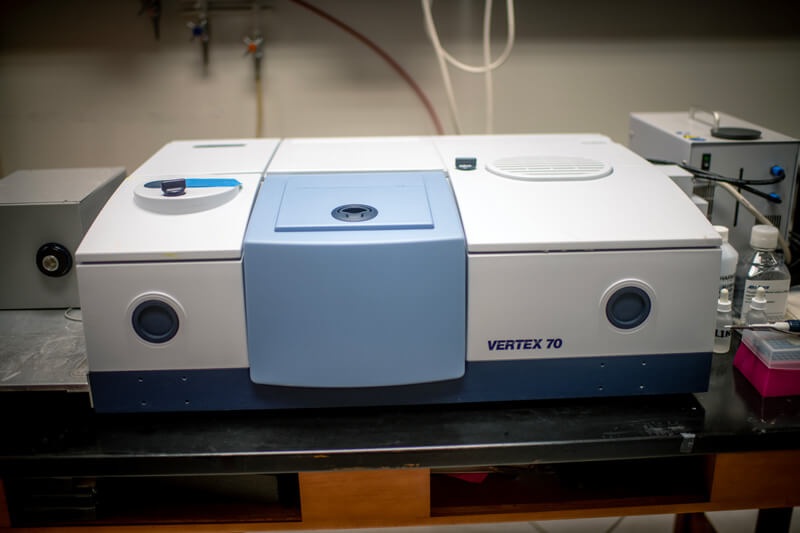
Smartphones Could Diagnose Diseases Using Infrared Scans
Rapid advancements in technology may soon make it possible for individuals to bypass invasive medical procedures by simply uploading a screenshot of their lab results from their phone directly to their doctor.... Read more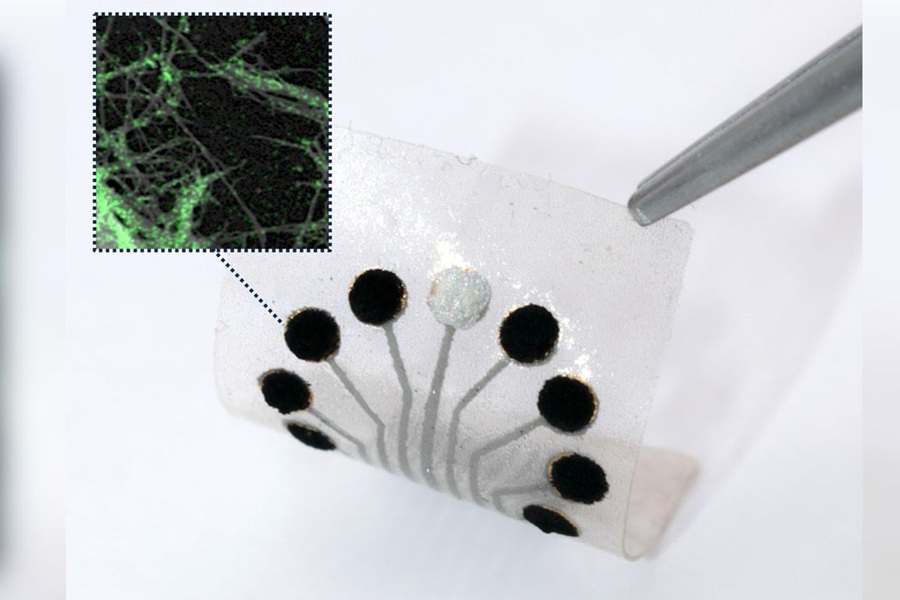
Novel Sensor Technology to Enable Early Diagnoses of Metabolic and Cardiovascular Disorders
Metabolites are critical compounds that fuel life's essential functions, playing a key role in producing energy, regulating cellular activities, and maintaining the balance of bodily systems.... Read more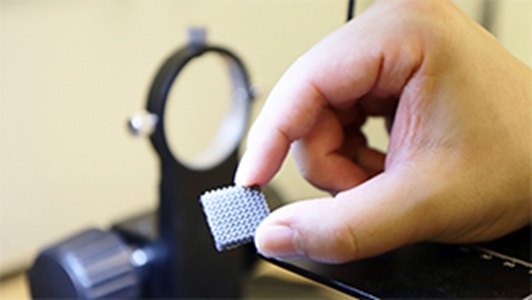
3D Printing Breakthrough Enables Large Scale Development of Tiny Microfluidic Devices
Microfluidic devices are diagnostic systems capable of analyzing small volumes of materials with precision and speed. These devices are used in a variety of applications, including cancer cell analysis,... Read moreIndustry
view channel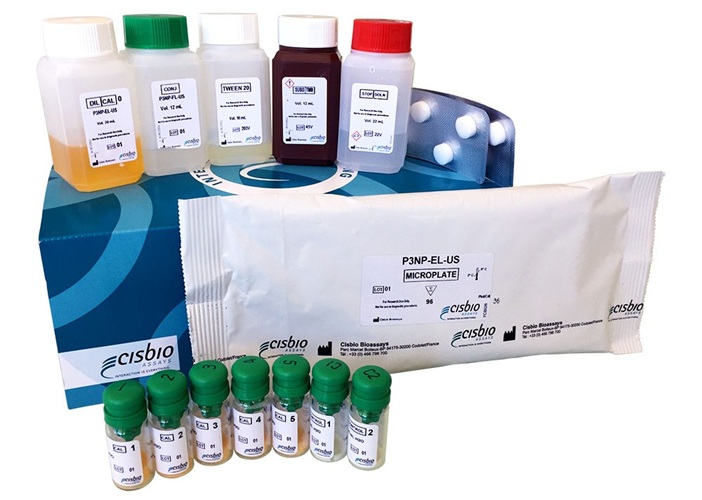
Tecan Acquires ELISA Immunoassay Assets from Revvity's Cisbio Bioassays
Tecan Group (Männedorf, Switzerland) has entered into an agreement to acquire certain assets relating to key ELISA immunoassay products from Cisbio Bioassays SAS (Codolet, France), a subsidiary of the... Read more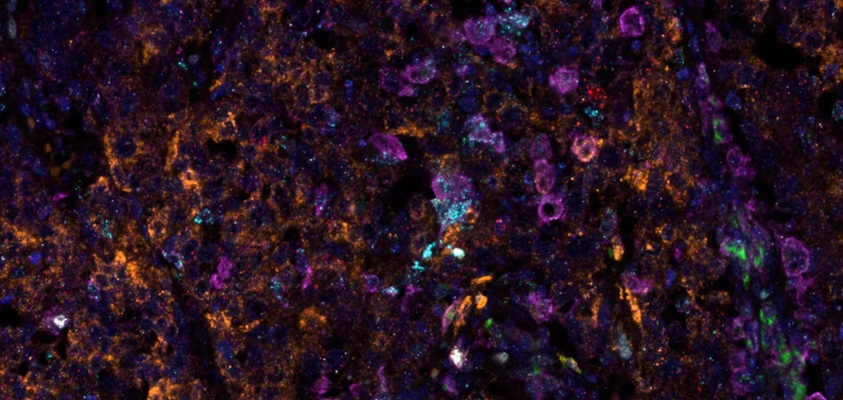