Automated Microscopy Compared for Routine Malaria Diagnosis
By LabMedica International staff writers Posted on 10 Oct 2018 |
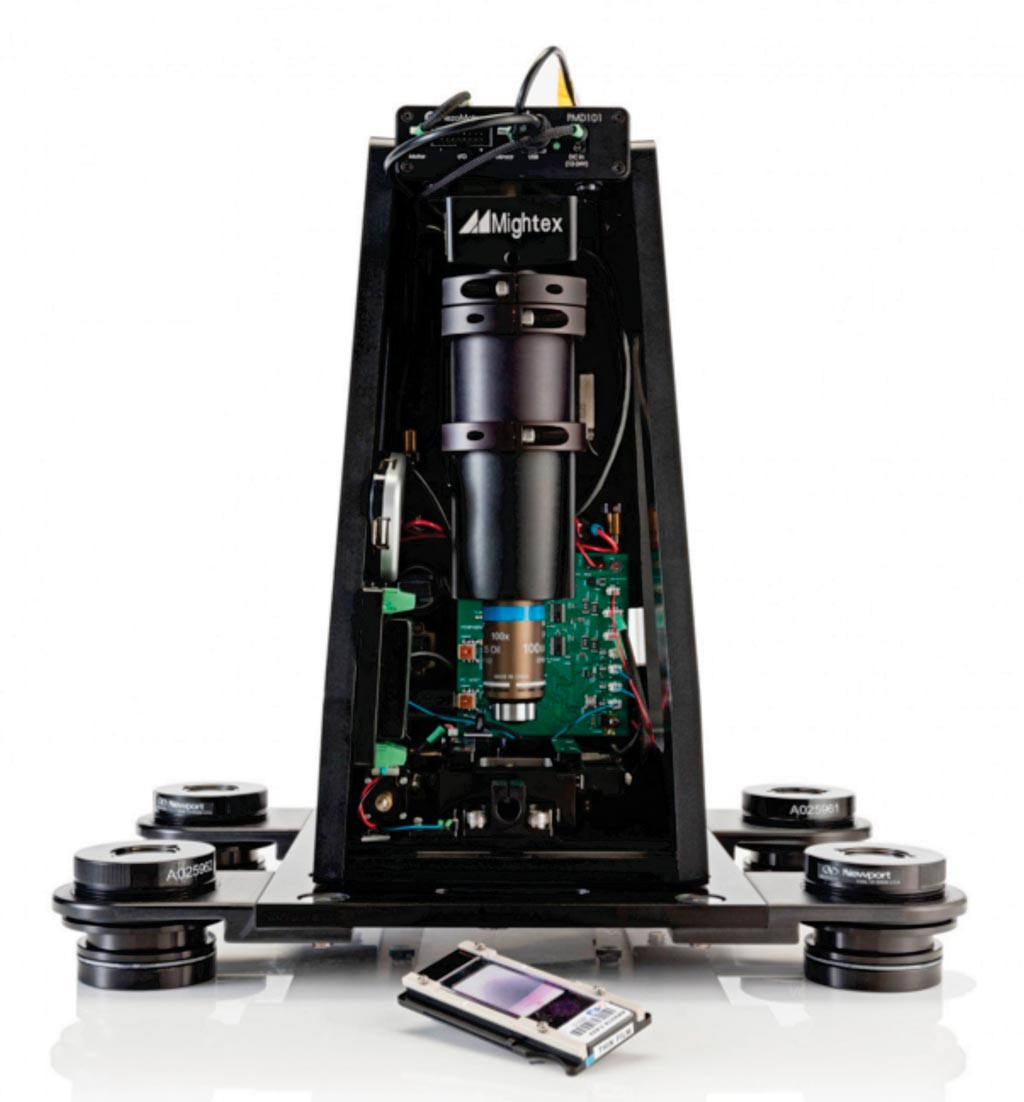
Image: The Autoscope uses deep-learning software to quantify the malaria parasites in a sample (Photo courtesy of Intellectual Ventures).
Microscopic examination of Giemsa-stained blood films remains a major form of diagnosis in malaria case management. However, as with other visualization-based diagnoses, accuracy depends on individual technician performance, making standardization difficult and reliability poor.
Automated image recognition based on machine-learning, utilizing convolutional neural networks, offers potential to overcome these drawbacks. The application of digital image recognition to malaria microscopy, using artificial intelligence algorithms to replace or supplement the human factor in blood film interpretation, have been attempted, usually on thin films.
A team of scientists collaborating with Intellectual Ventures (Bellevue, WA, USA) conducted a cross-sectional, observational trial was conducted at two peripheral primary health facilities in Peru. They enrolled 700 participants whose age was between 5 and 75 years, and had a history of fever in the last three days or elevated temperature on admission. A finger prick blood sample was taken to create blood films for microscopy diagnosis, and additional drops of blood were spotted onto filter paper for subsequent quantitative polymerase chain reaction (qPCR) analysis. A prototype digital microscope device employing an algorithm based on machine-learning, the Autoscope, was assessed for its potential in malaria microscopy.
The investigators reported that at one clinic, sensitivity of Autoscope for diagnosing malaria was 72% and specificity was 85%. Microscopy performance was similar to Autoscope, with sensitivity 68% and specificity 100%. At one clinic, 85% of prepared slides had a minimum of 600 white blood cells (WBCs) imaged, thus meeting Autoscope’s design assumptions. At the second clinic, the sensitivity of Autoscope was 52% and specificity was 70%. Microscopy performance at this second clinic was 42% and specificity was 97%. Only 39% of slides from this clinic met Autoscope’s design assumptions regarding WBCs imaged.
The authors concluded that Autoscope’s diagnostic performance was on par with routine microscopy when slides had adequate blood volume to meet its design assumptions, as represented by results from one clinic. Autoscope’s diagnostic performance was poorer than routine microscopy on slides from the other clinic, which generated slides with lower blood volumes. The study was published on September 25, 2018, in the Malaria Journal.
Related Links:
Intellectual Ventures
Automated image recognition based on machine-learning, utilizing convolutional neural networks, offers potential to overcome these drawbacks. The application of digital image recognition to malaria microscopy, using artificial intelligence algorithms to replace or supplement the human factor in blood film interpretation, have been attempted, usually on thin films.
A team of scientists collaborating with Intellectual Ventures (Bellevue, WA, USA) conducted a cross-sectional, observational trial was conducted at two peripheral primary health facilities in Peru. They enrolled 700 participants whose age was between 5 and 75 years, and had a history of fever in the last three days or elevated temperature on admission. A finger prick blood sample was taken to create blood films for microscopy diagnosis, and additional drops of blood were spotted onto filter paper for subsequent quantitative polymerase chain reaction (qPCR) analysis. A prototype digital microscope device employing an algorithm based on machine-learning, the Autoscope, was assessed for its potential in malaria microscopy.
The investigators reported that at one clinic, sensitivity of Autoscope for diagnosing malaria was 72% and specificity was 85%. Microscopy performance was similar to Autoscope, with sensitivity 68% and specificity 100%. At one clinic, 85% of prepared slides had a minimum of 600 white blood cells (WBCs) imaged, thus meeting Autoscope’s design assumptions. At the second clinic, the sensitivity of Autoscope was 52% and specificity was 70%. Microscopy performance at this second clinic was 42% and specificity was 97%. Only 39% of slides from this clinic met Autoscope’s design assumptions regarding WBCs imaged.
The authors concluded that Autoscope’s diagnostic performance was on par with routine microscopy when slides had adequate blood volume to meet its design assumptions, as represented by results from one clinic. Autoscope’s diagnostic performance was poorer than routine microscopy on slides from the other clinic, which generated slides with lower blood volumes. The study was published on September 25, 2018, in the Malaria Journal.
Related Links:
Intellectual Ventures
Latest Technology News
- Advanced Predictive Algorithms Identify Patients Having Undiagnosed Cancer
- Light Signature Algorithm to Enable Faster and More Precise Medical Diagnoses
- Disposable Microchip Technology Could Selectively Detect HIV in Whole Blood Samples
- Pain-On-A-Chip Microfluidic Device Determines Types of Chronic Pain from Blood Samples
- Innovative, Label-Free Ratiometric Fluorosensor Enables More Sensitive Viral RNA Detection
- Smartphones Could Diagnose Diseases Using Infrared Scans
- Novel Sensor Technology to Enable Early Diagnoses of Metabolic and Cardiovascular Disorders
- 3D Printing Breakthrough Enables Large Scale Development of Tiny Microfluidic Devices
- POC Paper-Based Sensor Platform to Transform Cardiac Diagnostics
- Study Explores Impact of POC Testing on Future of Diagnostics
- Low-Cost, Fast Response Sensor Enables Early and Accurate Detection of Lung Cancer
- Nanotechnology For Cervical Cancer Diagnosis Could Replace Invasive Pap Smears
- Lab-On-Chip Platform to Expedite Cancer Diagnoses
- Biosensing Platform Simultaneously Detects Vitamin C and SARS-CoV-2
- New Lens Method Analyzes Tears for Early Disease Detection
- FET-Based Sensors Pave Way for Portable Diagnostic Devices Capable of Detecting Multiple Diseases
Channels
Clinical Chemistry
view channel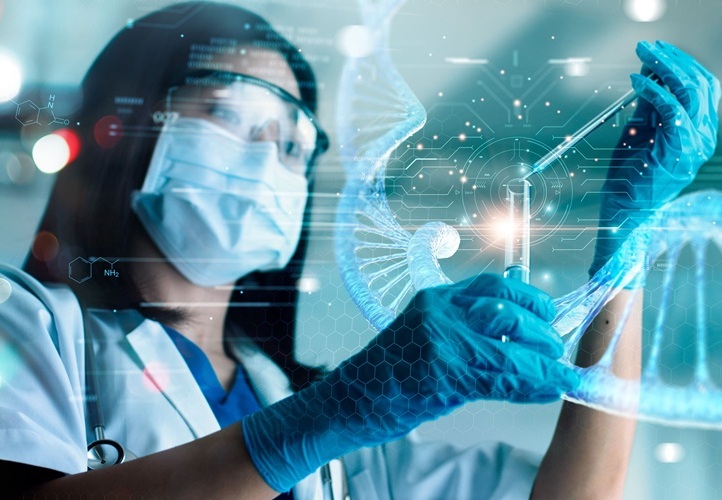
AI-Powered Blood Test Accurately Detects Ovarian Cancer
Ovarian cancer ranks as the fifth leading cause of cancer-related deaths in women, largely due to late-stage diagnoses. Although over 90% of women exhibit symptoms in Stage I, only 20% are diagnosed in... Read more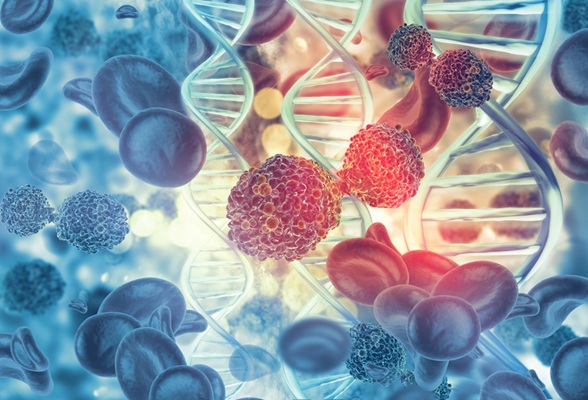
Automated Decentralized cfDNA NGS Assay Identifies Alterations in Advanced Solid Tumors
Current circulating cell-free DNA (cfDNA) assays are typically centralized, requiring specialized handling and transportation of samples. Introducing a flexible, decentralized sequencing system at the... Read moreMass Spectrometry Detects Bacteria Without Time-Consuming Isolation and Multiplication
Speed and accuracy are essential when diagnosing diseases. Traditionally, diagnosing bacterial infections involves the labor-intensive process of isolating pathogens and cultivating bacterial cultures,... Read more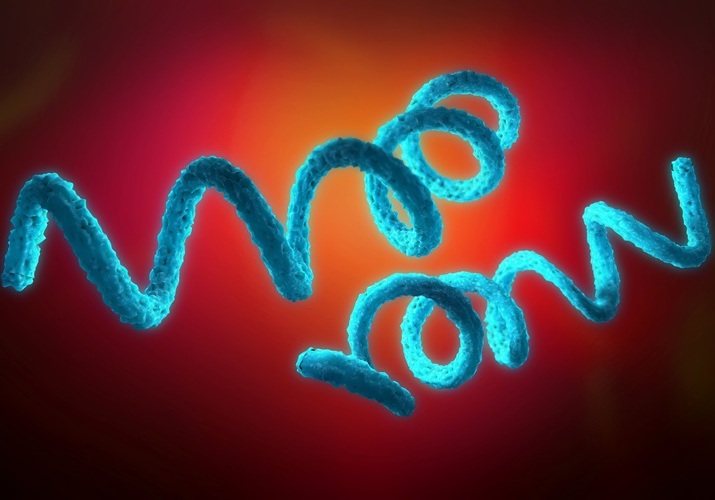
First Comprehensive Syphilis Test to Definitively Diagnose Active Infection In 10 Minutes
In the United States, syphilis cases have surged by nearly 80% from 2018 to 2023, with 209,253 cases recorded in the most recent year of data. Syphilis, which can be transmitted sexually or from mother... Read moreMolecular Diagnostics
view channel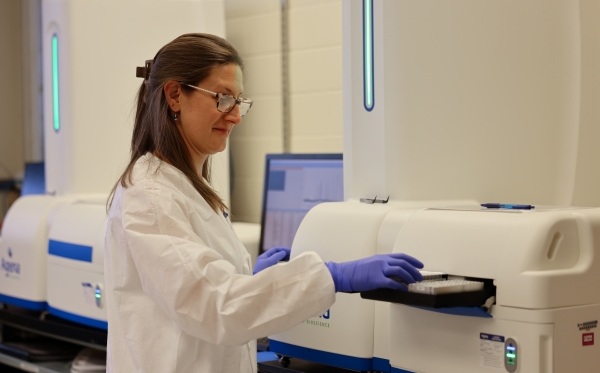
Groundbreaking Molecular Diagnostic Test Accurately Diagnoses Major Genetic Cause of COPD
Chronic obstructive pulmonary disease (COPD) and Alpha-1 Antitrypsin Deficiency (AATD) are both conditions that can cause breathing difficulties, but they differ in their origins and inheritance.... Read more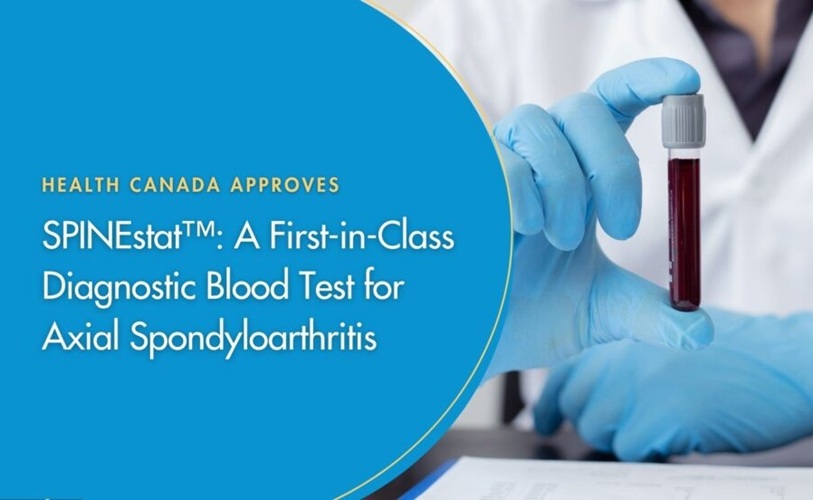
First-in-Class Diagnostic Blood Test Detects Axial Spondyloarthritis
Axial spondyloarthritis (axSpA) is a chronic inflammatory autoimmune condition that typically affects individuals during their most productive years, with symptoms often emerging before the age of 45.... Read more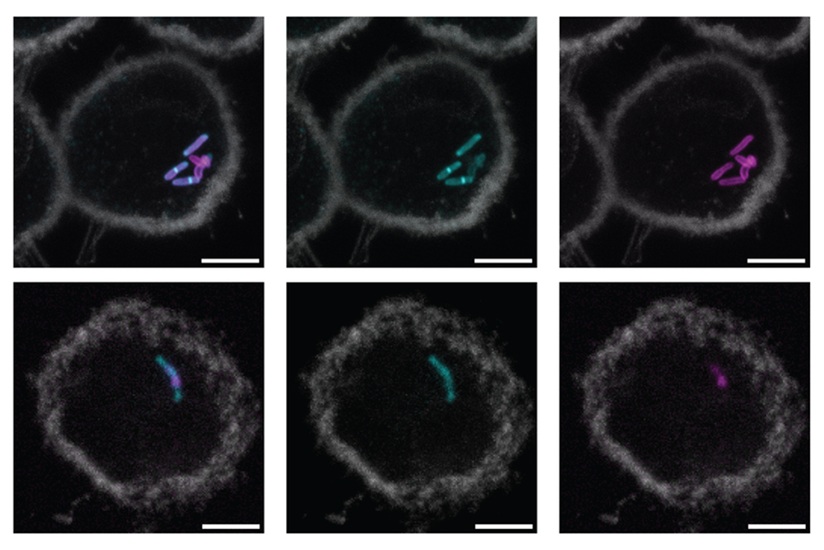
New Molecular Label to Help Develop Simpler and Faster Tuberculosis Tests
Tuberculosis (TB), the deadliest infectious disease globally, is responsible for infecting an estimated 10 million people each year and causing over 1 million deaths annually. While chest X-rays and molecular... Read more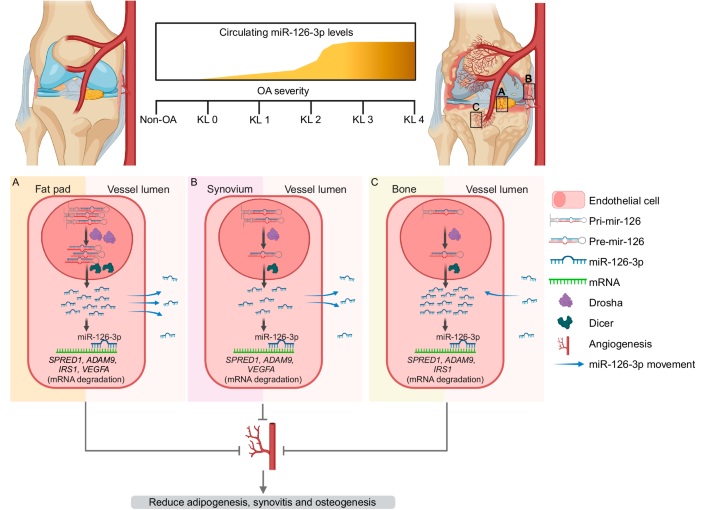
Biomarker Discovery Paves Way for Blood Tests to Detect and Treat Osteoarthritis
The number of individuals affected by osteoarthritis is projected to exceed 1 billion by 2050. The primary risk factor for this common, often painful chronic joint condition is aging, and, like aging itself,... Read moreHematology
view channel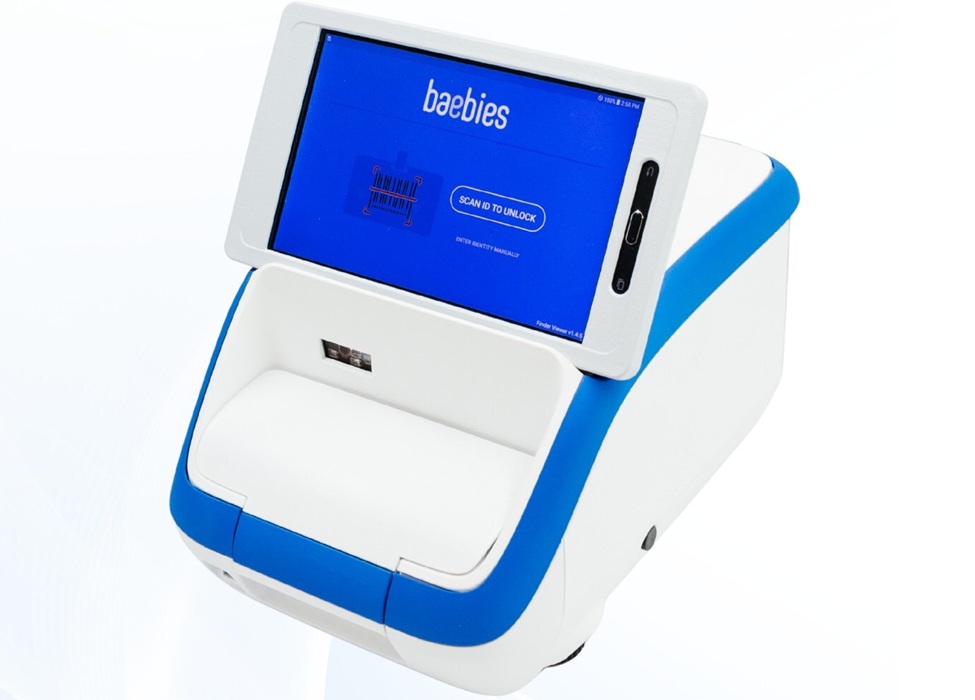
First Point-of-Care Heparin Monitoring Test Provides Results in Under 15 Minutes
Heparin dosing requires careful management to avoid both bleeding and clotting complications. In high-risk situations like extracorporeal membrane oxygenation (ECMO), mortality rates can reach about 50%,... Read more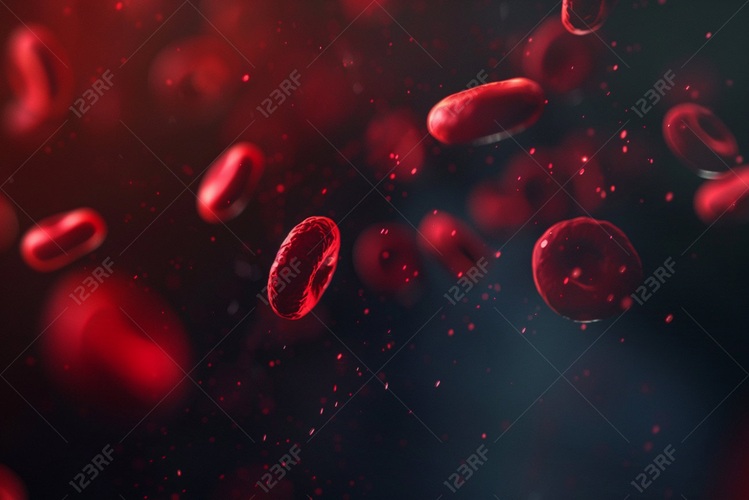
New Scoring System Predicts Risk of Developing Cancer from Common Blood Disorder
Clonal cytopenia of undetermined significance (CCUS) is a blood disorder commonly found in older adults, characterized by mutations in blood cells and a low blood count, but without any obvious cause or... Read moreImmunology
view channel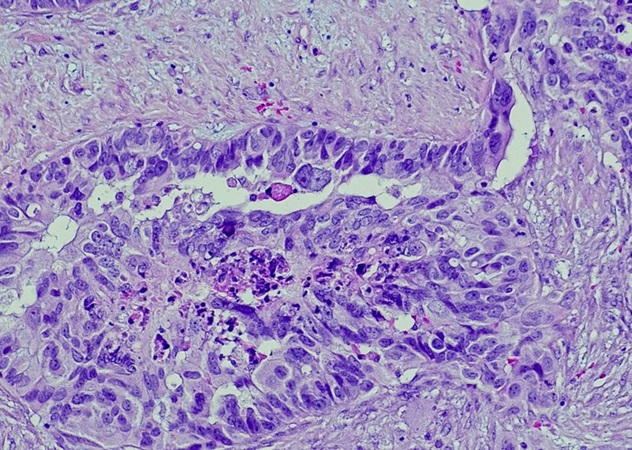
Stem Cell Test Predicts Treatment Outcome for Patients with Platinum-Resistant Ovarian Cancer
Epithelial ovarian cancer frequently responds to chemotherapy initially, but eventually, the tumor develops resistance to the therapy, leading to regrowth. This resistance is partially due to the activation... Read more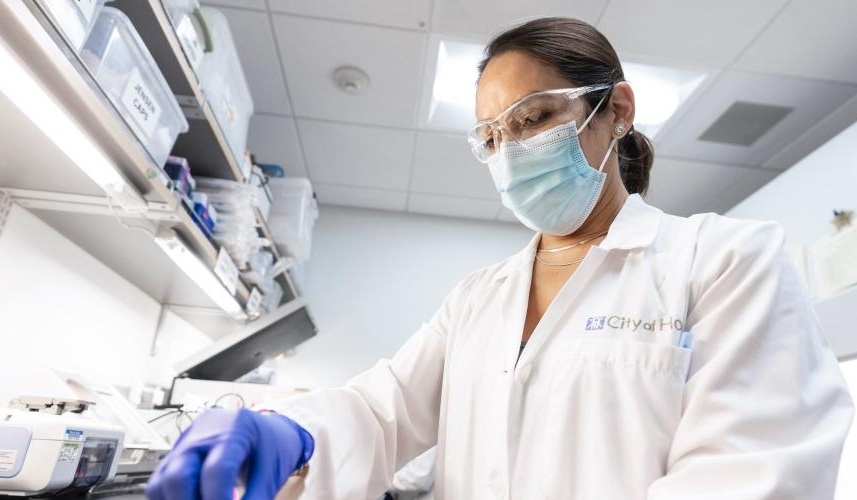
Machine Learning-Enabled Blood Test Predicts Immunotherapy Response in Lymphoma Patients
Chimeric antigen receptor (CAR) T-cell therapy has emerged as one of the most promising recent developments in the treatment of blood cancers. However, over half of non-Hodgkin lymphoma (NHL) patients... Read morePathology
view channel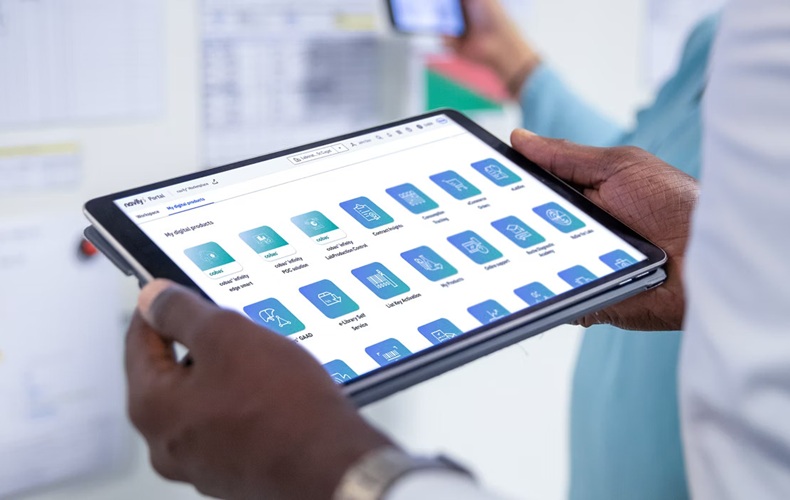
Groundbreaking Chest Pain Triage Algorithm to Transform Cardiac Care
Cardiovascular disease is responsible for a third of all deaths worldwide, and chest pain is the second most common reason for emergency department (ED) visits. With EDs often being some of the busiest... Read more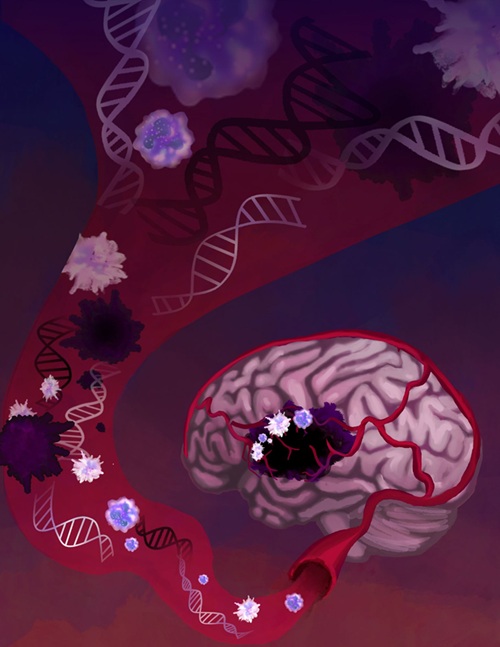
AI-Based Liquid Biopsy Approach to Revolutionize Brain Cancer Detection
Detecting brain cancers remains extremely challenging, with many patients only receiving a diagnosis at later stages after symptoms like headaches, seizures, or cognitive issues appear. Late-stage diagnoses... Read moreTechnology
view channel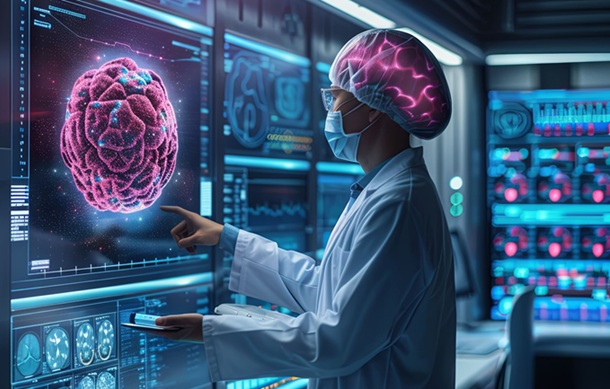
Advanced Predictive Algorithms Identify Patients Having Undiagnosed Cancer
Two newly developed advanced predictive algorithms leverage a person’s health conditions and basic blood test results to accurately predict the likelihood of having an undiagnosed cancer, including ch... Read more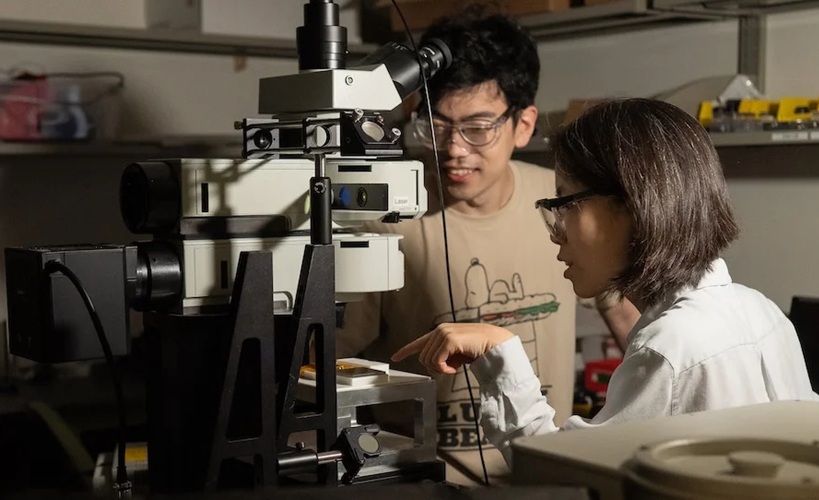
Light Signature Algorithm to Enable Faster and More Precise Medical Diagnoses
Every material or molecule interacts with light in a unique way, creating a distinct pattern, much like a fingerprint. Optical spectroscopy, which involves shining a laser on a material and observing how... Read more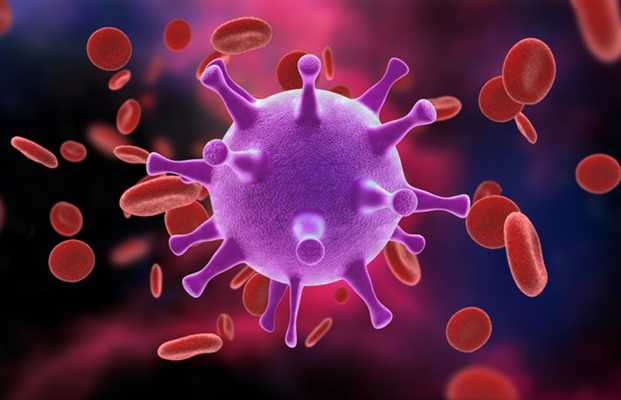
Disposable Microchip Technology Could Selectively Detect HIV in Whole Blood Samples
As of the end of 2023, approximately 40 million people globally were living with HIV, and around 630,000 individuals died from AIDS-related illnesses that same year. Despite a substantial decline in deaths... Read more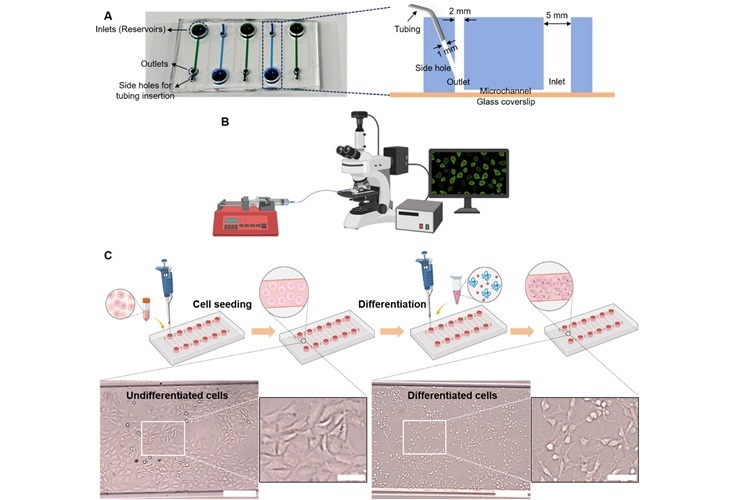
Pain-On-A-Chip Microfluidic Device Determines Types of Chronic Pain from Blood Samples
Chronic pain is a widespread condition that remains difficult to manage, and existing clinical methods for its treatment rely largely on self-reporting, which can be subjective and especially problematic... Read moreIndustry
view channel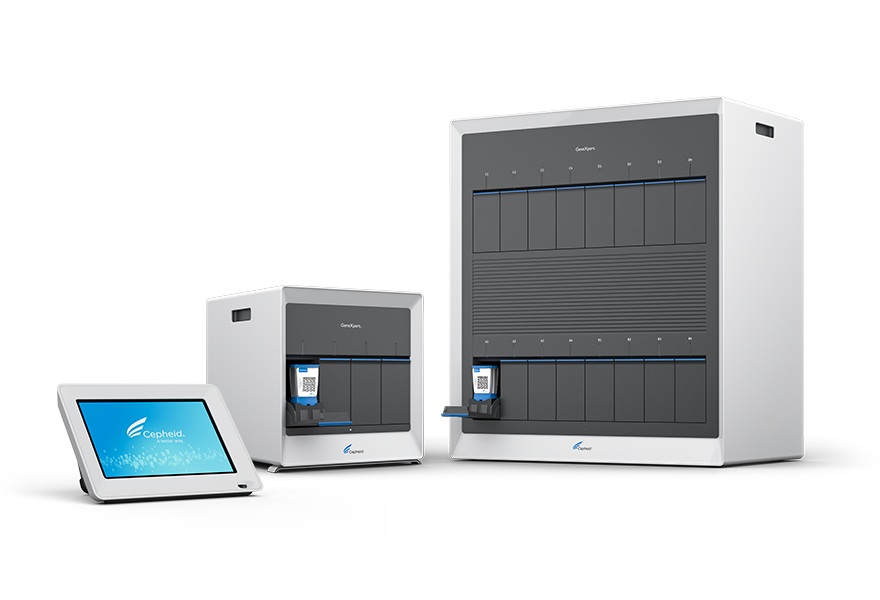
Cepheid and Oxford Nanopore Technologies Partner on Advancing Automated Sequencing-Based Solutions
Cepheid (Sunnyvale, CA, USA), a leading molecular diagnostics company, and Oxford Nanopore Technologies (Oxford, UK), the company behind a new generation of sequencing-based molecular analysis technologies,... Read more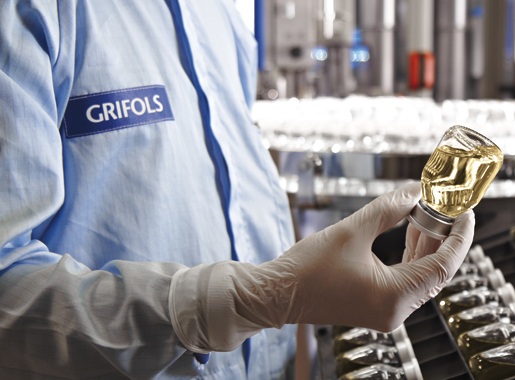
Grifols and Tecan’s IBL Collaborate on Advanced Biomarker Panels
Grifols (Barcelona, Spain), one of the world’s leading producers of plasma-derived medicines and innovative diagnostic solutions, is expanding its offer in clinical diagnostics through a strategic partnership... Read more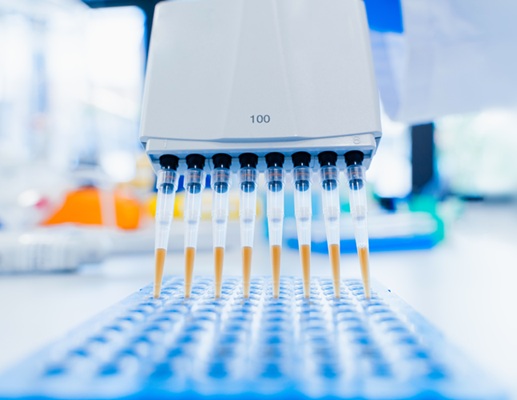