Image-Based AI Shows Promise for Parasite Detection in Digitized Stool Samples
Posted on 17 Apr 2024
Infections from soil-transmitted helminths (STHs), commonly known as intestinal parasitic worms, are among the most widespread neglected tropical diseases and impose a significant health burden in low- and middle-income countries, particularly among school-aged children. These infections often lead to chronic health issues that can cause disability, social stigma, and for their substantial economic impacts on communities. STHs are notorious role in nutrient loss, which can contribute to neurocognitive impairments, stunted growth and development, and persistent fatigue in affected children. Additionally, these parasites are a major cause of morbidity and complications during pregnancy. The standard diagnostic method for STHs involves manual microscopy, which requires up to 10 minutes per slide and is hindered by a lack of skilled professionals and access to necessary equipment and lab infrastructure in highly affected regions. There is a pressing need for improved diagnostic techniques, particularly for detecting infections of mild intensity, to effectively manage and aim for the elimination of STHs as a public health concern. Now, an artificial intelligence (AI) microscopy system has been shown to accurately identify intestinal worm infections, especially light-intensity infections that could be overlooked when using manual microscopy.
The new study by a multi-institutional team of specialists from the Karolinska Institute (Stockholm, Sweden) and University of Helsinki (Helsinki, Finland) marked the first clinical trial of the system to detect worm infections in a remote setting with whole-slide images. The study was carried out in rural areas of Kwale County, Kenya, where there is a high prevalence of STHs among children. During the study, 1,335 school-aged children were screened using the deep learning-based system for parasitic worm egg detection, with results compared against those obtained through expert manual microscopy.
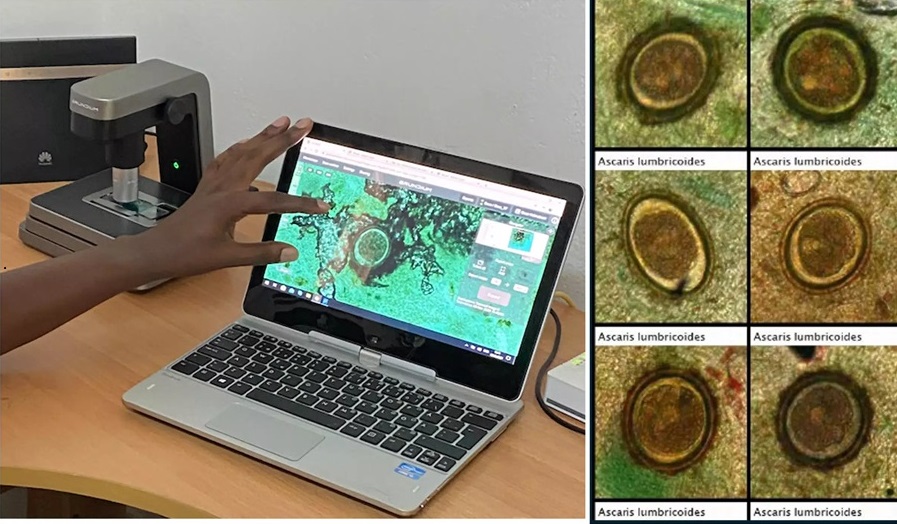
The analysis of digitally scanned stool samples using the deep learning system demonstrated high diagnostic accuracy in identifying three common types of parasitic worms: Ascaris lumbricoides (giant roundworm), Trichuris trichiura (whipworm), and hookworm (Ancylostoma duodenale or Necator americanus). The AI was able to detect between 76% and 92% of the infections identified by trained lab technicians, depending on the type of worm. Notably, the AI system identified a significant number of light-intensity infections that were missed in manual microscopy evaluations. In fact, in 79 samples (10% of the total), which were initially determined to be negative by manual microscopy, the AI system detected the presence of parasitic worm eggs. Moreover, the AI system provides a digital record of each sample that can be preserved for further analysis, offering a significant advantage over human samples, which typically dry out within hours and become more challenging for further analysis.
“We have shown that we can use our testing in a resource-limited setting and get high accuracy. Our method was especially efficient in light-intensity infections,” said Principal Investigator Professor Johan Lundin, MD, PhD, from the Karolinska Institutet. “With AI, once our sample is digitised, it takes just a few second and looks at the entire sample and is able to very accurately find the parasite eggs.”
Related Links:
Karolinska Institute
University of Helsinki