AI Approximates Human Performance in Breast Cancer Diagnosis
By LabMedica International staff writers
Posted on 25 Jul 2016
Artificial intelligence (AI) methods that train computers to interpret pathology images could make pathologic diagnoses more accurate.Posted on 25 Jul 2016
Researchers at Beth Israel Deaconess Medical Center (BIDMC; Boston, MA, USA) and Harvard Medical School (HMS; Boston, MA, USA) have developed a machine-learning algorithm that can be used for a range of applications, including speech and image recognition. The algorithm teaches machines to interpret complex patterns and structures observed in real-life data by building multi-layer artificial neural networks, in a process which is thought to show similarities with the learning process occurring in the neurons of the neocortex, the region where thinking occurs.
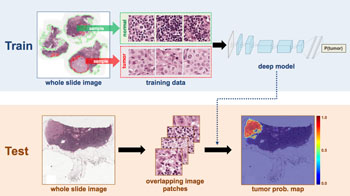
Image: The framework of cancer metastases detection (Photo courtesy of Andrew Beck / BIDMC).
The researchers trained the computer to distinguish between cancerous tumor regions and normal regions, based on a deep multilayer convolutional network that began with hundreds of training slides for which a pathologist has labeled regions of cancer and regions of normal cells. They then extracted millions of small training examples and used deep learning to build a computational model to classify them. The researchers then identified the specific training examples for which the computer was prone to making mistakes, and re-trained it using greater numbers of the more difficult training examples, gradually improving the computer’s performance.
The computers performance was tested in a competition at the 2016 International Symposium of Biomedical Imaging (ISBI), held during April in Prague (Czech Republic). The competition involved examining images of lymph nodes to decide whether or not they contained breast cancer. The computer algorithm identified correctly 92% percent of the time, nearly matching the 96% success rate of a human pathologist. The algorithm placed first in two separate categories, competing against private companies and academic research institutions from around the world. A technical report describing the approach was posted on June 18, 2016, on the arXiv.org repository.
“Peering into the microscope to sift through millions of normal cells to identify just a few malignant cells can prove extremely laborious using conventional methods. We thought this was a task that the computer could be quite good at,” said pathologist Andrew Beck, MD, PhD, director of bioinformatics at the Cancer Research Institute at BIDMC. “But the truly exciting thing was when we combined the pathologist’s analysis with our automated computational diagnostic method, the result improved to 99.5% accuracy. Combining these two methods yielded a major reduction in errors.”
“When we started this challenge, we expected some interesting results. The fact that computers had almost comparable performance to humans is way beyond what I had anticipated,” said Jeroen van der Laak, PhD, of Radboud University Medical Center (The Netherlands), an organizer for the competition. “It is a clear indication that artificial intelligence is going to shape the way we deal with histopathological images in the years to come.”
Related Links:
Beth Israel Deaconess Medical Center
Harvard Medical School