Machine Learning Approach Detects Cancer by Analyzing DNA in Blood Samples
By LabMedica International staff writers
Posted on 10 Jun 2019
Researchers have described a proof-of-principle approach for the screening, early detection, and monitoring of human cancer based on a machine learning approach that evaluates fragmentation patterns of cell-free DNA across the genome.Posted on 10 Jun 2019
While cell-free DNA in the blood provides a non-invasive diagnostic avenue for patients with cancer, characteristics of the origins and molecular features of cell-free DNA are poorly understood. To correct this lack, investigators at Johns Hopkins University (Baltimore, MD, USA) developed a machine learning-based approach to identify abnormal patterns of DNA fragments in the blood of patients with cancer.
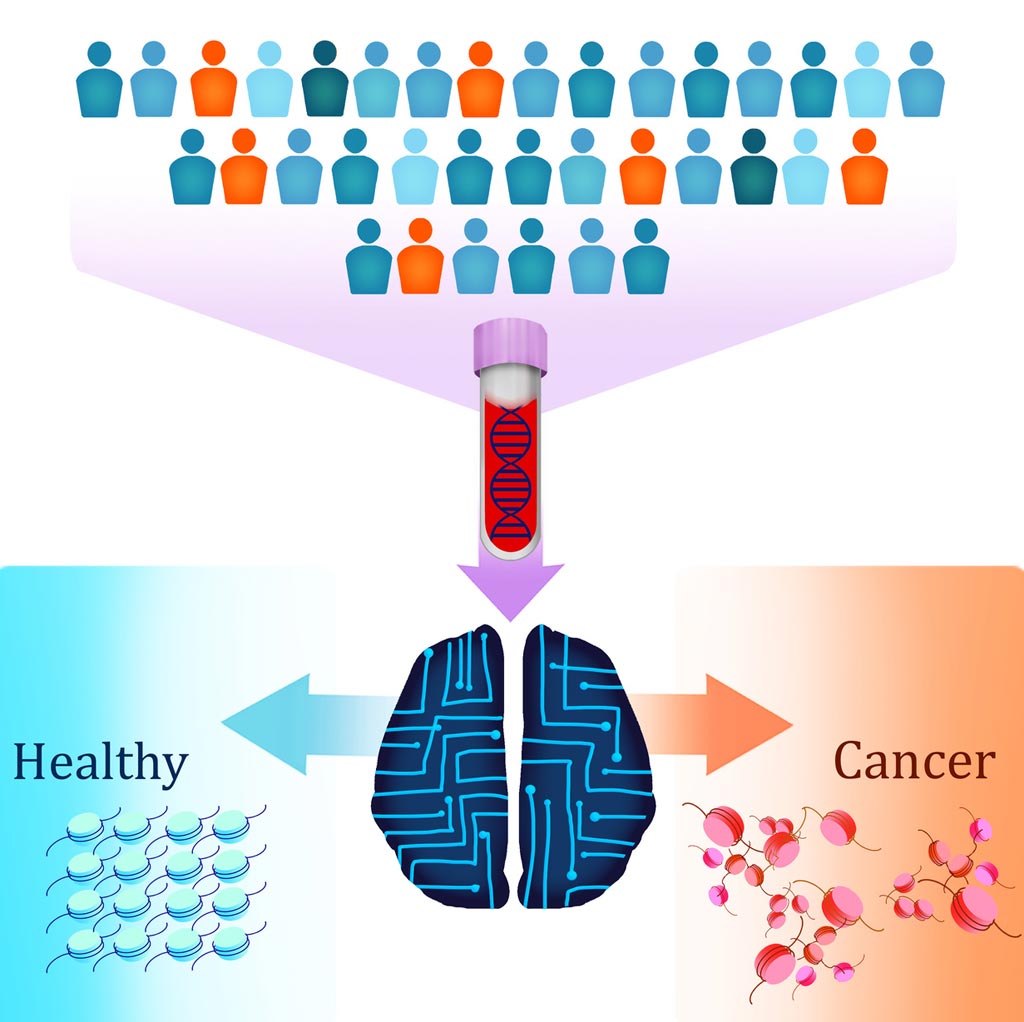
Image: A new liquid biopsy test called DELFI (DNA evaluation of fragments for early interception) uses artificial intelligence to detect patients with cancer by identifying altered DNA fragmentation in the blood (Photo courtesy of Carolyn Hruban, Johns Hopkins University).
They used this DELFI (DNA evaluation of fragments for early interception) method to analyze the fragmentation profiles of 236 patients with breast, colorectal, lung, ovarian, pancreatic, gastric, or bile duct cancer and 245 healthy individuals.
The machine-learning model incorporated genome-wide fragmentation features with sensitivities of detection ranging from 57% to more than 99% among the seven cancer types at 98% specificity. Fragmentation profiles could be used to identify the tissue of origin of the cancers to a limited number of sites in 75% of cases. Combining this approach with mutation-based cell-free DNA analyses detected 91% of patients with cancer.
"For various reasons, a cancer genome is disorganized in the way it is packaged, which means that when cancer cells die they release their DNA in a chaotic manner into the bloodstream," said first author Dr. Jillian Phallen, a postdoctoral research fellow at Johns Hopkins University. "By examining this cell-free DNA (cfDNA), DELFI helps identify the presence of cancer by detecting abnormalities in the size and amount of DNA in different regions of the genome based on how it is packaged."
"We are encouraged about the potential of DELFI because it looks at a completely independent set of cell-free DNA characteristics from those that have posed difficulties over the years, and we look forward to working with our collaborators worldwide to make this test available to patients," said senior author Dr. Victor E. Velculescu, professor of oncology at Johns Hopkins University.
The DELFI method was described in the May 29, 2019, online edition of the journal Nature.
Related Links:
Johns Hopkins University