AACC Competition Demonstrates How Labs Can Use Data Analytics to Solve Real Problems
By LabMedica International staff writers Posted on 17 Oct 2022 |
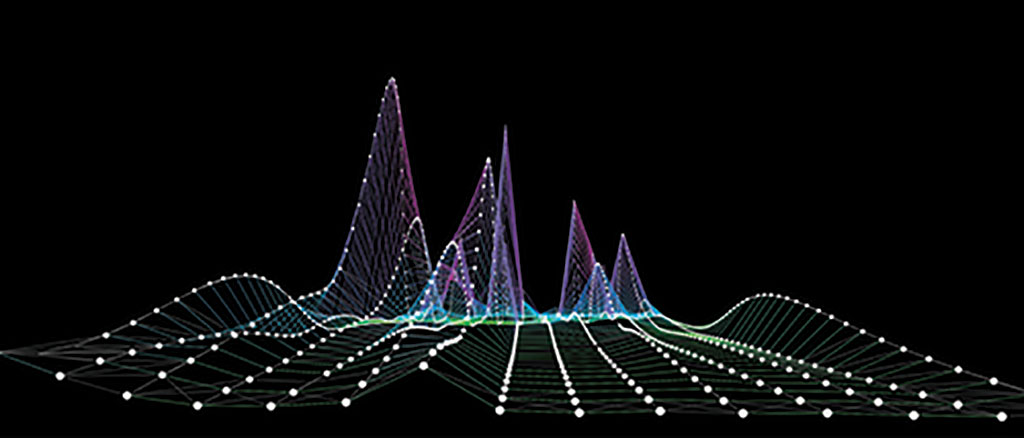
Clinicians rely on parathyroid hormone-related peptide (PTHrP) measurement to help establish a diagnosis of humoral hypercalcemia of malignancy - a rare form of cancer that causes, among other things, high levels of calcium in the blood. The problem: Clinicians often order it for patients with low pretest probability. Excessive PTHrP testing can lead to expensive, unnecessary, and potentially harmful procedures, including invasive laboratory testing to locate a possibly nonexistent cancerous tumor. A successful predictive algorithm would help laboratorians quickly and accurately identify potentially inappropriate PTHrP test orders by predicting whether laboratory data available at the time of order already suggest an abnormal PTHrP result. A machine-learning challenge introduced for the first time by the American Association for Clinical Chemistry (Washington, DC, USA; www.aacc.org) at the 2022 AACC Annual Scientific Meeting & Clinical Lab Expo demonstrated how laboratories can use data analytics to solve these real problems facing patients and clinicians.
The Predicting PTHrP Results Competition introduced by the AACC at the event in association with the informatics section in the department of pathology and immunology of Washington University School of Medicine, St. Louis (WUSM, St. Louis, MI, USA) aimed to engage the community of laboratory medicine practitioners in a fun and friendly online environment where they could practice their data analytics skills, learn from each other, and see how others approach problems on the data-driven side of laboratory medicine. Competition participants formed teams and used securely shared real, de-identified clinical data from PTHrP orders at WUSM to build their predictive algorithms. This is termed the “practice dataset”. Using real clinical data was a big deal because most machine-learning competitions use synthesized datasets. Organizers set up the competition using Kaggle, a popular online platform for machine-learning modeling and contests, and selected F1 score (the harmonic mean of sensitivity and specificity) as the performance metric.
A major challenge for the teams was developing a predictive model that achieved high accuracy without overfitting it to the public dataset (the practice dataset). Overfitting would mean the algorithm worked well on the initial data but failed if applied to new data and was not generalizable. Organizers used a second, private dataset to judge the algorithm’s effectiveness. From May to June 2022, 24 teams ran a total of 395 iterations of their predictive models through the public dataset. Each time a team submitted a predictive model for an attempt, they used the resulting F1 score to improve - or “train” - the model. For the final attempt, each team ran their predictive model through the private dataset. The winning team, Team Kagglist, achieved an F1 score of 0.9 with their predictive model. For reference, WUSM’s manual approach for identifying patients at risk for PTHrP had an F1 score of 0.6, making the algorithm a significant improvement over standard practice.
“We shouldn’t expect a predictive model trained on data from one hospital to automatically work at other hospitals,” said Team Kaggle’s Yingheng Wang. “Ultimately, we should aim to create adaptive models that can be fine-tuned by other institutions for their specific populations.”
“The quality of all 24 models was excellent and showed a high degree of accuracy for the very difficult task we challenged participants with,” said competition organizer Mark Zaydman, MD, PhD, an assistant professor of pathology and immunology at WUSM. “This competition really showed our community is ready to engage with sophisticated machine learning and data analytics tools.”
Related Links:
AACC
Latest Industry News
- Danaher and Johns Hopkins University Collaborate to Improve Neurological Diagnosis
- Beckman Coulter and MeMed Expand Host Immune Response Diagnostics Partnership
- Thermo Fisher and Bio-Techne Enter Into Strategic Distribution Agreement for Europe
- ECCMID Congress Name Changes to ESCMID Global
- Bosch and Randox Partner to Make Strategic Investment in Vivalytic Analysis Platform
- Siemens to Close Fast Track Diagnostics Business
- Beckman Coulter and Fujirebio Expand Partnership on Neurodegenerative Disease Diagnostics
- Sysmex and Hitachi Collaborate on Development of New Genetic Testing Systems
- Sysmex and CellaVision Expand Collaboration to Advance Hematology Solutions
- BD and Techcyte Collaborate on AI-Based Digital Cervical Cytology System for Pap Testing
- Medlab Middle East 2024 to Address Transformative Potential of Artificial Intelligence
- Seegene and Microsoft Collaborate to Realize a World Free from All Diseases and Future Pandemics
- Medlab Middle East 2024 to Highlight Importance of Sustainability in Laboratories
- Fujirebio and Agappe Collaborate on CLIA-Based Immunoassay
- Medlab Middle East 2024 to Highlight Groundbreaking NextGen Medicine
- bioMérieux Acquires Software Company LUMED to Support Fight against Antimicrobial Resistance
Channels
Clinical Chemistry
view channel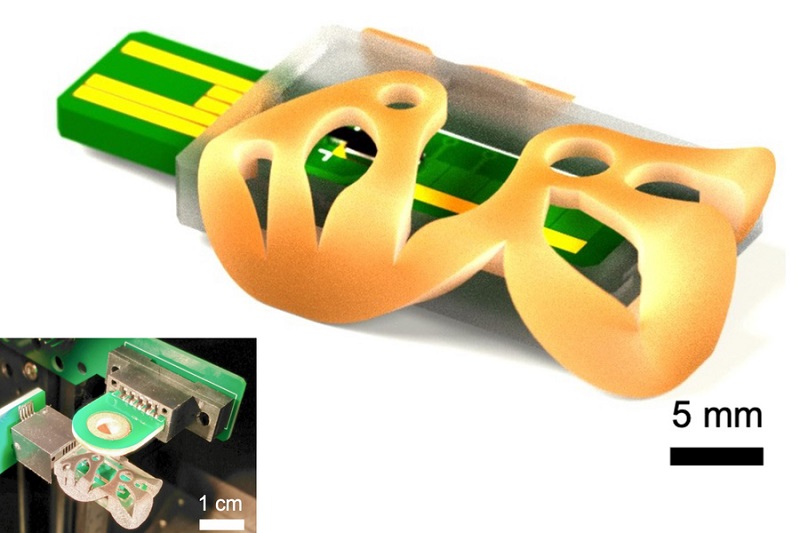
3D Printed Point-Of-Care Mass Spectrometer Outperforms State-Of-The-Art Models
Mass spectrometry is a precise technique for identifying the chemical components of a sample and has significant potential for monitoring chronic illness health states, such as measuring hormone levels... Read more.jpg)
POC Biomedical Test Spins Water Droplet Using Sound Waves for Cancer Detection
Exosomes, tiny cellular bioparticles carrying a specific set of proteins, lipids, and genetic materials, play a crucial role in cell communication and hold promise for non-invasive diagnostics.... Read more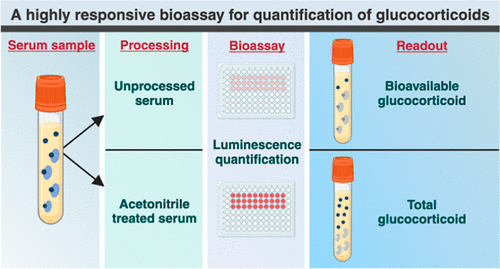
Highly Reliable Cell-Based Assay Enables Accurate Diagnosis of Endocrine Diseases
The conventional methods for measuring free cortisol, the body's stress hormone, from blood or saliva are quite demanding and require sample processing. The most common method, therefore, involves collecting... Read moreMolecular Diagnostics
view channel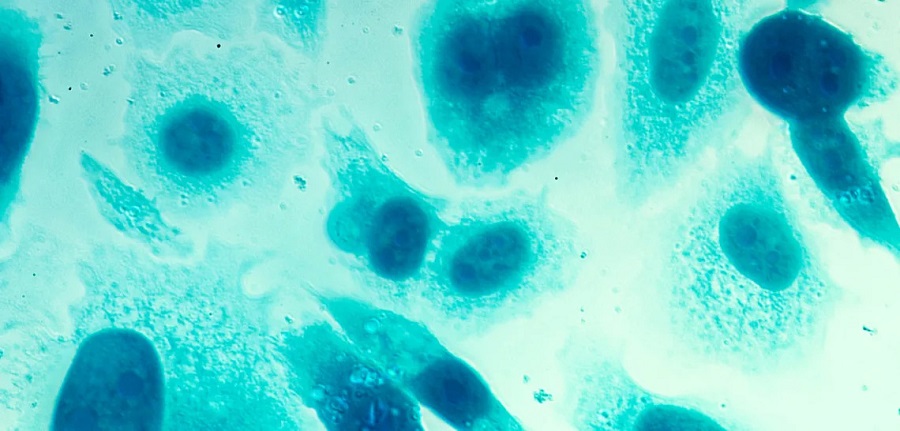
Blood Proteins Could Warn of Cancer Seven Years before Diagnosis
Two studies have identified proteins in the blood that could potentially alert individuals to the presence of cancer more than seven years before the disease is clinically diagnosed. Researchers found... Read more (1)_1.jpg)
Ultrasound-Aided Blood Testing Detects Cancer Biomarkers from Cells
Ultrasound imaging serves as a noninvasive method to locate and monitor cancerous tumors effectively. However, crucial details about the cancer, such as the specific types of cells and genetic mutations... Read moreHematology
view channel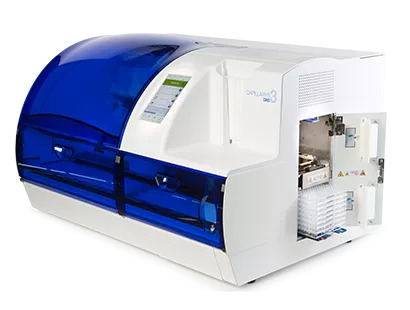
Next Generation Instrument Screens for Hemoglobin Disorders in Newborns
Hemoglobinopathies, the most widespread inherited conditions globally, affect about 7% of the population as carriers, with 2.7% of newborns being born with these conditions. The spectrum of clinical manifestations... Read more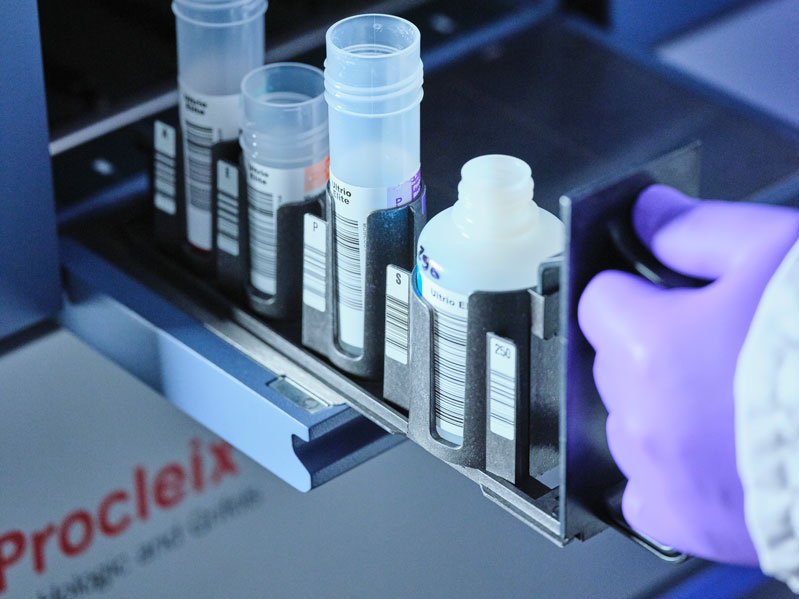
First 4-in-1 Nucleic Acid Test for Arbovirus Screening to Reduce Risk of Transfusion-Transmitted Infections
Arboviruses represent an emerging global health threat, exacerbated by climate change and increased international travel that is facilitating their spread across new regions. Chikungunya, dengue, West... Read more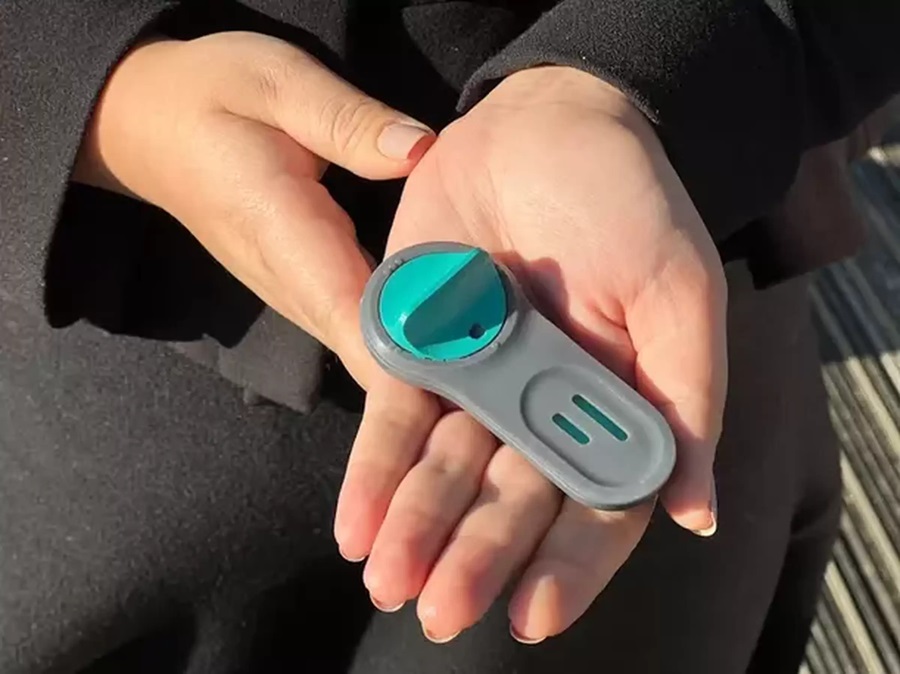
POC Finger-Prick Blood Test Determines Risk of Neutropenic Sepsis in Patients Undergoing Chemotherapy
Neutropenia, a decrease in neutrophils (a type of white blood cell crucial for fighting infections), is a frequent side effect of certain cancer treatments. This condition elevates the risk of infections,... Read more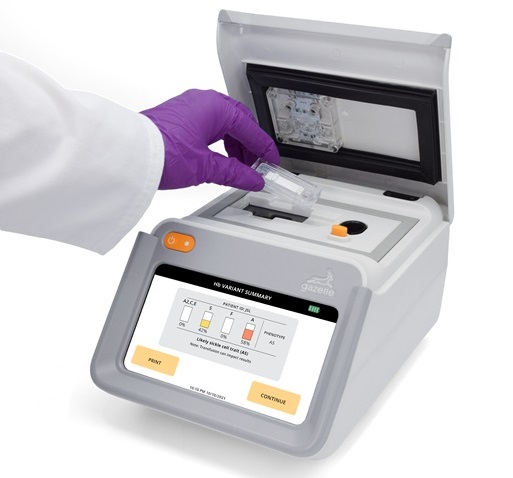
First Affordable and Rapid Test for Beta Thalassemia Demonstrates 99% Diagnostic Accuracy
Hemoglobin disorders rank as some of the most prevalent monogenic diseases globally. Among various hemoglobin disorders, beta thalassemia, a hereditary blood disorder, affects about 1.5% of the world's... Read moreImmunology
view channel.jpg)
AI Predicts Tumor-Killing Cells with High Accuracy
Cellular immunotherapy involves extracting immune cells from a patient's tumor, potentially enhancing their cancer-fighting capabilities through engineering, and then expanding and reintroducing them into the body.... Read more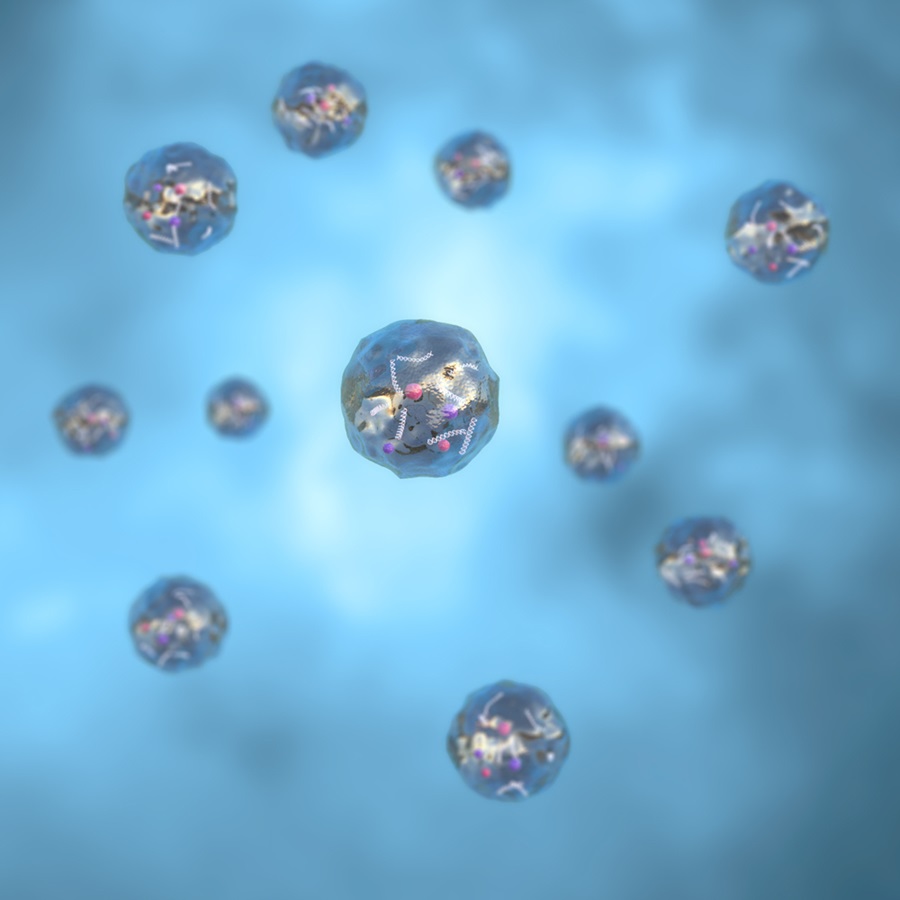
Diagnostic Blood Test for Cellular Rejection after Organ Transplant Could Replace Surgical Biopsies
Transplanted organs constantly face the risk of being rejected by the recipient's immune system which differentiates self from non-self using T cells and B cells. T cells are commonly associated with acute... Read more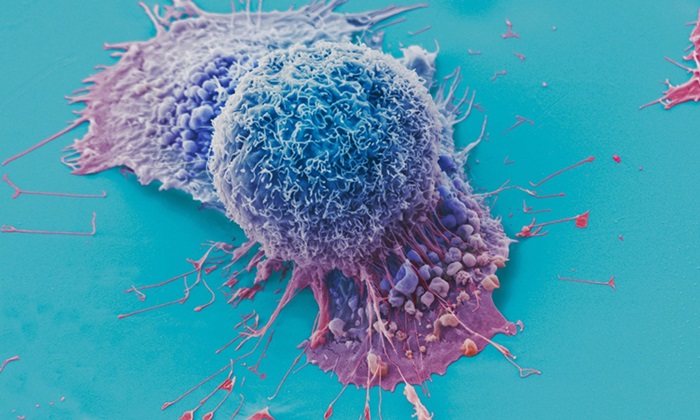
AI Tool Precisely Matches Cancer Drugs to Patients Using Information from Each Tumor Cell
Current strategies for matching cancer patients with specific treatments often depend on bulk sequencing of tumor DNA and RNA, which provides an average profile from all cells within a tumor sample.... Read more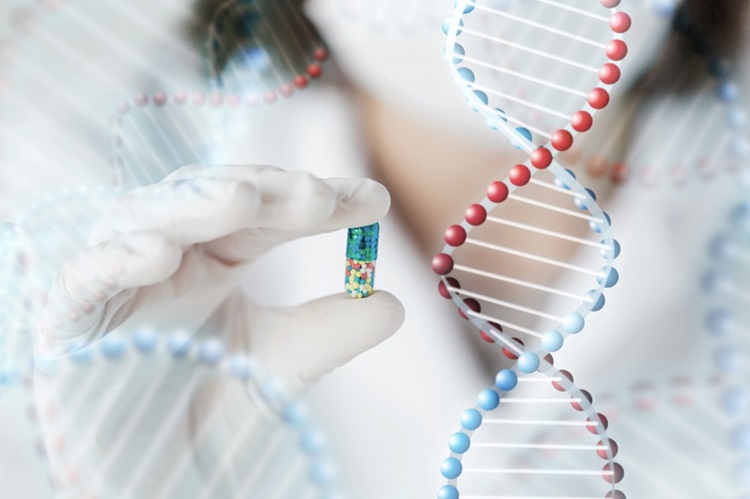
Genetic Testing Combined With Personalized Drug Screening On Tumor Samples to Revolutionize Cancer Treatment
Cancer treatment typically adheres to a standard of care—established, statistically validated regimens that are effective for the majority of patients. However, the disease’s inherent variability means... Read moreMicrobiology
view channel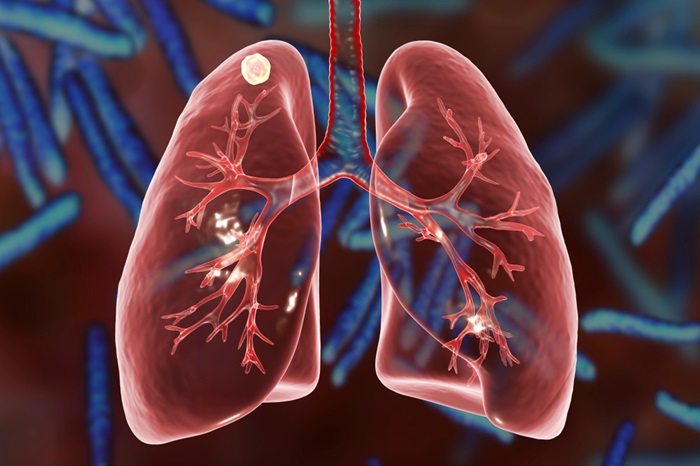
Integrated Solution Ushers New Era of Automated Tuberculosis Testing
Tuberculosis (TB) is responsible for 1.3 million deaths every year, positioning it as one of the top killers globally due to a single infectious agent. In 2022, around 10.6 million people were diagnosed... Read more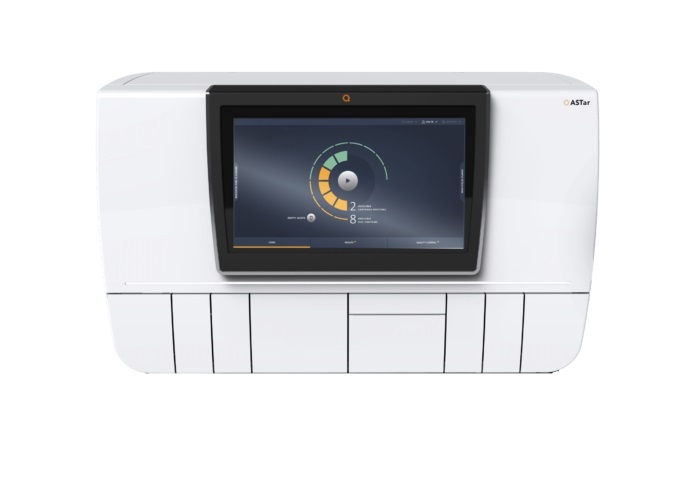
Automated Sepsis Test System Enables Rapid Diagnosis for Patients with Severe Bloodstream Infections
Sepsis affects up to 50 million people globally each year, with bacteraemia, formerly known as blood poisoning, being a major cause. In the United States alone, approximately two million individuals are... Read more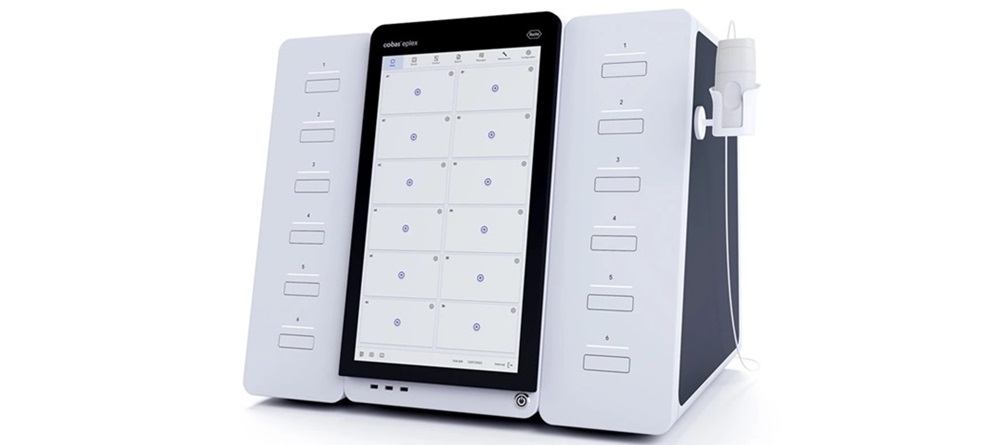
Enhanced Rapid Syndromic Molecular Diagnostic Solution Detects Broad Range of Infectious Diseases
GenMark Diagnostics (Carlsbad, CA, USA), a member of the Roche Group (Basel, Switzerland), has rebranded its ePlex® system as the cobas eplex system. This rebranding under the globally renowned cobas name... Read more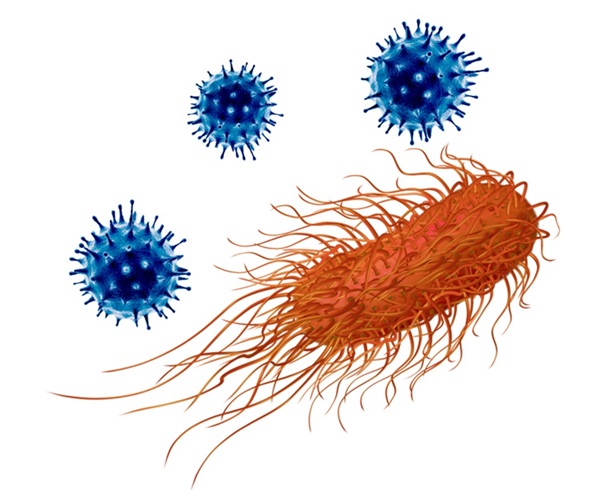
Clinical Decision Support Software a Game-Changer in Antimicrobial Resistance Battle
Antimicrobial resistance (AMR) is a serious global public health concern that claims millions of lives every year. It primarily results from the inappropriate and excessive use of antibiotics, which reduces... Read morePathology
view channel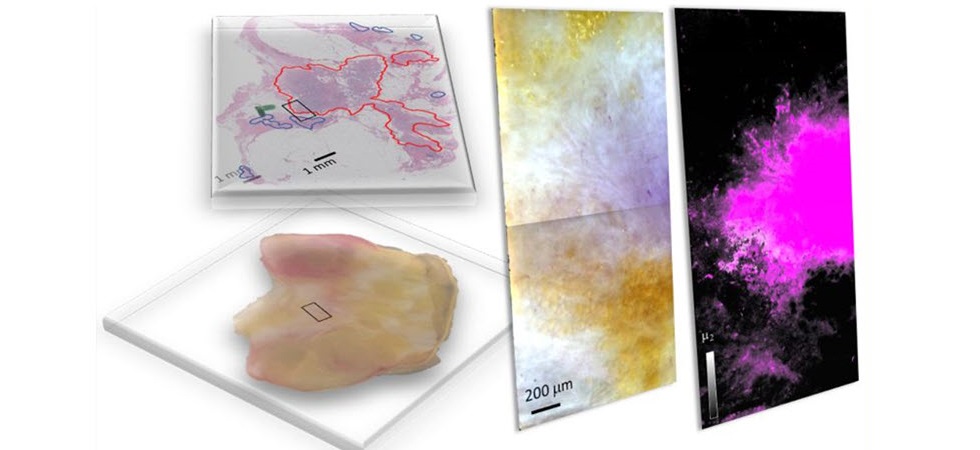
Hyperspectral Dark-Field Microscopy Enables Rapid and Accurate Identification of Cancerous Tissues
Breast cancer remains a major cause of cancer-related mortality among women. Breast-conserving surgery (BCS), also known as lumpectomy, is the removal of the cancerous lump and a small margin of surrounding tissue.... Read more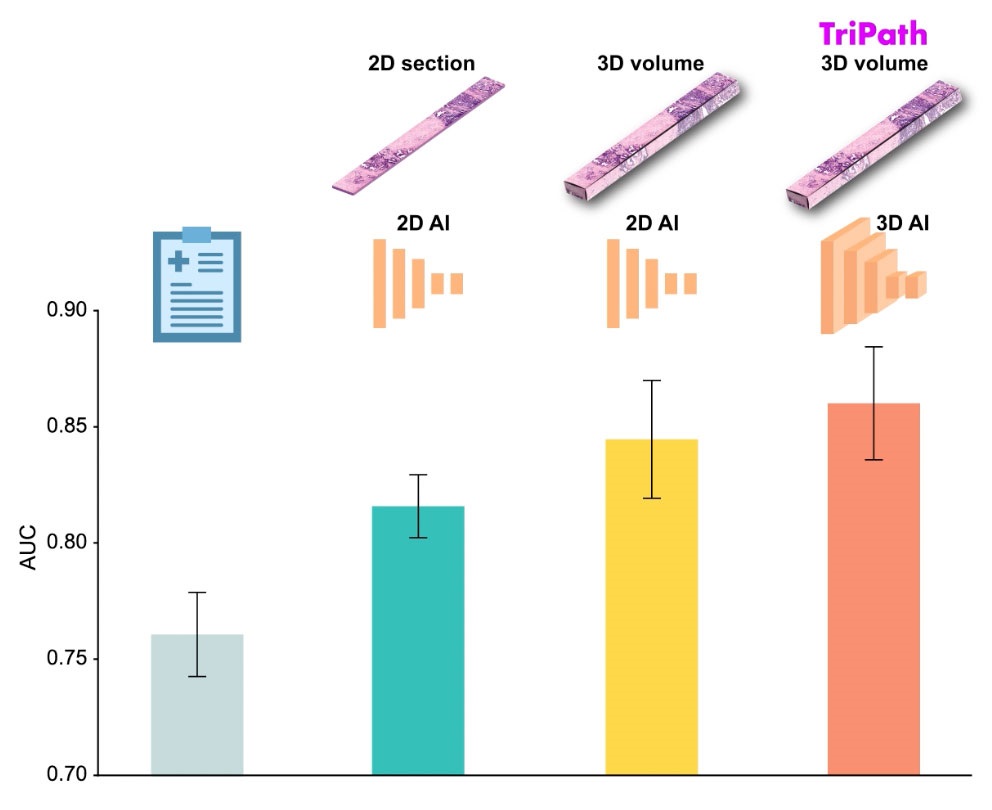
AI Advancements Enable Leap into 3D Pathology
Human tissue is complex, intricate, and naturally three-dimensional. However, the thin two-dimensional tissue slices commonly used by pathologists to diagnose diseases provide only a limited view of the... Read more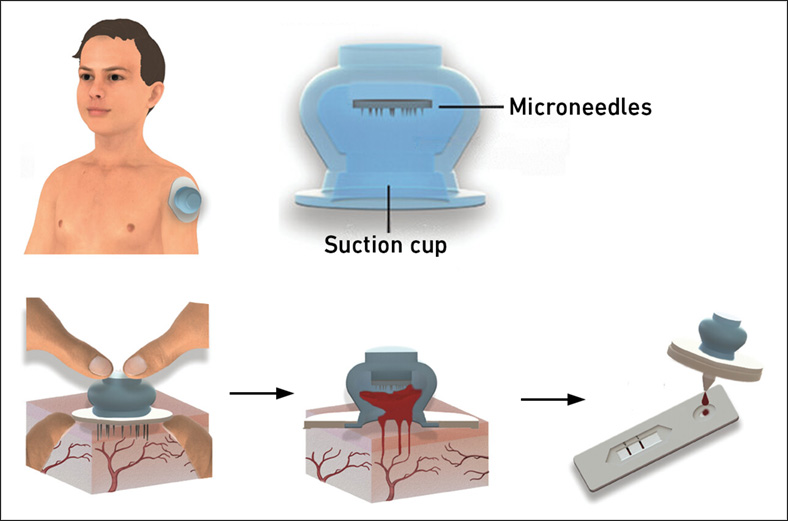
New Blood Test Device Modeled on Leeches to Help Diagnose Malaria
Many individuals have a fear of needles, making the experience of having blood drawn from their arm particularly distressing. An alternative method involves taking blood from the fingertip or earlobe,... Read more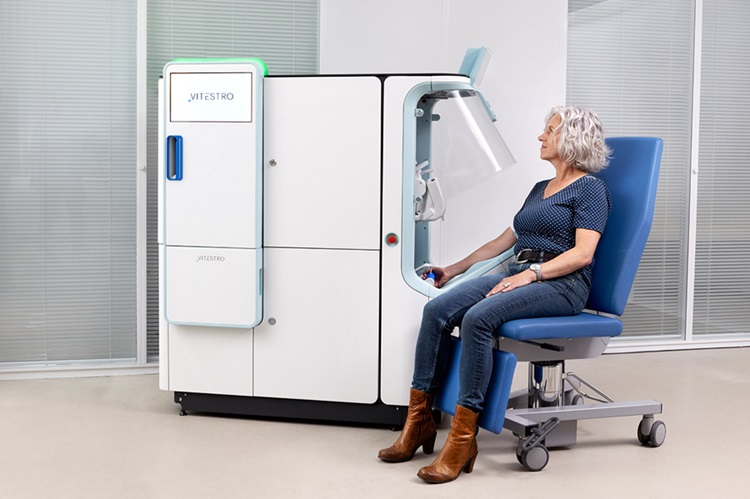
Robotic Blood Drawing Device to Revolutionize Sample Collection for Diagnostic Testing
Blood drawing is performed billions of times each year worldwide, playing a critical role in diagnostic procedures. Despite its importance, clinical laboratories are dealing with significant staff shortages,... Read moreTechnology
view channel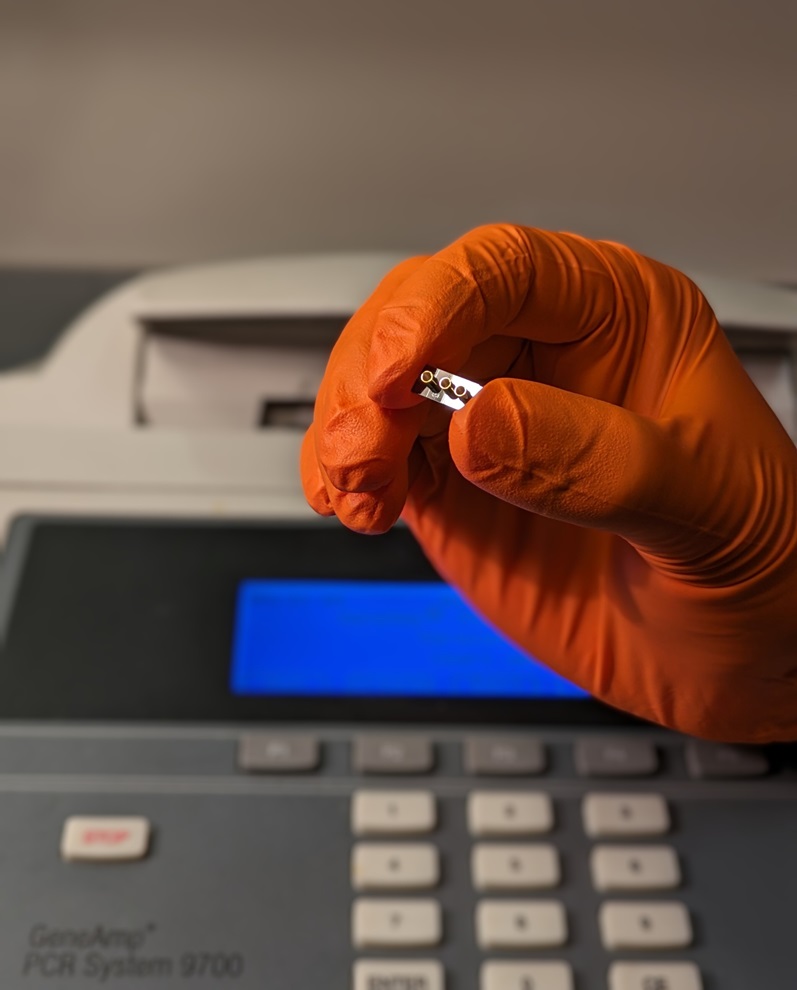
New Diagnostic System Achieves PCR Testing Accuracy
While PCR tests are the gold standard of accuracy for virology testing, they come with limitations such as complexity, the need for skilled lab operators, and longer result times. They also require complex... Read more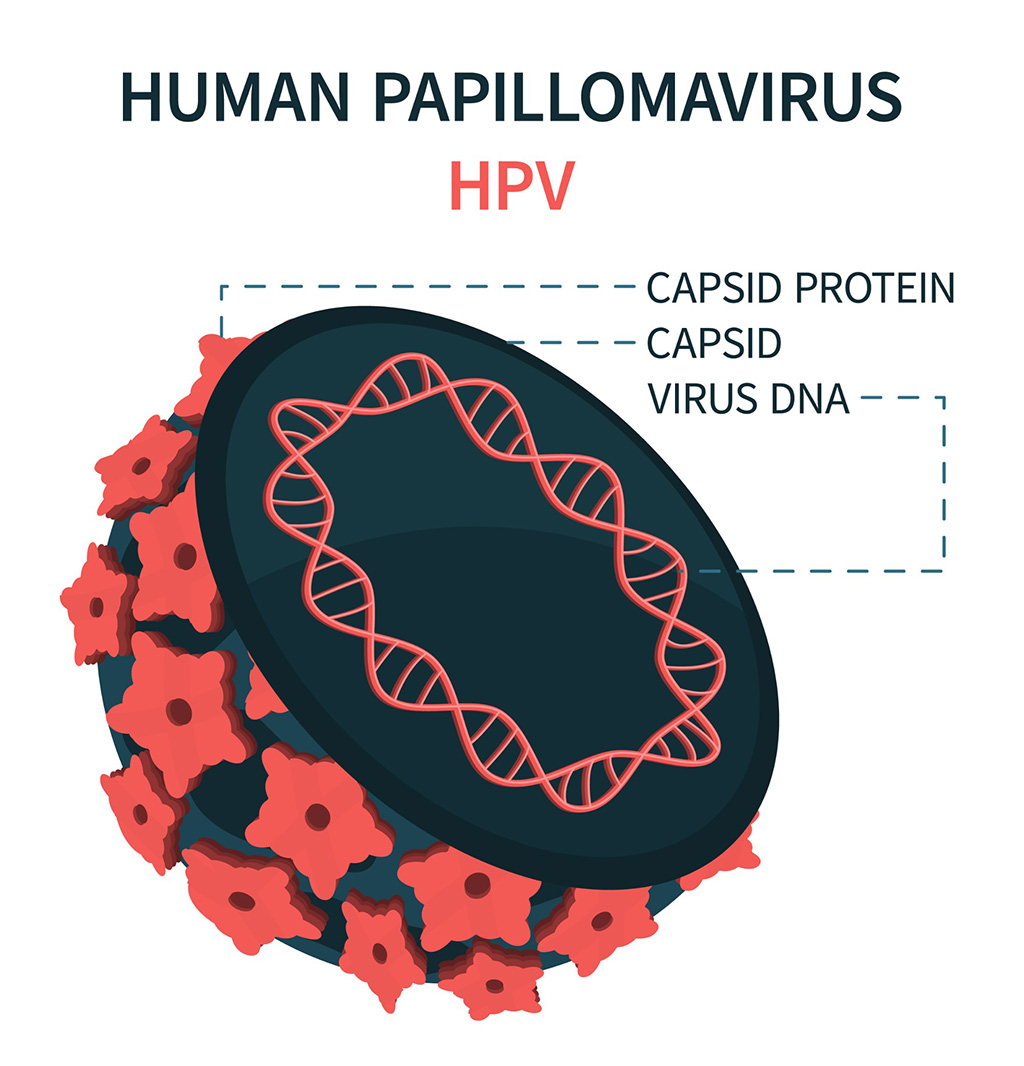
DNA Biosensor Enables Early Diagnosis of Cervical Cancer
Molybdenum disulfide (MoS2), recognized for its potential to form two-dimensional nanosheets like graphene, is a material that's increasingly catching the eye of the scientific community.... Read more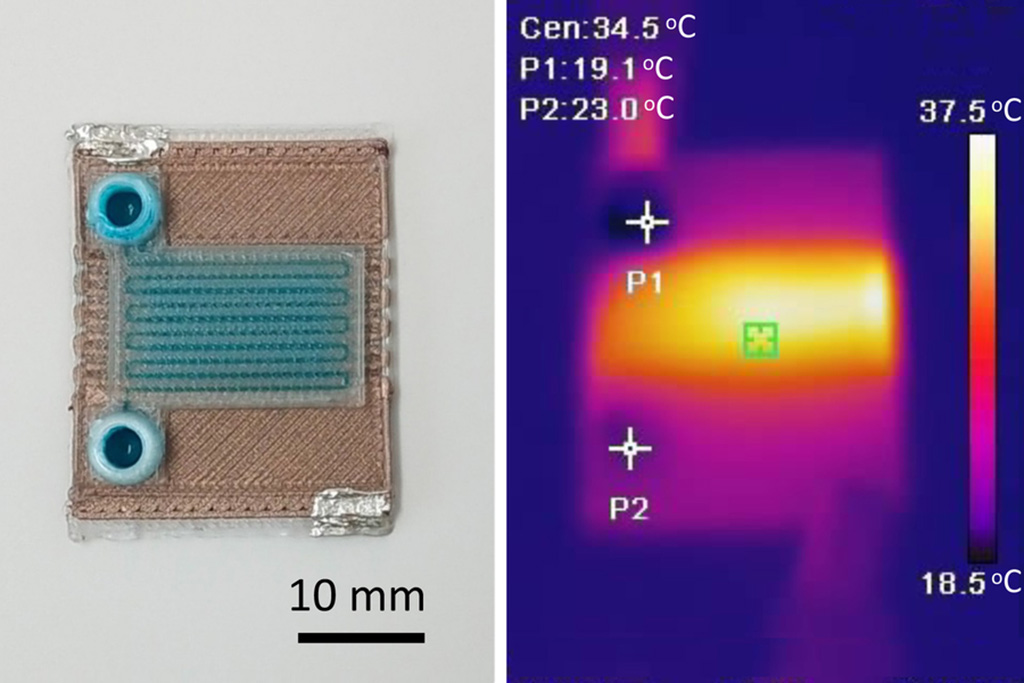
Self-Heating Microfluidic Devices Can Detect Diseases in Tiny Blood or Fluid Samples
Microfluidics, which are miniature devices that control the flow of liquids and facilitate chemical reactions, play a key role in disease detection from small samples of blood or other fluids.... Read more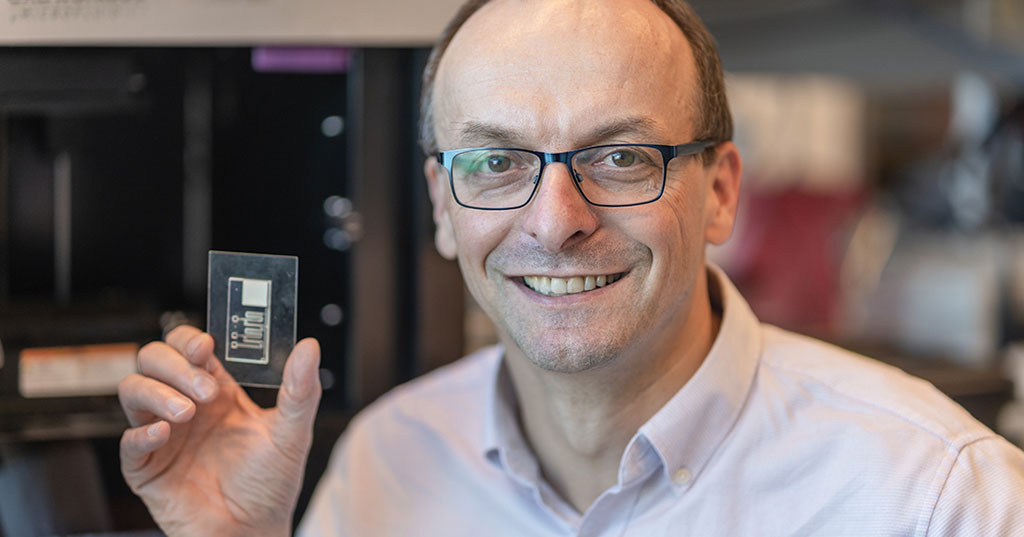