Prostate Cancer Diagnosed Better Using Artificial Intelligence
By LabMedica International staff writers Posted on 22 Jan 2020 |
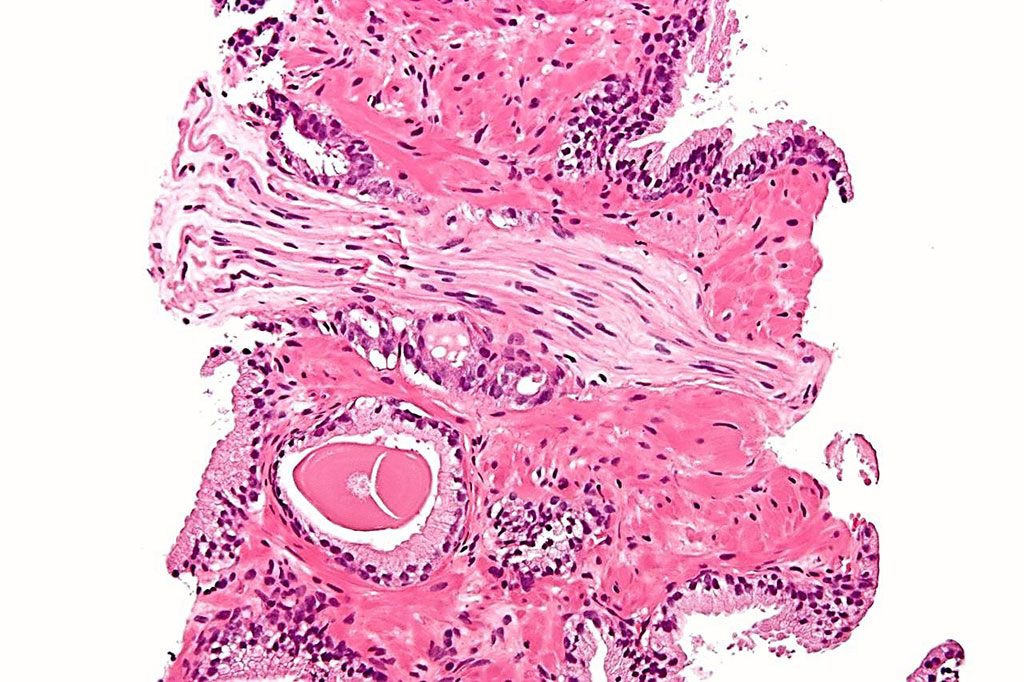
Image: Photomicrograph of a histological biopsy of a prostatic adenocarcinoma, conventional (acinar) type, the most common form of prostate cancer (Photo courtesy of Nephron).
Prostate cancer is a frequently occurring type of cancer, but not always aggressive: more men die with prostate cancer than from prostate cancer. However, its treatment has many consequences for the quality of life of patients, so determining aggressiveness is an important step in choosing a treatment.
To determine the aggressiveness of the cancer, biopsies are taken from the prostate, which are scored by a pathologist. This 'Gleason score' is then used to classify biopsies into five groups - the Gleason Grade Groups - which indicate the risk of dying from prostate cancer. However, this is a subjective process; whether and how a patient is treated may depend on the pathologist who assesses the tissue.
A team of scientists from the Radboud University Medical Center (Nijmegen, The Netherlands) developed an AI system that examines those biopsies the same way a pathologist does. The AI system also determines the Gleason score, and then the system can classify a biopsy according to the Gleason Grade Groups. By means of deep learning, the system examined thousands of images of biopsies to learn what a healthy prostate is, and what more or less aggressive prostate cancer tissue looks like. A semi-automatic labeling technique was used to circumvent the need for manual annotations by pathologists, using pathologists' reports as the reference standard during training. The system was developed to delineate individual glands, assign Gleason growth patterns, and determine the biopsy-level grade.
The investigators collected 5,759 biopsies from 1,243 patients. The developed system achieved a high agreement with the reference standard and scored highly at clinical decision thresholds: benign versus malignant (area under the curve 0.99), grade group of 2 or more (0.978), and grade group of 3 or more (0.974). In an observer experiment, the deep-learning system scored higher (kappa 0.854) than the panel (median kappa 0.819), outperforming 10 of 15 pathologist observers. On the external test dataset, the system obtained a high agreement with the reference standard set independently by two pathologists (quadratic Cohen's kappa 0.723 and 0.707) and within inter-observer variability (kappa 0.71).
The authors concluded that their automated deep-learning system achieved a performance similar to pathologists for Gleason grading and could potentially contribute to prostate cancer diagnosis. The system could potentially assist pathologists by screening biopsies, providing second opinions on grade group, and presenting quantitative measurements of volume percentages. The study was published on January 8, 2020 in the journal The Lancet Oncology.
Related Links:
Radboud University Medical Center
To determine the aggressiveness of the cancer, biopsies are taken from the prostate, which are scored by a pathologist. This 'Gleason score' is then used to classify biopsies into five groups - the Gleason Grade Groups - which indicate the risk of dying from prostate cancer. However, this is a subjective process; whether and how a patient is treated may depend on the pathologist who assesses the tissue.
A team of scientists from the Radboud University Medical Center (Nijmegen, The Netherlands) developed an AI system that examines those biopsies the same way a pathologist does. The AI system also determines the Gleason score, and then the system can classify a biopsy according to the Gleason Grade Groups. By means of deep learning, the system examined thousands of images of biopsies to learn what a healthy prostate is, and what more or less aggressive prostate cancer tissue looks like. A semi-automatic labeling technique was used to circumvent the need for manual annotations by pathologists, using pathologists' reports as the reference standard during training. The system was developed to delineate individual glands, assign Gleason growth patterns, and determine the biopsy-level grade.
The investigators collected 5,759 biopsies from 1,243 patients. The developed system achieved a high agreement with the reference standard and scored highly at clinical decision thresholds: benign versus malignant (area under the curve 0.99), grade group of 2 or more (0.978), and grade group of 3 or more (0.974). In an observer experiment, the deep-learning system scored higher (kappa 0.854) than the panel (median kappa 0.819), outperforming 10 of 15 pathologist observers. On the external test dataset, the system obtained a high agreement with the reference standard set independently by two pathologists (quadratic Cohen's kappa 0.723 and 0.707) and within inter-observer variability (kappa 0.71).
The authors concluded that their automated deep-learning system achieved a performance similar to pathologists for Gleason grading and could potentially contribute to prostate cancer diagnosis. The system could potentially assist pathologists by screening biopsies, providing second opinions on grade group, and presenting quantitative measurements of volume percentages. The study was published on January 8, 2020 in the journal The Lancet Oncology.
Related Links:
Radboud University Medical Center
Latest Pathology News
- Spit Test More Accurate at Identifying Future Prostate Cancer Risk
- DNA Nanotechnology Boosts Sensitivity of Test Strips
- Novel UV and Machine Learning-Aided Method Detects Microbial Contamination in Cell Cultures
- New Error-Corrected Method to Help Detect Cancer from Blood Samples Alone
- "Metal Detector" Algorithm Hunts Down Vulnerable Tumors
- Novel Technique Uses ‘Sugar’ Signatures to Identify and Classify Pancreatic Cancer Cell Subtypes
- Advanced Imaging Reveals Mechanisms Causing Autoimmune Disease
- AI Model Effectively Predicts Patient Outcomes in Common Lung Cancer Type
- AI Model Predicts Patient Response to Bladder Cancer Treatment
- New Laser-Based Method to Accelerate Cancer Diagnosis
- New AI Model Predicts Gene Variants’ Effects on Specific Diseases
- Powerful AI Tool Diagnoses Coeliac Disease from Biopsy Images with Over 97% Accuracy
- Pre-Analytical Conditions Influence Cell-Free MicroRNA Stability in Blood Plasma Samples
- 3D Cell Culture System Could Revolutionize Cancer Diagnostics
- Painless Technique Measures Glucose Concentrations in Solution and Tissue Via Sound Waves
- Skin-Based Test to Improve Diagnosis of Rare, Debilitating Neurodegenerative Disease
Channels
Clinical Chemistry
view channel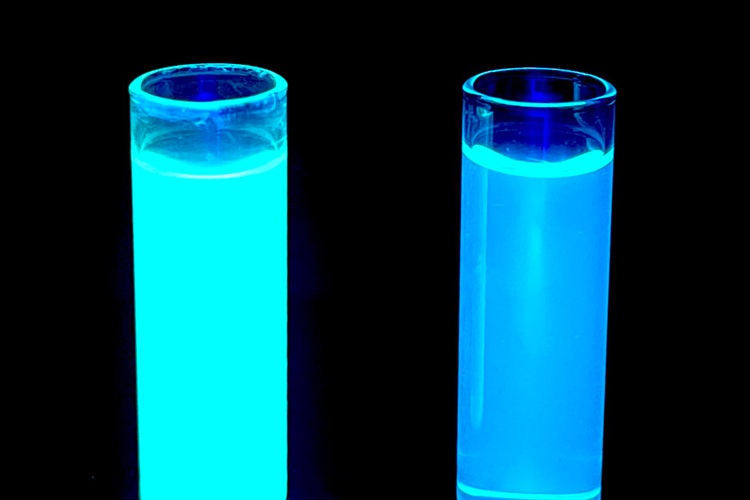
‘Brilliantly Luminous’ Nanoscale Chemical Tool to Improve Disease Detection
Thousands of commercially available glowing molecules known as fluorophores are commonly used in medical imaging, disease detection, biomarker tagging, and chemical analysis. They are also integral in... Read more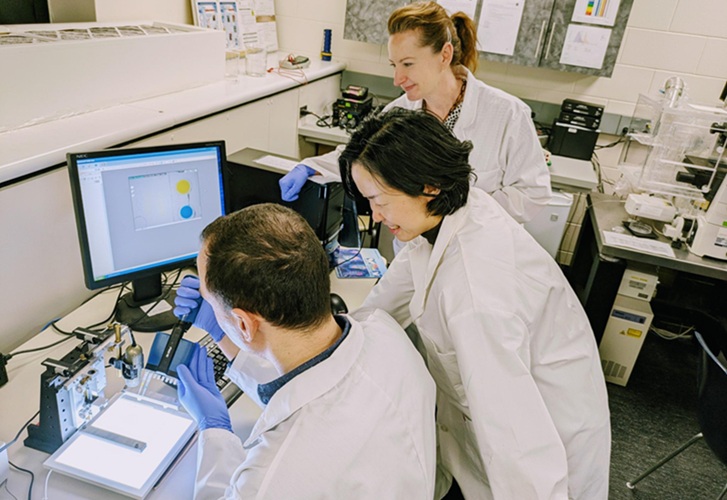
Low-Cost Portable Screening Test to Transform Kidney Disease Detection
Millions of individuals suffer from kidney disease, which often remains undiagnosed until it has reached a critical stage. This silent epidemic not only diminishes the quality of life for those affected... Read more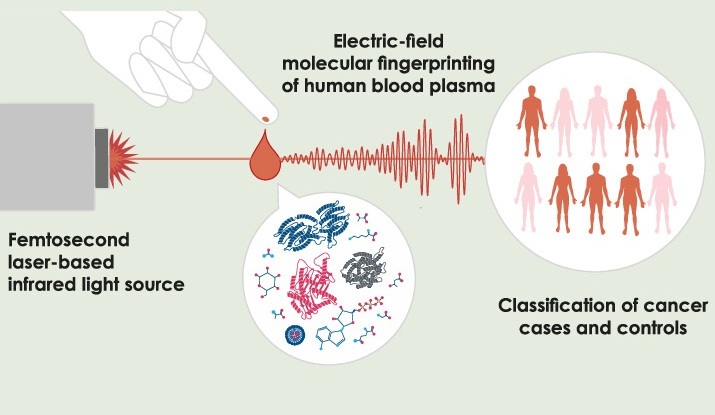
New Method Uses Pulsed Infrared Light to Find Cancer's 'Fingerprints' In Blood Plasma
Cancer diagnoses have traditionally relied on invasive or time-consuming procedures like tissue biopsies. Now, new research published in ACS Central Science introduces a method that utilizes pulsed infrared... Read moreMolecular Diagnostics
view channel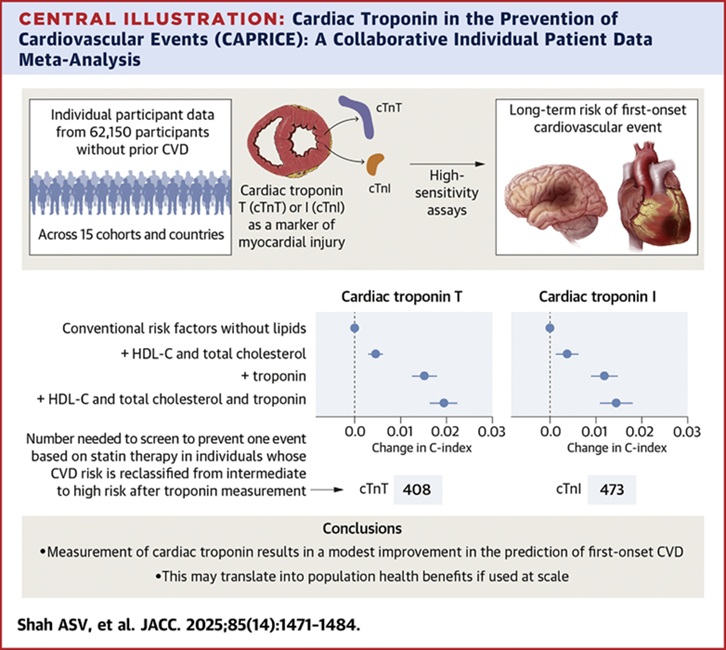
Simple Blood Test Improves Heart Attack and Stroke Risk Prediction
Troponin is a protein found in heart muscle cells that is released into the bloodstream when the heart is damaged. High-sensitivity troponin blood tests are commonly used in hospitals to diagnose heart... Read more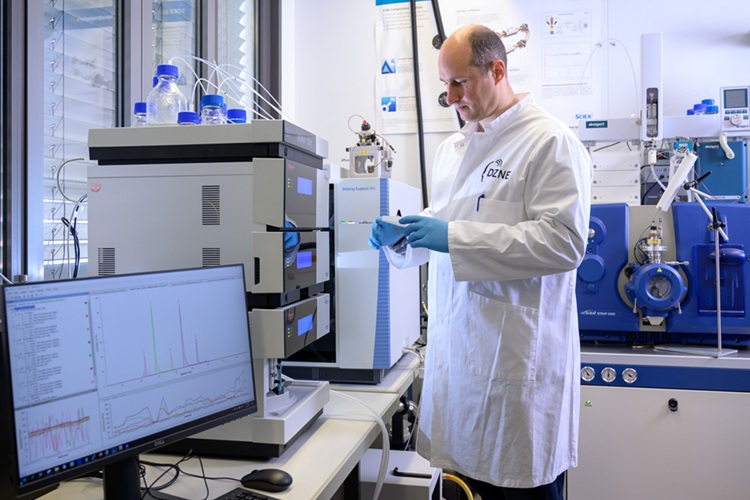
Blood Biomarker Test Could Detect Genetic Predisposition to Alzheimer’s
New medications for Alzheimer’s disease, the most common form of dementia, are now becoming available. These treatments, known as “amyloid antibodies,” work by promoting the removal of small deposits from... Read more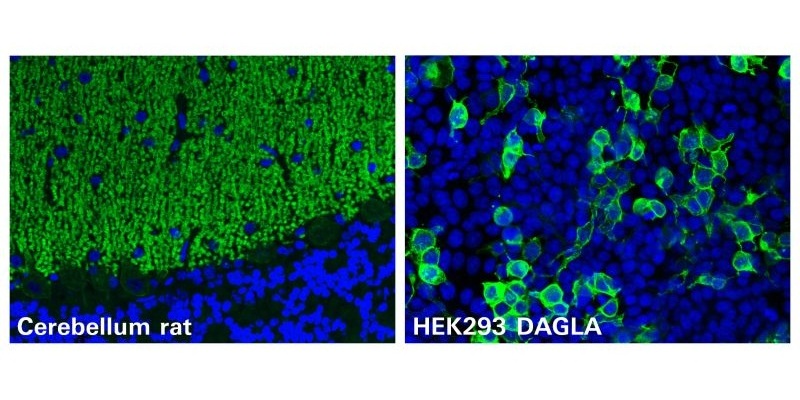
Novel Autoantibody Against DAGLA Discovered in Cerebellitis
Autoimmune cerebellar ataxias are strongly disabling disorders characterized by an impaired ability to coordinate muscle movement. Cerebellar autoantibodies serve as useful biomarkers to support rapid... Read more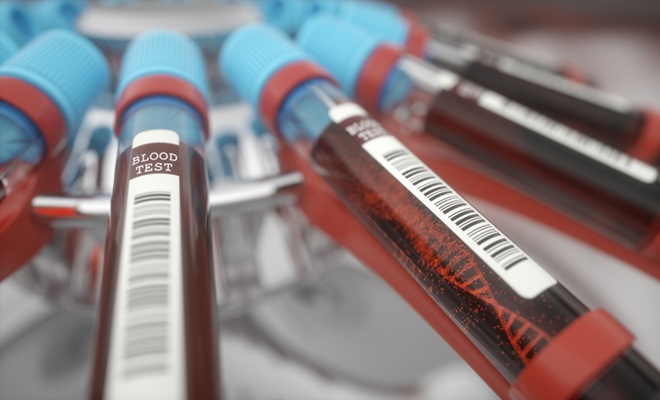
Gene-Based Blood Test Accurately Predicts Tumor Recurrence of Advanced Skin Cancer
Melanoma, an aggressive form of skin cancer, becomes extremely difficult to treat once it spreads to other parts of the body. For patients with metastatic melanoma tumors that cannot be surgically removed... Read moreHematology
view channel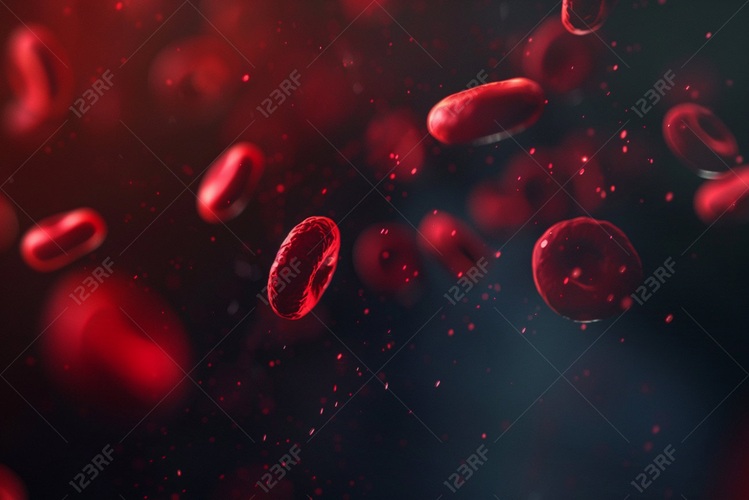
New Scoring System Predicts Risk of Developing Cancer from Common Blood Disorder
Clonal cytopenia of undetermined significance (CCUS) is a blood disorder commonly found in older adults, characterized by mutations in blood cells and a low blood count, but without any obvious cause or... Read more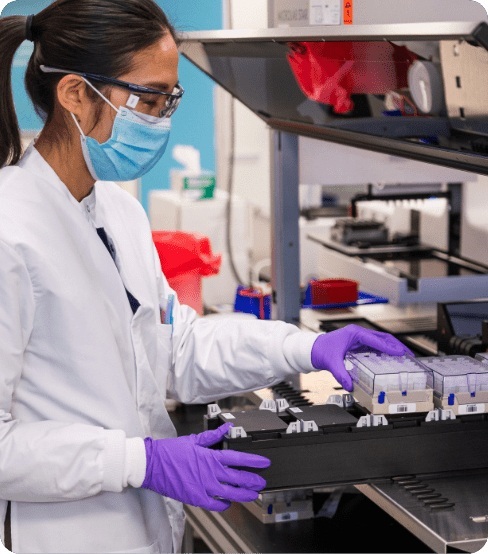
Non-Invasive Prenatal Test for Fetal RhD Status Demonstrates 100% Accuracy
In the United States, approximately 15% of pregnant individuals are RhD-negative. However, in about 40% of these cases, the fetus is also RhD-negative, making the administration of RhoGAM unnecessary.... Read moreImmunology
view channel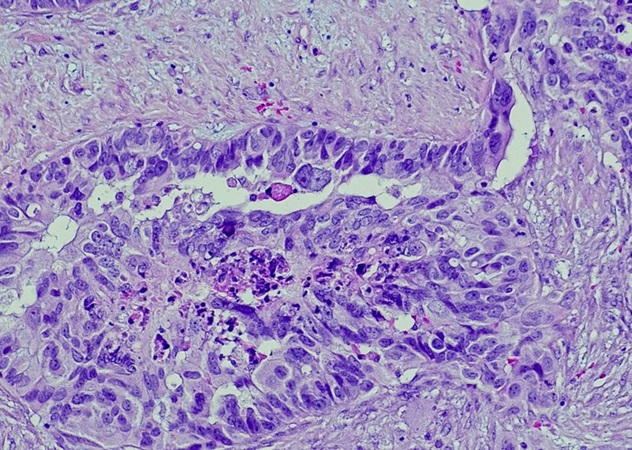
Stem Cell Test Predicts Treatment Outcome for Patients with Platinum-Resistant Ovarian Cancer
Epithelial ovarian cancer frequently responds to chemotherapy initially, but eventually, the tumor develops resistance to the therapy, leading to regrowth. This resistance is partially due to the activation... Read more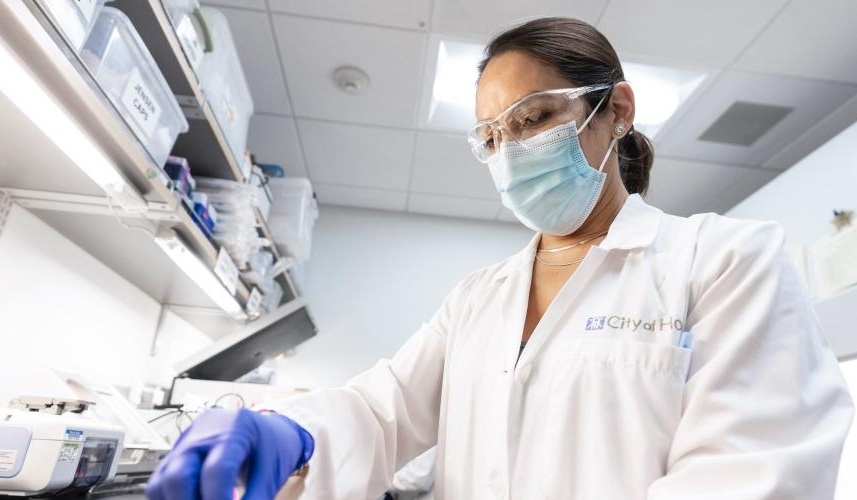
Machine Learning-Enabled Blood Test Predicts Immunotherapy Response in Lymphoma Patients
Chimeric antigen receptor (CAR) T-cell therapy has emerged as one of the most promising recent developments in the treatment of blood cancers. However, over half of non-Hodgkin lymphoma (NHL) patients... Read moreMicrobiology
view channel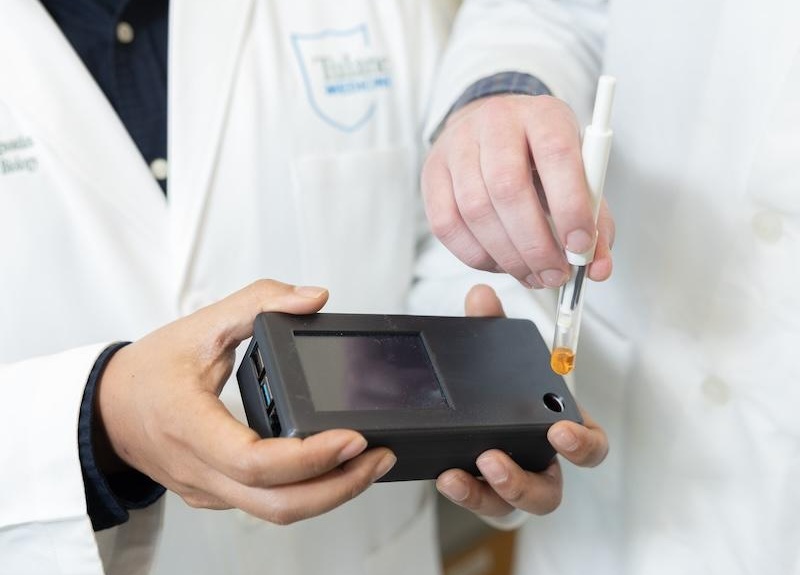
Handheld Device Delivers Low-Cost TB Results in Less Than One Hour
Tuberculosis (TB) remains the deadliest infectious disease globally, affecting an estimated 10 million people annually. In 2021, about 4.2 million TB cases went undiagnosed or unreported, mainly due to... Read more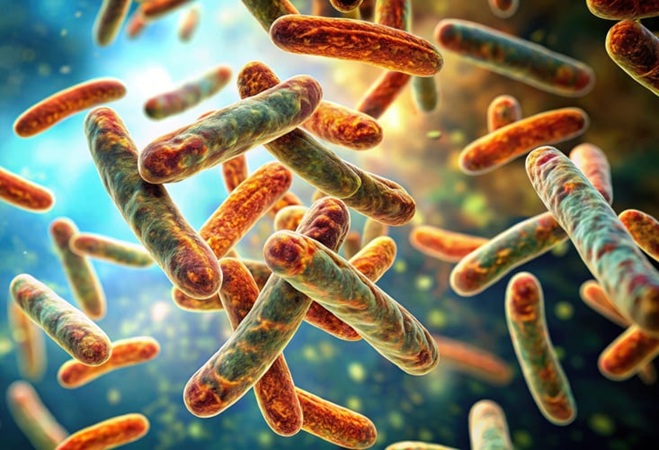
New AI-Based Method Improves Diagnosis of Drug-Resistant Infections
Drug-resistant infections, particularly those caused by deadly bacteria like tuberculosis and staphylococcus, are rapidly emerging as a global health emergency. These infections are more difficult to treat,... Read more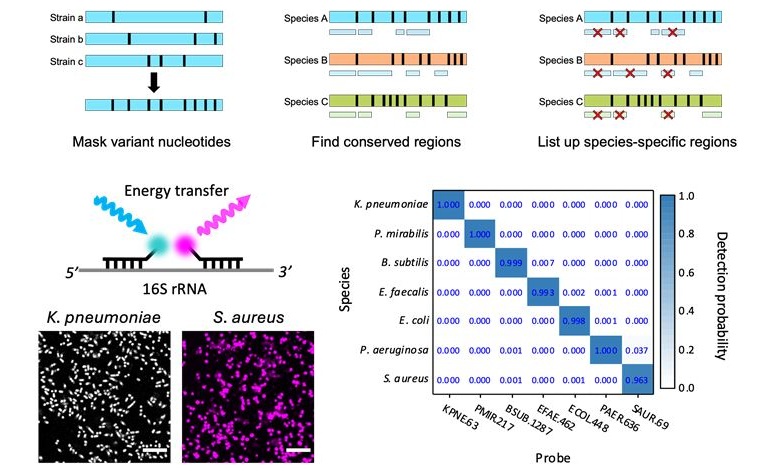
Breakthrough Diagnostic Technology Identifies Bacterial Infections with Almost 100% Accuracy within Three Hours
Rapid and precise identification of pathogenic microbes in patient samples is essential for the effective treatment of acute infectious diseases, such as sepsis. The fluorescence in situ hybridization... Read moreTechnology
view channel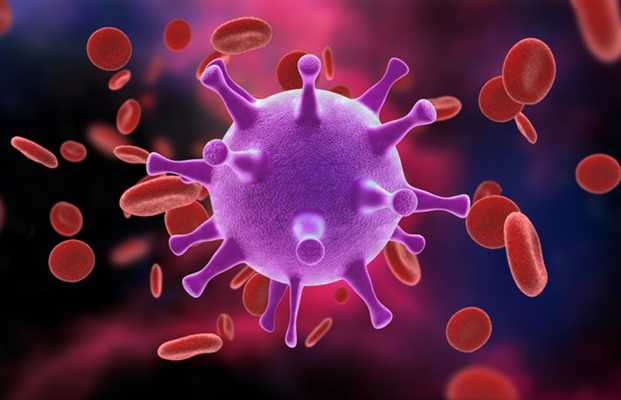
Disposable Microchip Technology Could Selectively Detect HIV in Whole Blood Samples
As of the end of 2023, approximately 40 million people globally were living with HIV, and around 630,000 individuals died from AIDS-related illnesses that same year. Despite a substantial decline in deaths... Read more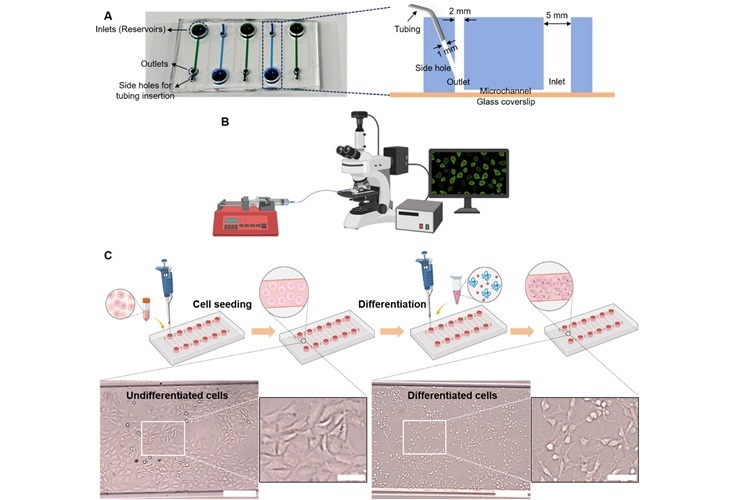
Pain-On-A-Chip Microfluidic Device Determines Types of Chronic Pain from Blood Samples
Chronic pain is a widespread condition that remains difficult to manage, and existing clinical methods for its treatment rely largely on self-reporting, which can be subjective and especially problematic... Read more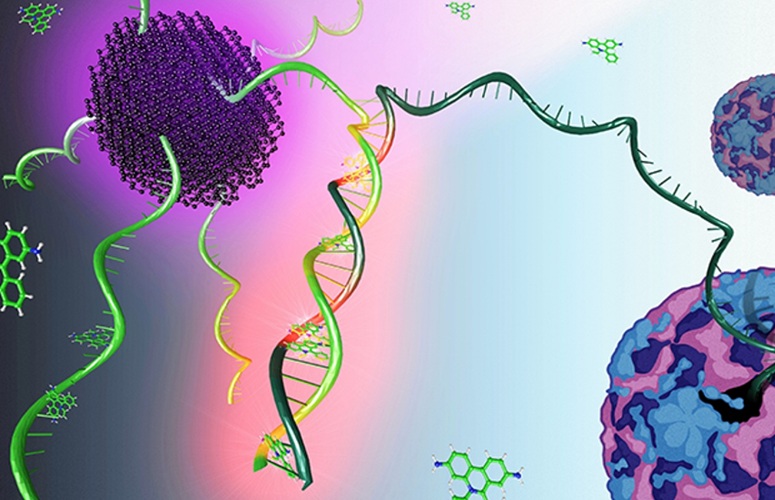
Innovative, Label-Free Ratiometric Fluorosensor Enables More Sensitive Viral RNA Detection
Viruses present a major global health risk, as demonstrated by recent pandemics, making early detection and identification essential for preventing new outbreaks. While traditional detection methods are... Read moreIndustry
view channel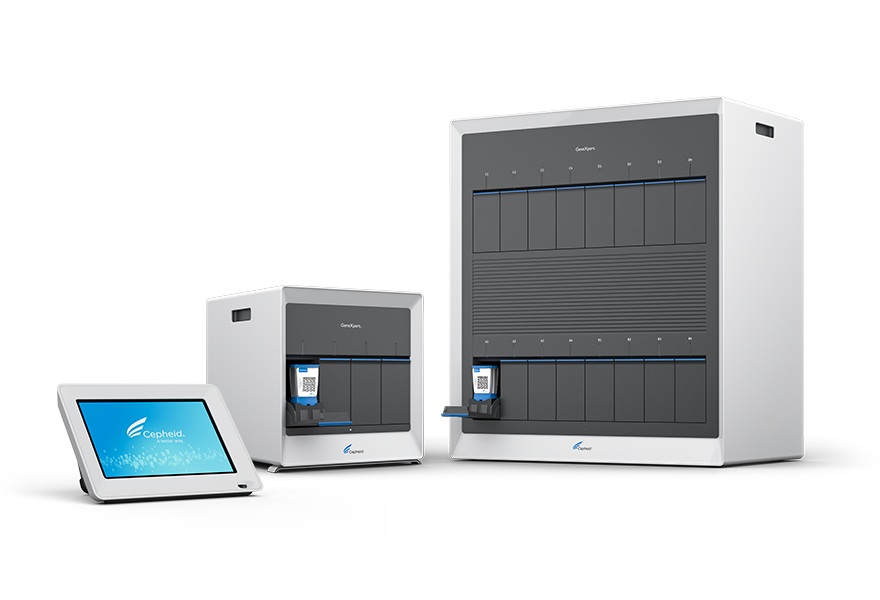
Cepheid and Oxford Nanopore Technologies Partner on Advancing Automated Sequencing-Based Solutions
Cepheid (Sunnyvale, CA, USA), a leading molecular diagnostics company, and Oxford Nanopore Technologies (Oxford, UK), the company behind a new generation of sequencing-based molecular analysis technologies,... Read more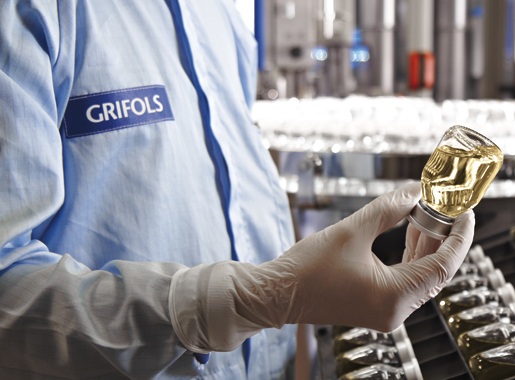
Grifols and Tecan’s IBL Collaborate on Advanced Biomarker Panels
Grifols (Barcelona, Spain), one of the world’s leading producers of plasma-derived medicines and innovative diagnostic solutions, is expanding its offer in clinical diagnostics through a strategic partnership... Read more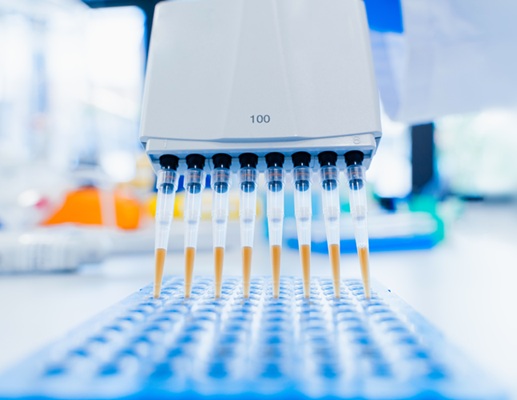