Self-Teaching AI Algorithm Uses Pathology Images to Diagnose Rare Diseases
By LabMedica International staff writers Posted on 11 Oct 2022 |
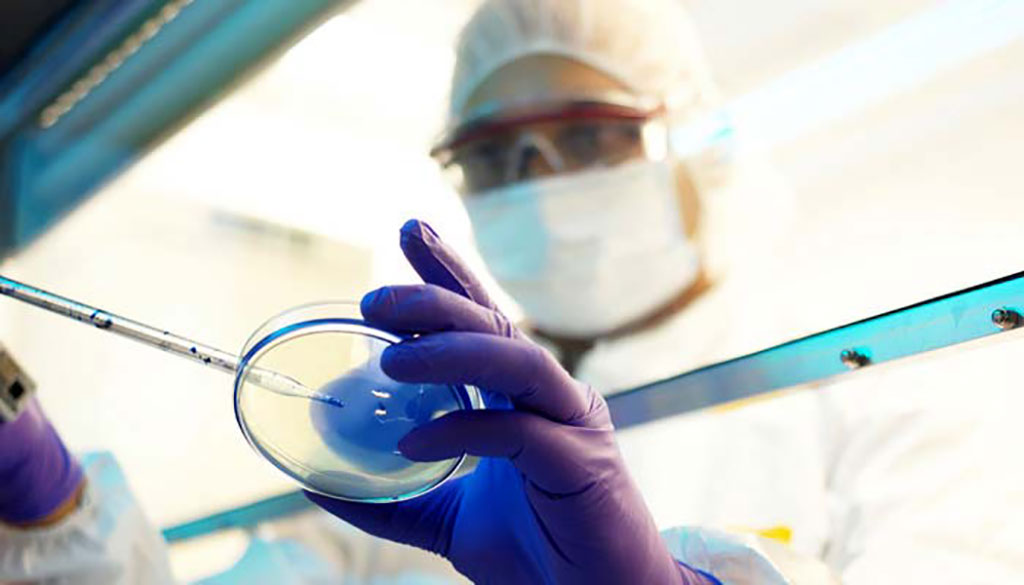
Rare diseases are often difficult to diagnose and predicting the best course of treatment can be challenging for clinicians. Modern electronic databases can store an immense amount of digital records and reference images, particularly in pathology through whole slide images (WSIs). However, the gigapixel size of each individual WSI and the ever-increasing number of images in large repositories, means that search and retrieval of WSIs can be slow and complicated. As a result, scalability remains a pertinent roadblock for efficient use. To solve this issue, researchers have now developed a deep learning algorithm that can teach itself to learn features which can then be used to find similar cases in large pathology image repositories.
Known as SISH (Self-Supervised Image search for Histology), the new tool developed by investigators at Brigham and Women’s Hospital (Boston, MA, USA) acts like a search engine for pathology images and has many potential applications, including identifying rare diseases and helping clinicians determine which patients are likely to respond to similar therapies. The algorithm teaches itself to learn feature representations which can be used to find cases with analogous features in pathology at a constant speed regardless of the size of the database.
In their study, the researchers tested the speed and ability of SISH to retrieve interpretable disease subtype information for common and rare cancers. The algorithm successfully retrieved images with speed and accuracy from a database of tens of thousands of whole slide images from over 22,000 patient cases, with over 50 different disease types and over a dozen anatomical sites. The speed of retrieval outperformed other methods in many scenarios, including disease subtype retrieval, particularly as the image database size scaled into the thousands of images. Even while the repositories expanded in size, SISH was still able to maintain a constant search speed.
The self-teaching algorithm, however, has some limitations including a large memory requirement, limited context awareness within large tissue slides and the fact that it is limited to a single imaging modality. Overall, the algorithm demonstrated the ability to efficiently retrieve images independent of repository size and in diverse datasets. It also demonstrated proficiency in diagnosis of rare disease types and the ability to serve as a search engine to recognize certain regions of images that may be relevant for diagnosis. This work may greatly inform future disease diagnosis, prognosis, and analysis.
“We show that our system can assist with the diagnosis of rare diseases and find cases with similar morphologic patterns without the need for manual annotations, and large datasets for supervised training,” said senior author Faisal Mahmood, PhD, in the Brigham’s Department of Pathology. “This system has the potential to improve pathology training, disease subtyping, tumor identification, and rare morphology identification.”
“As the sizes of image databases continue to grow, we hope that SISH will be useful in making identification of diseases easier,” added Mahmood. “We believe one important future direction in this area is multimodal case retrieval which involves jointly using pathology, radiology, genomic and electronic medical record data to find similar patient cases.”
Related Links:
Brigham and Women’s Hospital
Latest Pathology News
- AI Advancements Enable Leap into 3D Pathology
- New Blood Test Device Modeled on Leeches to Help Diagnose Malaria
- Robotic Blood Drawing Device to Revolutionize Sample Collection for Diagnostic Testing
- Use of DICOM Images for Pathology Diagnostics Marks Significant Step towards Standardization
- First of Its Kind Universal Tool to Revolutionize Sample Collection for Diagnostic Tests
- AI-Powered Digital Imaging System to Revolutionize Cancer Diagnosis
- New Mycobacterium Tuberculosis Panel to Support Real-Time Surveillance and Combat Antimicrobial Resistance
- New Method Offers Sustainable Approach to Universal Metabolic Cancer Diagnosis
- Spatial Tissue Analysis Identifies Patterns Associated With Ovarian Cancer Relapse
- Unique Hand-Warming Technology Supports High-Quality Fingertip Blood Sample Collection
- Image-Based AI Shows Promise for Parasite Detection in Digitized Stool Samples
- Deep Learning Powered AI Algorithms Improve Skin Cancer Diagnostic Accuracy
- Microfluidic Device for Cancer Detection Precisely Separates Tumor Entities
- Virtual Skin Biopsy Determines Presence of Cancerous Cells
- AI Detects Viable Tumor Cells for Accurate Bone Cancer Prognoses Post Chemotherapy
- First Ever Technique Identifies Single Cancer Cells in Blood for Targeted Treatments
Channels
Clinical Chemistry
view channel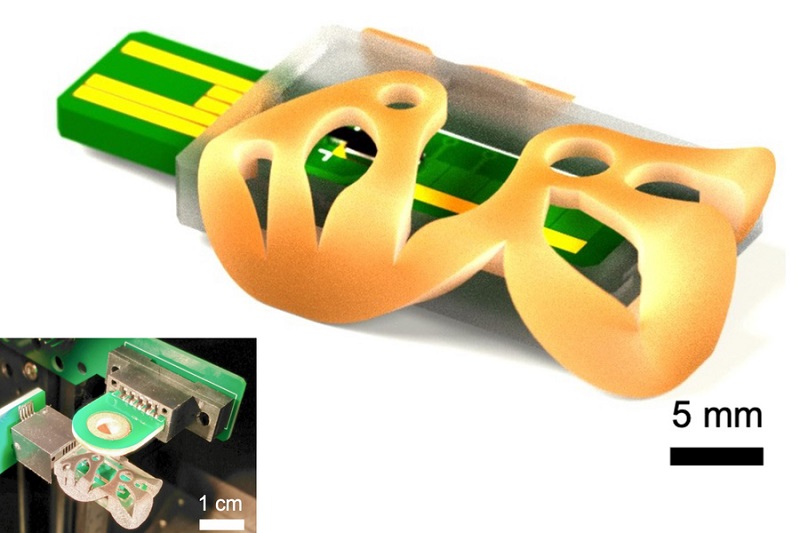
3D Printed Point-Of-Care Mass Spectrometer Outperforms State-Of-The-Art Models
Mass spectrometry is a precise technique for identifying the chemical components of a sample and has significant potential for monitoring chronic illness health states, such as measuring hormone levels... Read more.jpg)
POC Biomedical Test Spins Water Droplet Using Sound Waves for Cancer Detection
Exosomes, tiny cellular bioparticles carrying a specific set of proteins, lipids, and genetic materials, play a crucial role in cell communication and hold promise for non-invasive diagnostics.... Read more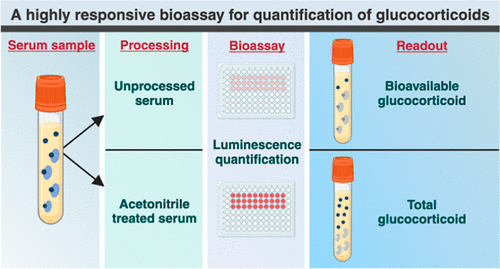
Highly Reliable Cell-Based Assay Enables Accurate Diagnosis of Endocrine Diseases
The conventional methods for measuring free cortisol, the body's stress hormone, from blood or saliva are quite demanding and require sample processing. The most common method, therefore, involves collecting... Read moreMolecular Diagnostics
view channel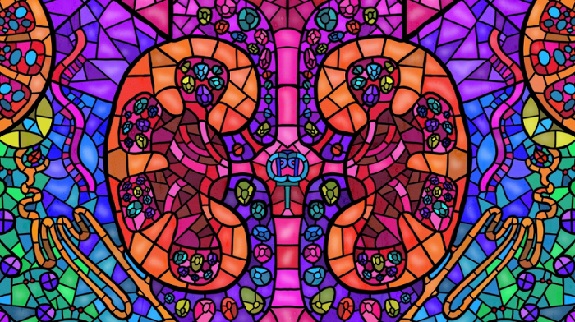
Novel Biomarkers to Improve Diagnosis of Renal Cell Carcinoma Subtypes
Renal cell carcinomas (RCCs) are notably diverse, encompassing over 20 distinct subtypes and generally categorized into clear cell and non-clear cell types; around 20% of all RCCs fall into the non-clear... Read more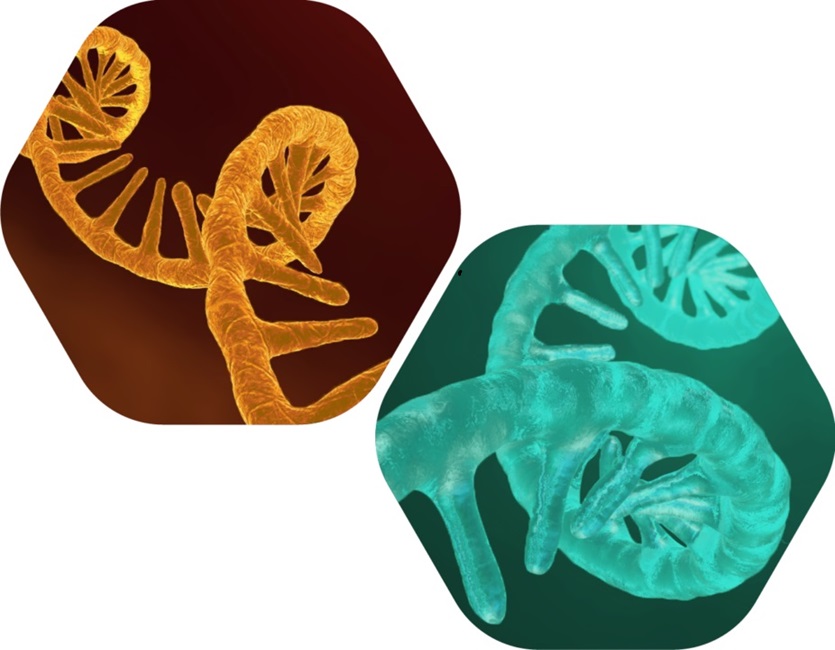
RNA-Powered Molecular Test to Help Combat Early-Age Onset Colorectal Cancer
Colorectal cancer (CRC) ranks as the second most lethal cancer in the United States. Nevertheless, many Americans eligible for screening do not undergo testing due to limited access or reluctance towards... Read moreHematology
view channel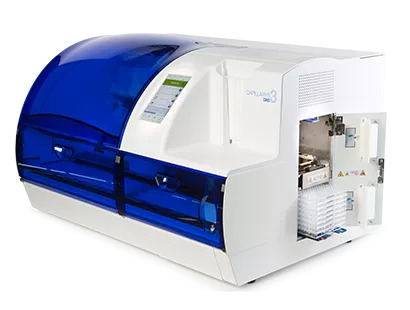
Next Generation Instrument Screens for Hemoglobin Disorders in Newborns
Hemoglobinopathies, the most widespread inherited conditions globally, affect about 7% of the population as carriers, with 2.7% of newborns being born with these conditions. The spectrum of clinical manifestations... Read more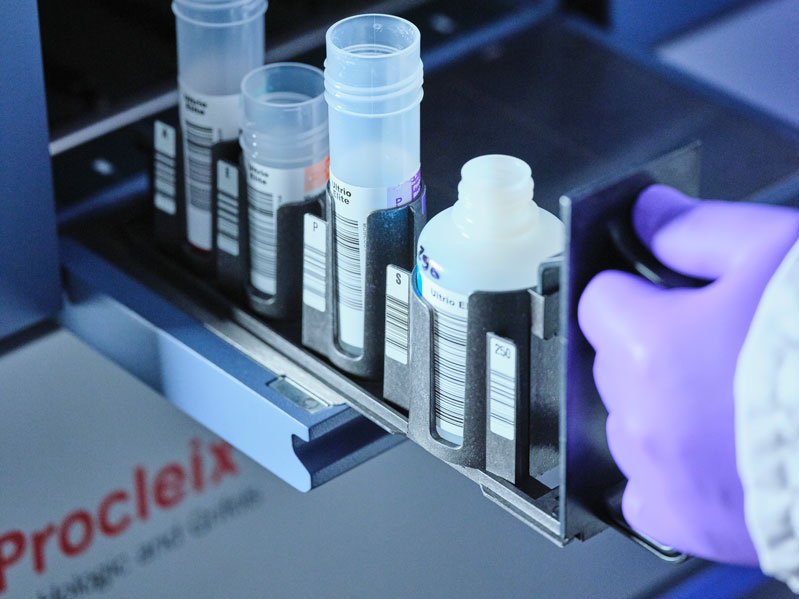
First 4-in-1 Nucleic Acid Test for Arbovirus Screening to Reduce Risk of Transfusion-Transmitted Infections
Arboviruses represent an emerging global health threat, exacerbated by climate change and increased international travel that is facilitating their spread across new regions. Chikungunya, dengue, West... Read more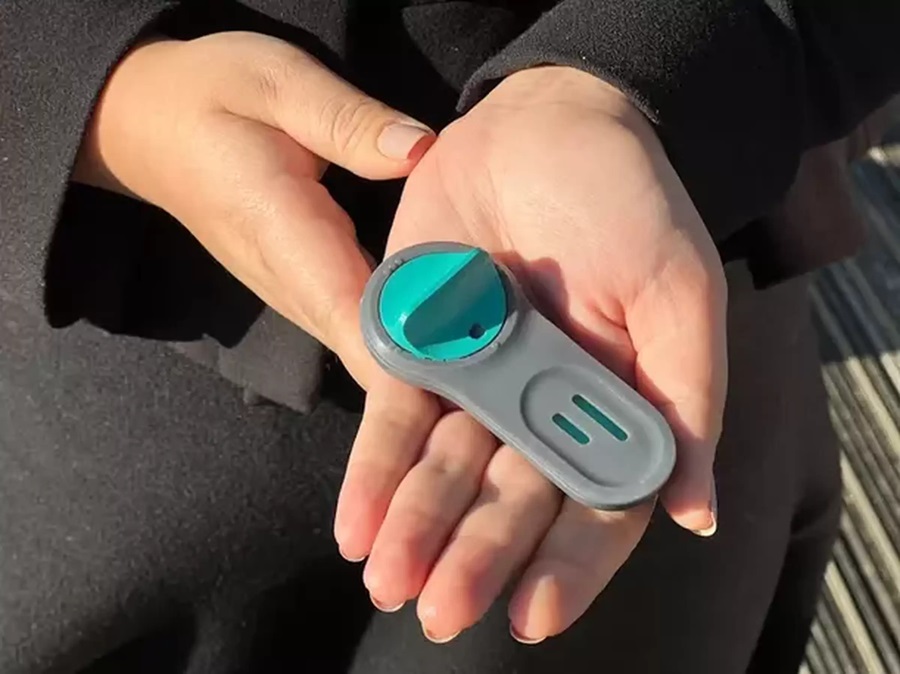
POC Finger-Prick Blood Test Determines Risk of Neutropenic Sepsis in Patients Undergoing Chemotherapy
Neutropenia, a decrease in neutrophils (a type of white blood cell crucial for fighting infections), is a frequent side effect of certain cancer treatments. This condition elevates the risk of infections,... Read more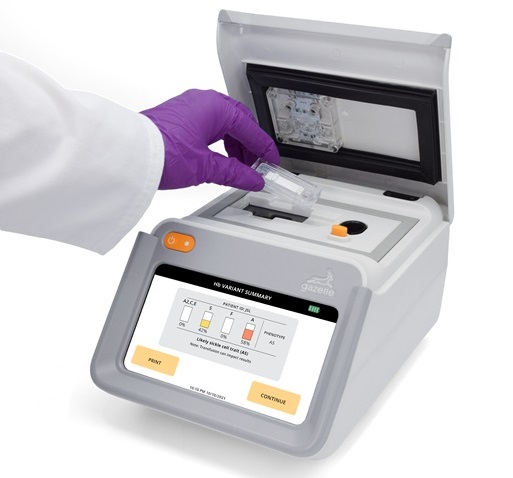
First Affordable and Rapid Test for Beta Thalassemia Demonstrates 99% Diagnostic Accuracy
Hemoglobin disorders rank as some of the most prevalent monogenic diseases globally. Among various hemoglobin disorders, beta thalassemia, a hereditary blood disorder, affects about 1.5% of the world's... Read moreImmunology
view channel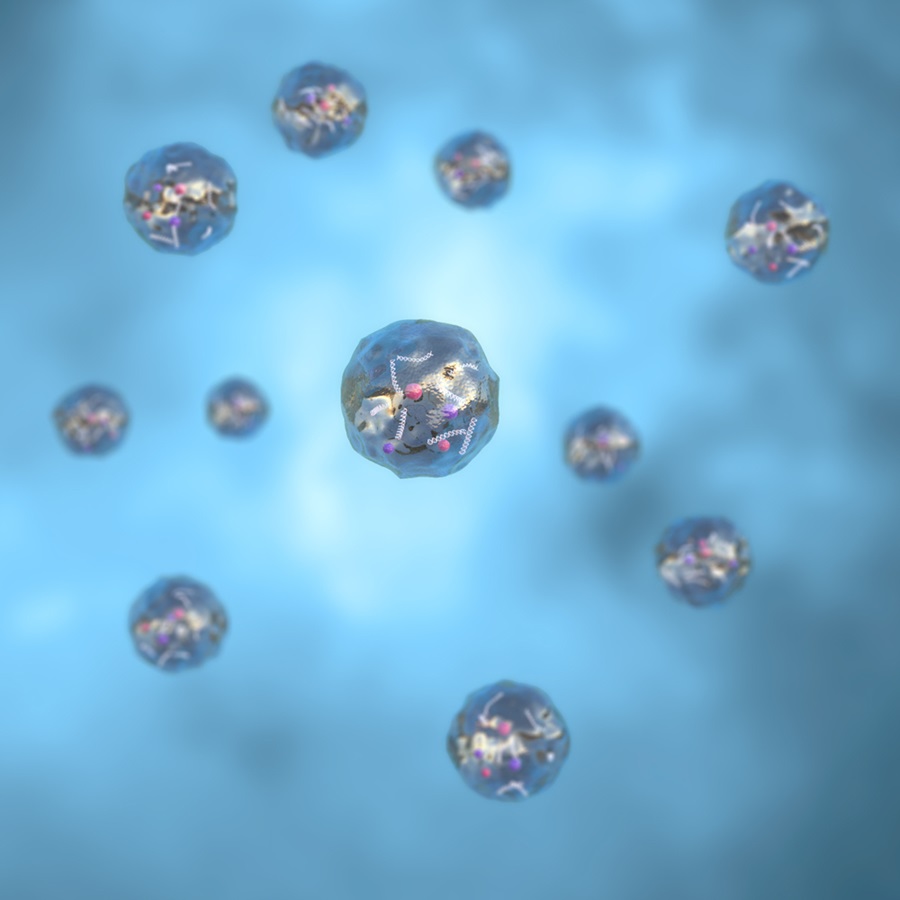
Diagnostic Blood Test for Cellular Rejection after Organ Transplant Could Replace Surgical Biopsies
Transplanted organs constantly face the risk of being rejected by the recipient's immune system which differentiates self from non-self using T cells and B cells. T cells are commonly associated with acute... Read more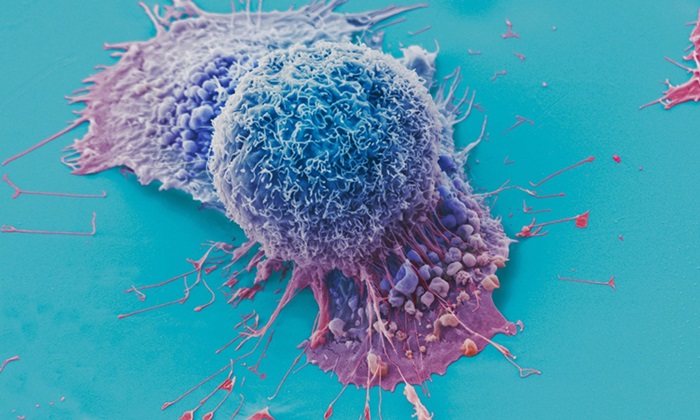
AI Tool Precisely Matches Cancer Drugs to Patients Using Information from Each Tumor Cell
Current strategies for matching cancer patients with specific treatments often depend on bulk sequencing of tumor DNA and RNA, which provides an average profile from all cells within a tumor sample.... Read more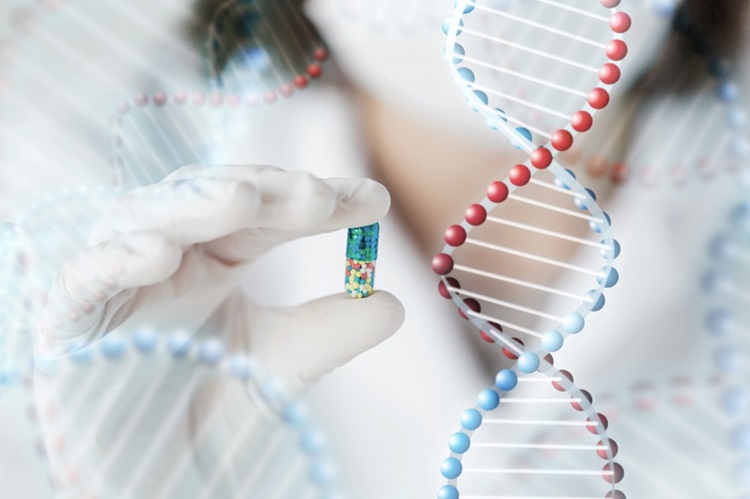
Genetic Testing Combined With Personalized Drug Screening On Tumor Samples to Revolutionize Cancer Treatment
Cancer treatment typically adheres to a standard of care—established, statistically validated regimens that are effective for the majority of patients. However, the disease’s inherent variability means... Read moreMicrobiology
view channel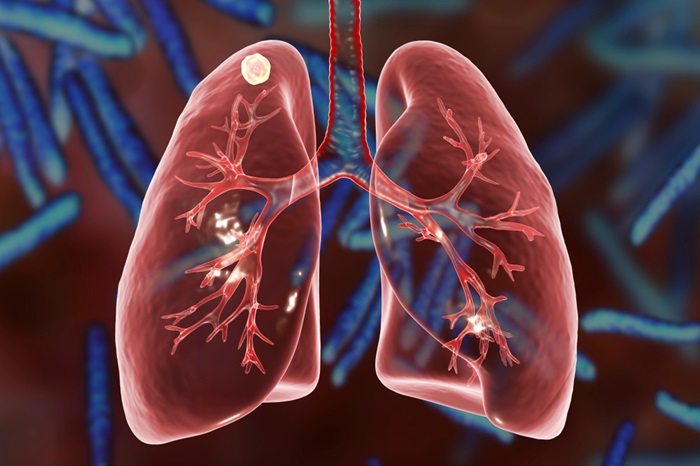
Integrated Solution Ushers New Era of Automated Tuberculosis Testing
Tuberculosis (TB) is responsible for 1.3 million deaths every year, positioning it as one of the top killers globally due to a single infectious agent. In 2022, around 10.6 million people were diagnosed... Read more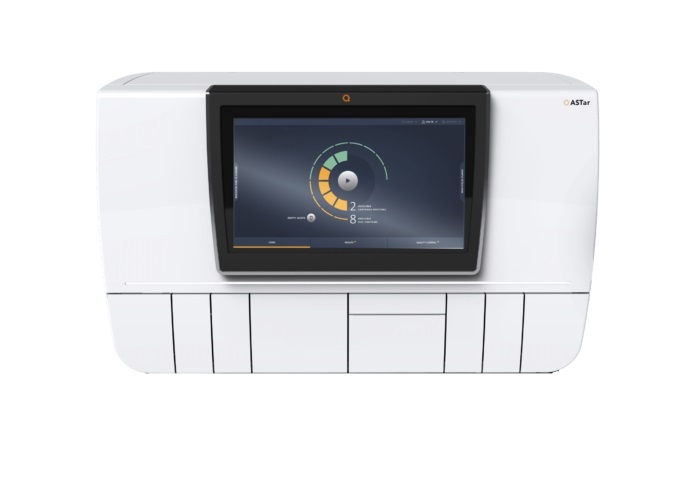
Automated Sepsis Test System Enables Rapid Diagnosis for Patients with Severe Bloodstream Infections
Sepsis affects up to 50 million people globally each year, with bacteraemia, formerly known as blood poisoning, being a major cause. In the United States alone, approximately two million individuals are... Read more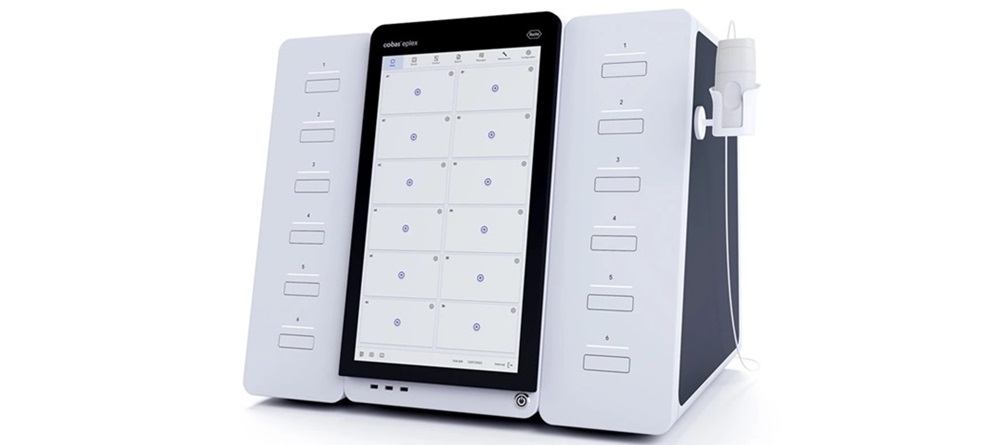
Enhanced Rapid Syndromic Molecular Diagnostic Solution Detects Broad Range of Infectious Diseases
GenMark Diagnostics (Carlsbad, CA, USA), a member of the Roche Group (Basel, Switzerland), has rebranded its ePlex® system as the cobas eplex system. This rebranding under the globally renowned cobas name... Read more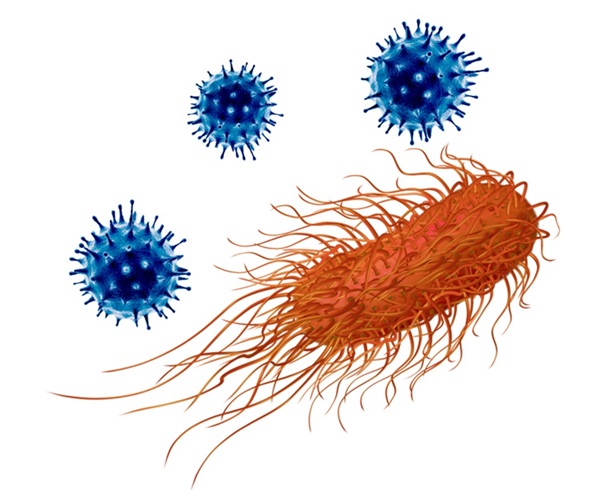
Clinical Decision Support Software a Game-Changer in Antimicrobial Resistance Battle
Antimicrobial resistance (AMR) is a serious global public health concern that claims millions of lives every year. It primarily results from the inappropriate and excessive use of antibiotics, which reduces... Read moreTechnology
view channel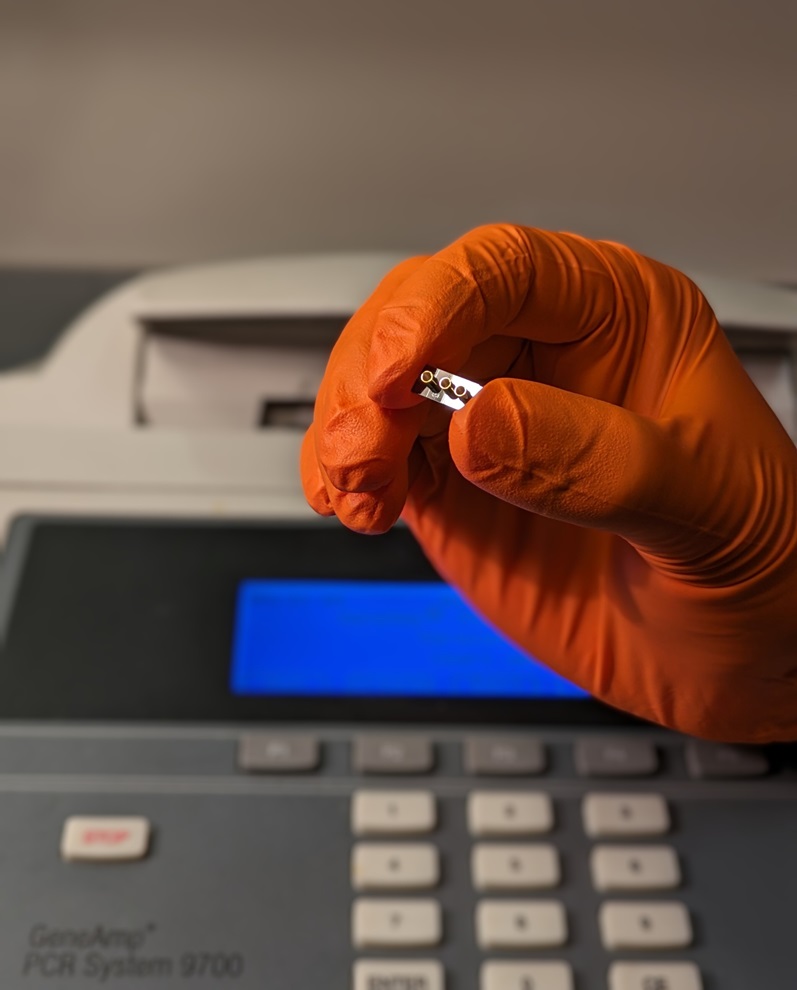
New Diagnostic System Achieves PCR Testing Accuracy
While PCR tests are the gold standard of accuracy for virology testing, they come with limitations such as complexity, the need for skilled lab operators, and longer result times. They also require complex... Read more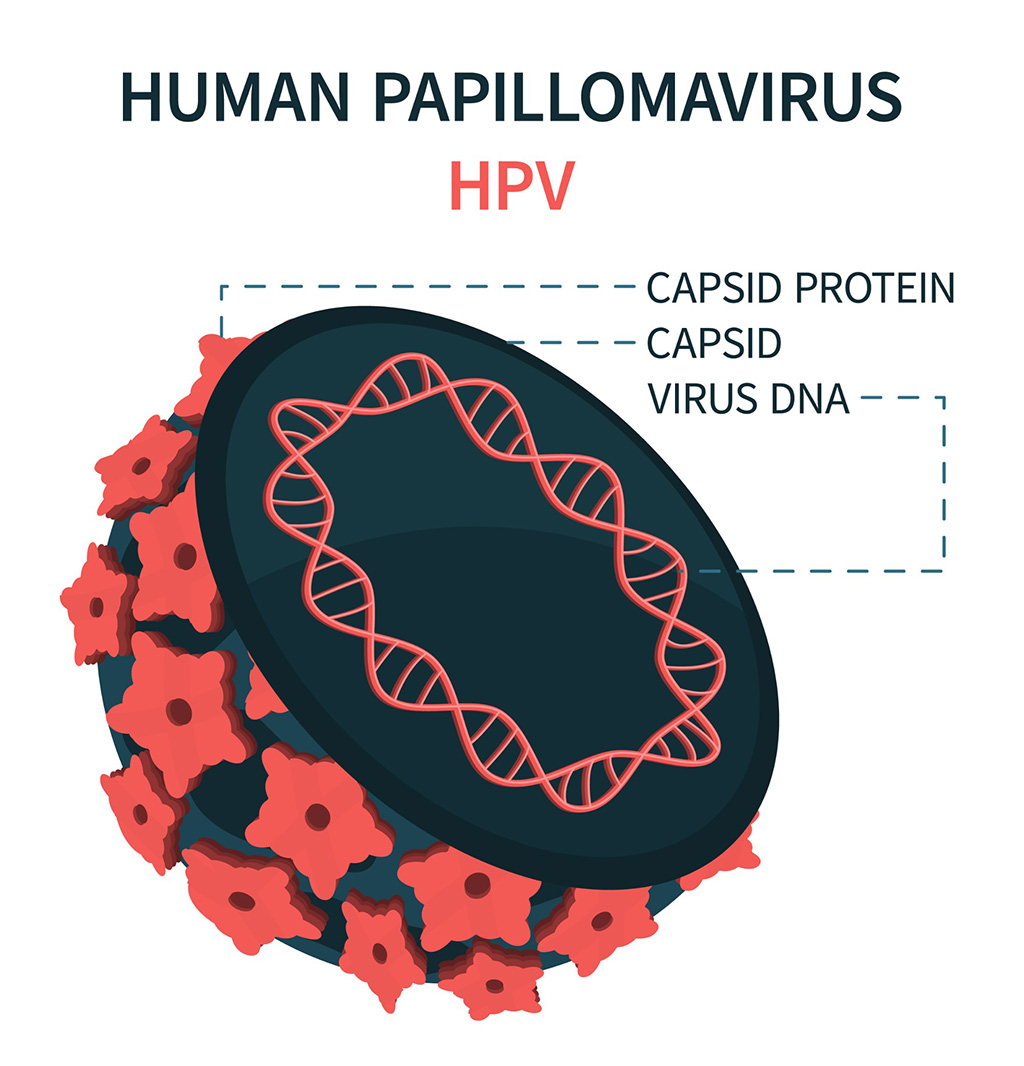
DNA Biosensor Enables Early Diagnosis of Cervical Cancer
Molybdenum disulfide (MoS2), recognized for its potential to form two-dimensional nanosheets like graphene, is a material that's increasingly catching the eye of the scientific community.... Read more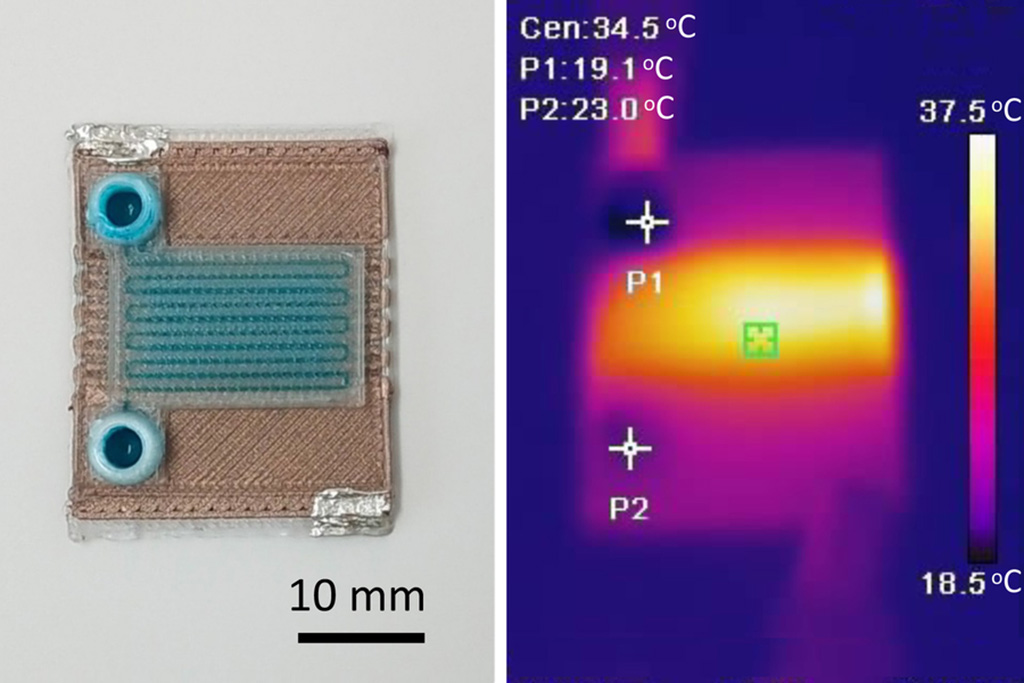
Self-Heating Microfluidic Devices Can Detect Diseases in Tiny Blood or Fluid Samples
Microfluidics, which are miniature devices that control the flow of liquids and facilitate chemical reactions, play a key role in disease detection from small samples of blood or other fluids.... Read more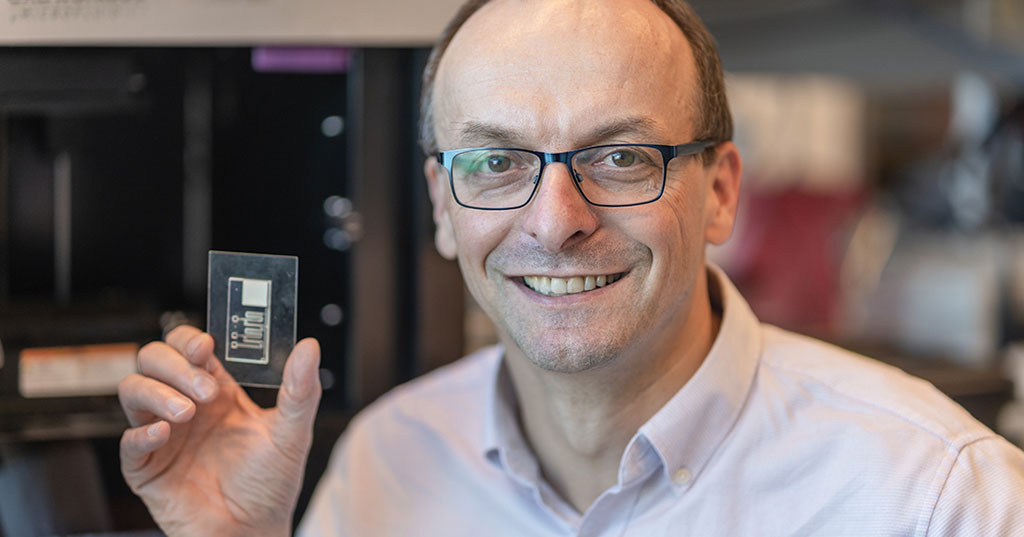
Breakthrough in Diagnostic Technology Could Make On-The-Spot Testing Widely Accessible
Home testing gained significant importance during the COVID-19 pandemic, yet the availability of rapid tests is limited, and most of them can only drive one liquid across the strip, leading to continued... Read moreIndustry
view channel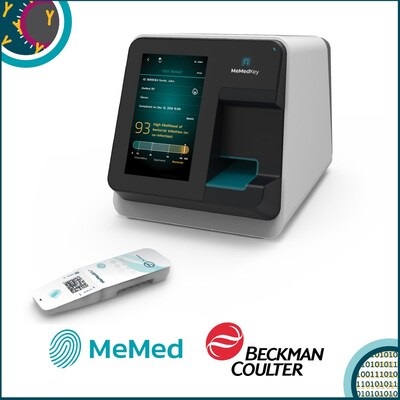
Beckman Coulter and MeMed Expand Host Immune Response Diagnostics Partnership
Beckman Coulter Diagnostics (Brea, CA, USA) and MeMed BV (Haifa, Israel) have expanded their host immune response diagnostics partnership. Beckman Coulter is now an authorized distributor of the MeMed... Read more_1.jpg)
Thermo Fisher and Bio-Techne Enter Into Strategic Distribution Agreement for Europe
Thermo Fisher Scientific (Waltham, MA USA) has entered into a strategic distribution agreement with Bio-Techne Corporation (Minneapolis, MN, USA), resulting in a significant collaboration between two industry... Read more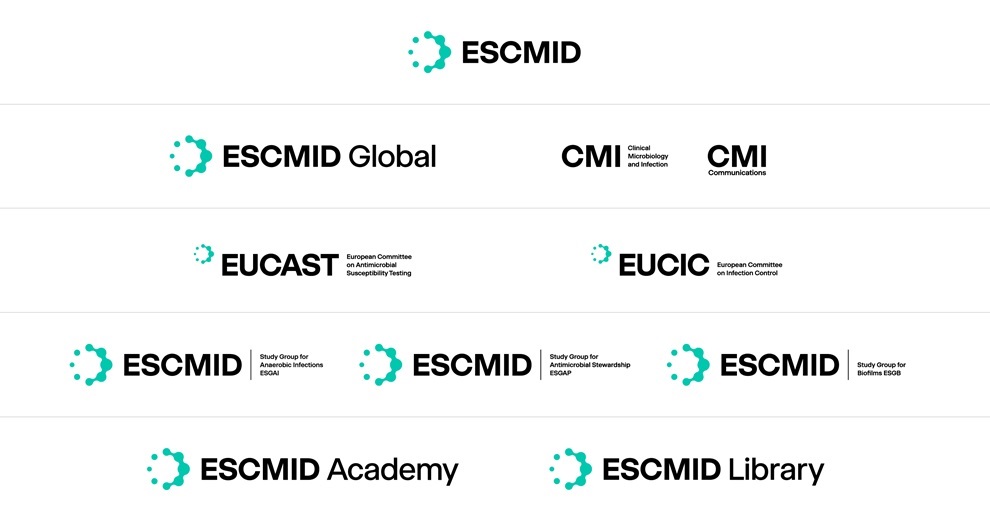
ECCMID Congress Name Changes to ESCMID Global
Over the last few years, the European Society of Clinical Microbiology and Infectious Diseases (ESCMID, Basel, Switzerland) has evolved remarkably. The society is now stronger and broader than ever before... Read more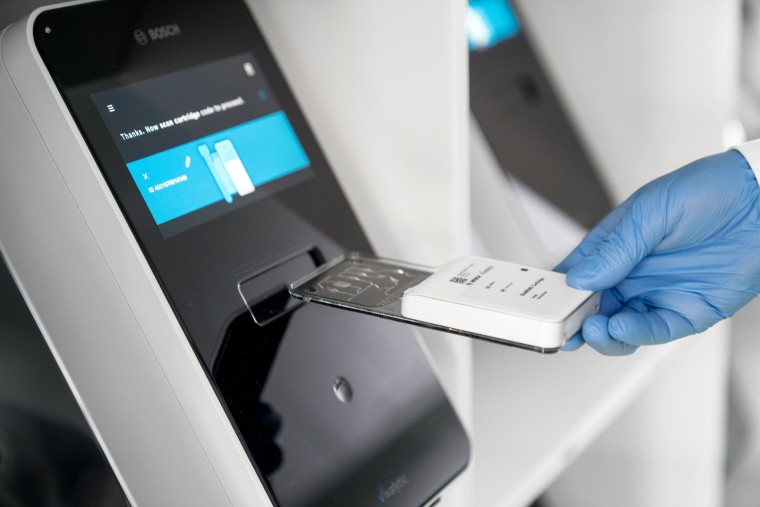