Optimized Microscope Hardware Developed for Accurate Image Classification
By LabMedica International staff writers Posted on 03 Dec 2019 |
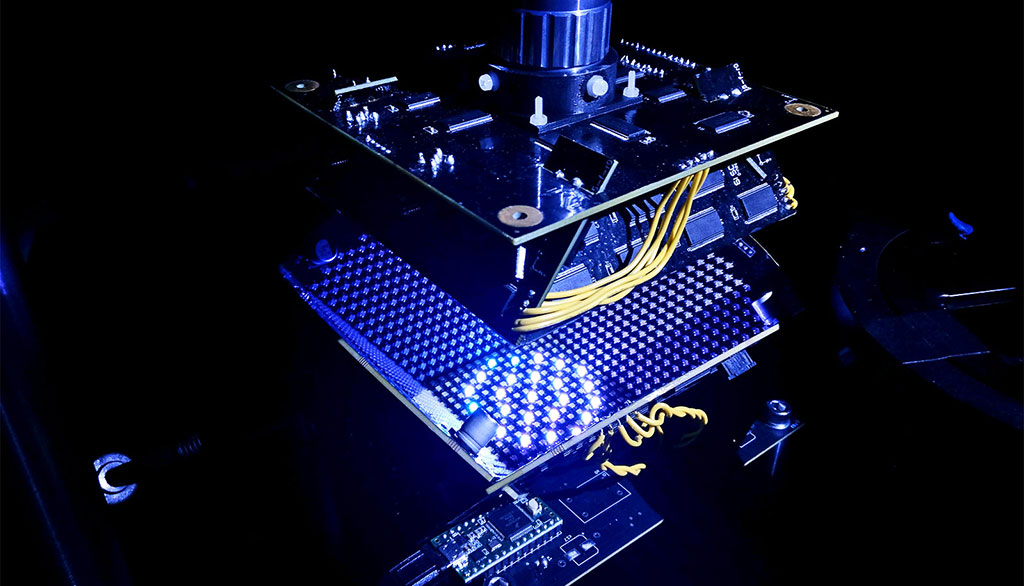
Image: A new type of microscope has been developed that uses a bowl studded with LED lights of various colors and lighting schemes produced by machine learning (Photo courtesy of Duke University)
Despite the widespread automation enabled by new post-processing software, the physical layout of the standard microscope has still changed relatively little as it is, for the most part, still optimized for a human viewer to peer through and inspect what is placed beneath.
A microscope has been developed that adapts its lighting angles, colors and patterns while teaching itself the optimal settings needed to complete a given diagnostic task. Rather than diffusing white light from below to evenly illuminate the slide, engineers developed a bowl-shaped light source with LEDs embedded throughout its surface. This allows samples to be illuminated from different angles up to nearly 90 degrees with different colors, which essentially casts shadows and highlights different features of the sample depending on the pattern of LEDs used.
An international team of bioengineers working with Duke University (Durham NC, USA) fed the microscope hundreds of samples of malaria-infected red blood cells prepared as thin smears, in which the cell bodies remain whole and are ideally spread out in a single layer on a microscope slide. Using a type of machine learning algorithm called a convolutional neural network; the microscope learned which features of the sample were most important for diagnosing malaria and how best to highlight those features.
The algorithm eventually landed on a ring-shaped LED pattern of different colors coming from relatively high angles. While the resulting images are noisier than a regular microscope image, they highlight the malaria parasite in a bright spot and are correctly classified about 90% of the time. Trained physicians and other machine learning algorithms typically perform with about 75% accuracy. The team also showed that the microscope works well with thick blood smear preparations, in which the red blood cells form a highly non-uniform background and may be broken apart. For this preparation, the machine learning algorithm was successful 99% of the time.
Roarke Horstmeyer, PhD, an assistant professor of biomedical engineering and senior author of the study, said, “A standard microscope illuminates a sample with the same amount of light coming from all directions, and that lighting has been optimized for human eyes over hundreds of years. But computers can see things humans can't. So not only have we redesigned the hardware to provide a diverse range of lighting options, we've allowed the microscope to optimize the illumination for itself.” The study was published in the November, 2019 issue of the journal Biomedical Optics Express.
Related Links:
Duke University
A microscope has been developed that adapts its lighting angles, colors and patterns while teaching itself the optimal settings needed to complete a given diagnostic task. Rather than diffusing white light from below to evenly illuminate the slide, engineers developed a bowl-shaped light source with LEDs embedded throughout its surface. This allows samples to be illuminated from different angles up to nearly 90 degrees with different colors, which essentially casts shadows and highlights different features of the sample depending on the pattern of LEDs used.
An international team of bioengineers working with Duke University (Durham NC, USA) fed the microscope hundreds of samples of malaria-infected red blood cells prepared as thin smears, in which the cell bodies remain whole and are ideally spread out in a single layer on a microscope slide. Using a type of machine learning algorithm called a convolutional neural network; the microscope learned which features of the sample were most important for diagnosing malaria and how best to highlight those features.
The algorithm eventually landed on a ring-shaped LED pattern of different colors coming from relatively high angles. While the resulting images are noisier than a regular microscope image, they highlight the malaria parasite in a bright spot and are correctly classified about 90% of the time. Trained physicians and other machine learning algorithms typically perform with about 75% accuracy. The team also showed that the microscope works well with thick blood smear preparations, in which the red blood cells form a highly non-uniform background and may be broken apart. For this preparation, the machine learning algorithm was successful 99% of the time.
Roarke Horstmeyer, PhD, an assistant professor of biomedical engineering and senior author of the study, said, “A standard microscope illuminates a sample with the same amount of light coming from all directions, and that lighting has been optimized for human eyes over hundreds of years. But computers can see things humans can't. So not only have we redesigned the hardware to provide a diverse range of lighting options, we've allowed the microscope to optimize the illumination for itself.” The study was published in the November, 2019 issue of the journal Biomedical Optics Express.
Related Links:
Duke University
Latest Technology News
- New Diagnostic System Achieves PCR Testing Accuracy
- DNA Biosensor Enables Early Diagnosis of Cervical Cancer
- Self-Heating Microfluidic Devices Can Detect Diseases in Tiny Blood or Fluid Samples
- Breakthrough in Diagnostic Technology Could Make On-The-Spot Testing Widely Accessible
- First of Its Kind Technology Detects Glucose in Human Saliva
- Electrochemical Device Identifies People at Higher Risk for Osteoporosis Using Single Blood Drop
- Novel Noninvasive Test Detects Malaria Infection without Blood Sample
- Portable Optofluidic Sensing Devices Could Simultaneously Perform Variety of Medical Tests
- Point-of-Care Software Solution Helps Manage Disparate POCT Scenarios across Patient Testing Locations
- Electronic Biosensor Detects Biomarkers in Whole Blood Samples without Addition of Reagents
- Breakthrough Test Detects Biological Markers Related to Wider Variety of Cancers
- Rapid POC Sensing Kit to Determine Gut Health from Blood Serum and Stool Samples
- Device Converts Smartphone into Fluorescence Microscope for Just USD 50
- Wi-Fi Enabled Handheld Tube Reader Designed for Easy Portability
Channels
Clinical Chemistry
view channel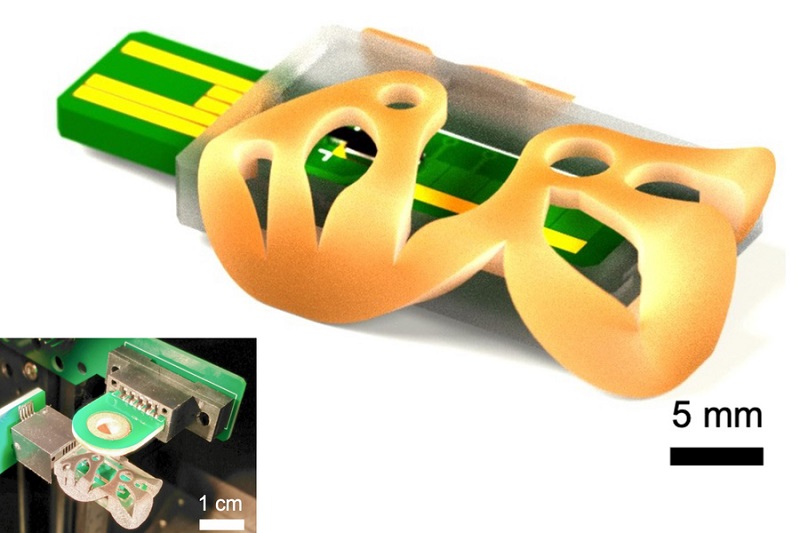
3D Printed Point-Of-Care Mass Spectrometer Outperforms State-Of-The-Art Models
Mass spectrometry is a precise technique for identifying the chemical components of a sample and has significant potential for monitoring chronic illness health states, such as measuring hormone levels... Read more.jpg)
POC Biomedical Test Spins Water Droplet Using Sound Waves for Cancer Detection
Exosomes, tiny cellular bioparticles carrying a specific set of proteins, lipids, and genetic materials, play a crucial role in cell communication and hold promise for non-invasive diagnostics.... Read more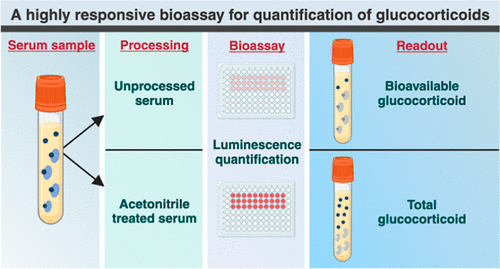
Highly Reliable Cell-Based Assay Enables Accurate Diagnosis of Endocrine Diseases
The conventional methods for measuring free cortisol, the body's stress hormone, from blood or saliva are quite demanding and require sample processing. The most common method, therefore, involves collecting... Read moreMolecular Diagnostics
view channel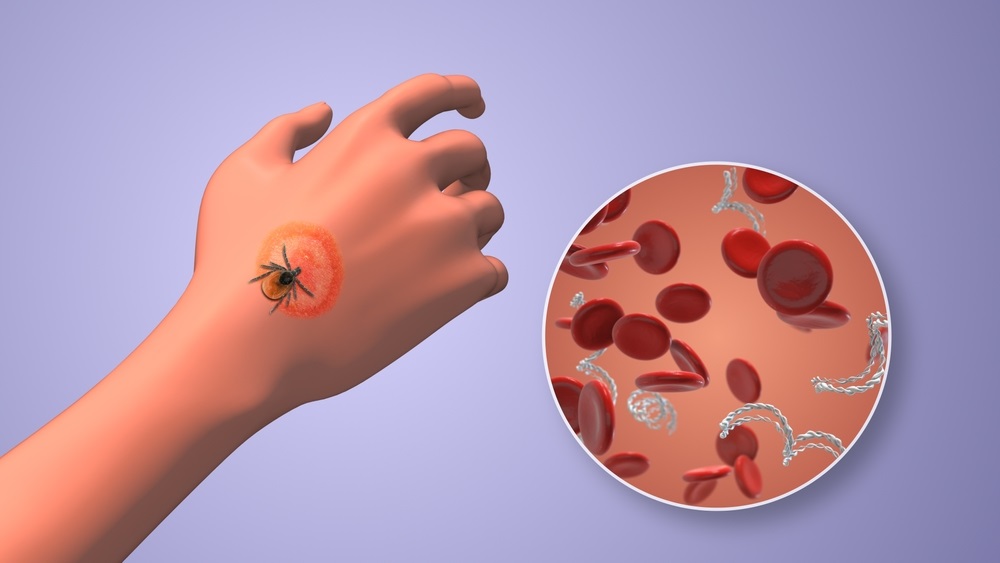
Urine Test to Revolutionize Lyme Disease Testing
Lyme disease is the most common animal-to-human transmitted disease in the United States, with around 476,000 people diagnosed and treated annually, and its incidence has been increasing.... Read more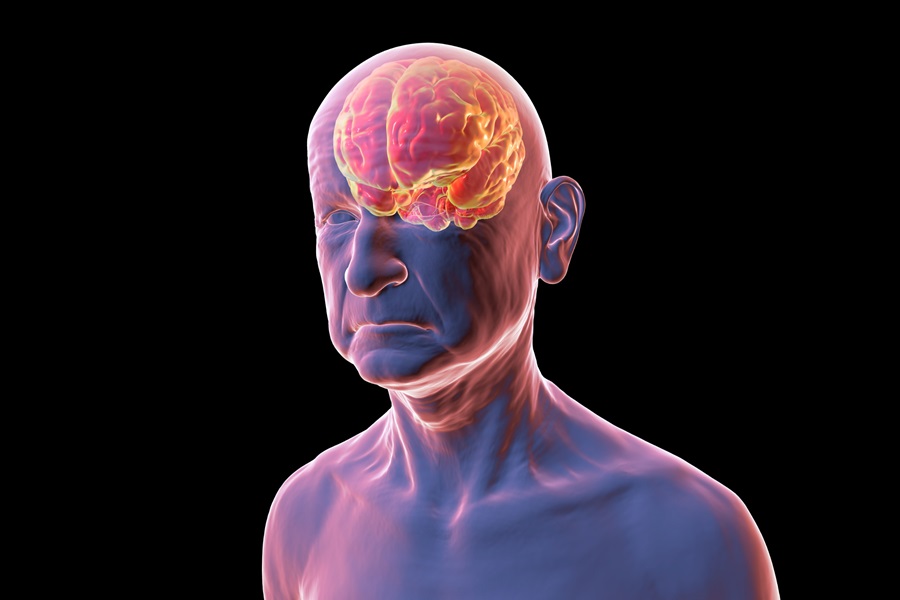
Simple Blood Test Could Enable First Quantitative Assessments for Future Cerebrovascular Disease
Cerebral small vessel disease is a common cause of stroke and cognitive decline, particularly in the elderly. Presently, assessing the risk for cerebral vascular diseases involves using a mix of diagnostic... Read more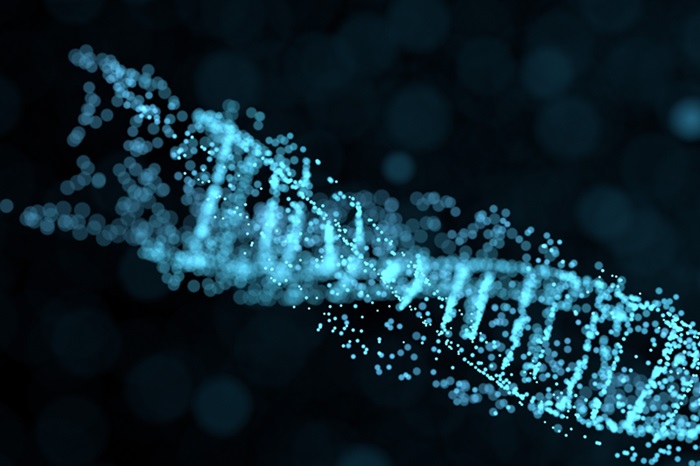
New Genetic Testing Procedure Combined With Ultrasound Detects High Cardiovascular Risk
A key interest area in cardiovascular research today is the impact of clonal hematopoiesis on cardiovascular diseases. Clonal hematopoiesis results from mutations in hematopoietic stem cells and may lead... Read moreHematology
view channel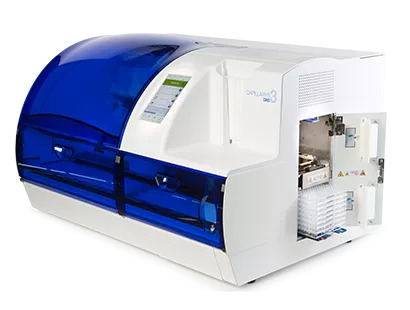
Next Generation Instrument Screens for Hemoglobin Disorders in Newborns
Hemoglobinopathies, the most widespread inherited conditions globally, affect about 7% of the population as carriers, with 2.7% of newborns being born with these conditions. The spectrum of clinical manifestations... Read more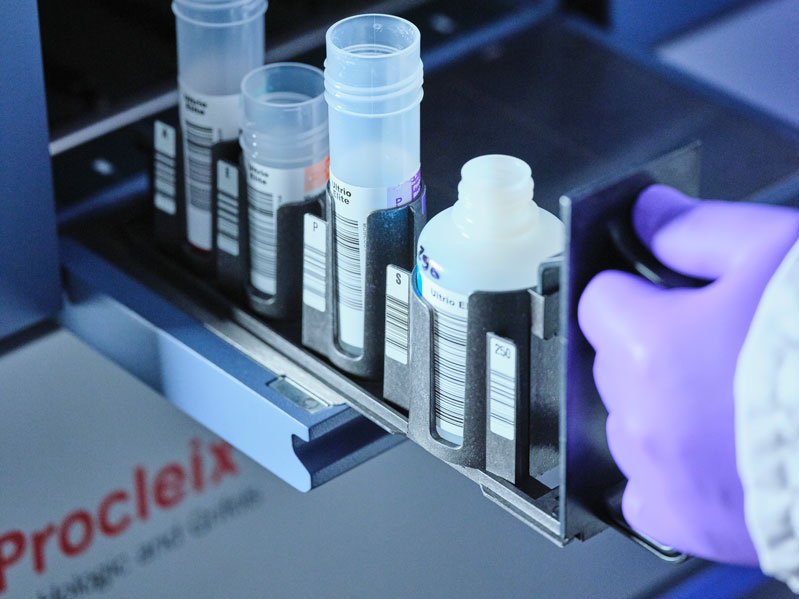
First 4-in-1 Nucleic Acid Test for Arbovirus Screening to Reduce Risk of Transfusion-Transmitted Infections
Arboviruses represent an emerging global health threat, exacerbated by climate change and increased international travel that is facilitating their spread across new regions. Chikungunya, dengue, West... Read more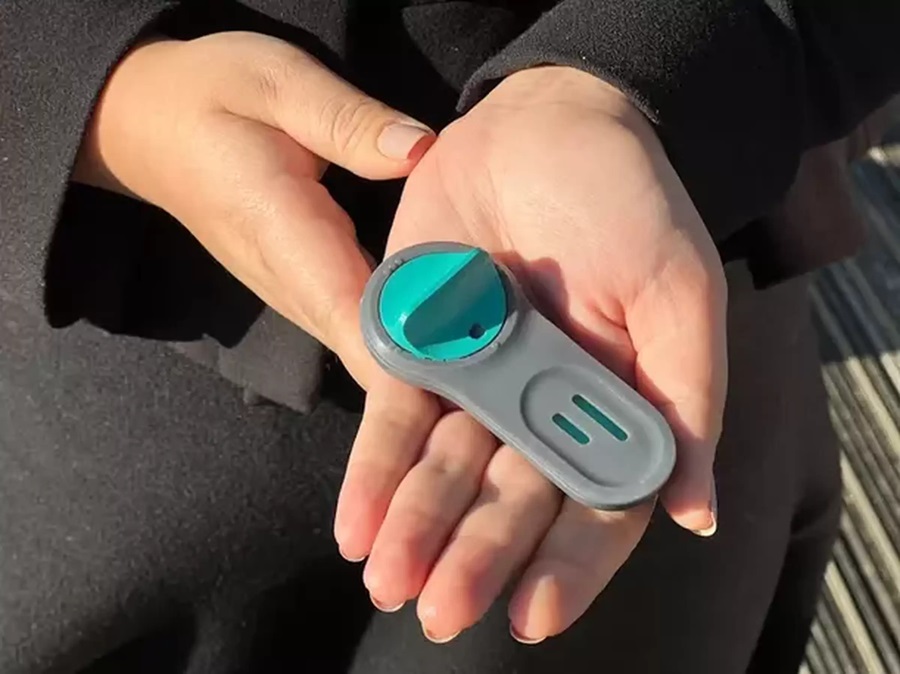
POC Finger-Prick Blood Test Determines Risk of Neutropenic Sepsis in Patients Undergoing Chemotherapy
Neutropenia, a decrease in neutrophils (a type of white blood cell crucial for fighting infections), is a frequent side effect of certain cancer treatments. This condition elevates the risk of infections,... Read more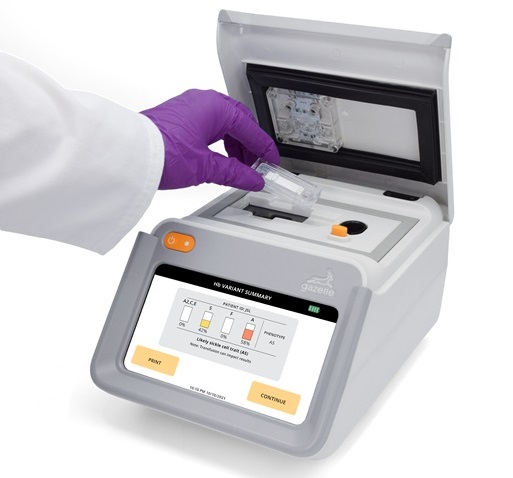
First Affordable and Rapid Test for Beta Thalassemia Demonstrates 99% Diagnostic Accuracy
Hemoglobin disorders rank as some of the most prevalent monogenic diseases globally. Among various hemoglobin disorders, beta thalassemia, a hereditary blood disorder, affects about 1.5% of the world's... Read moreImmunology
view channel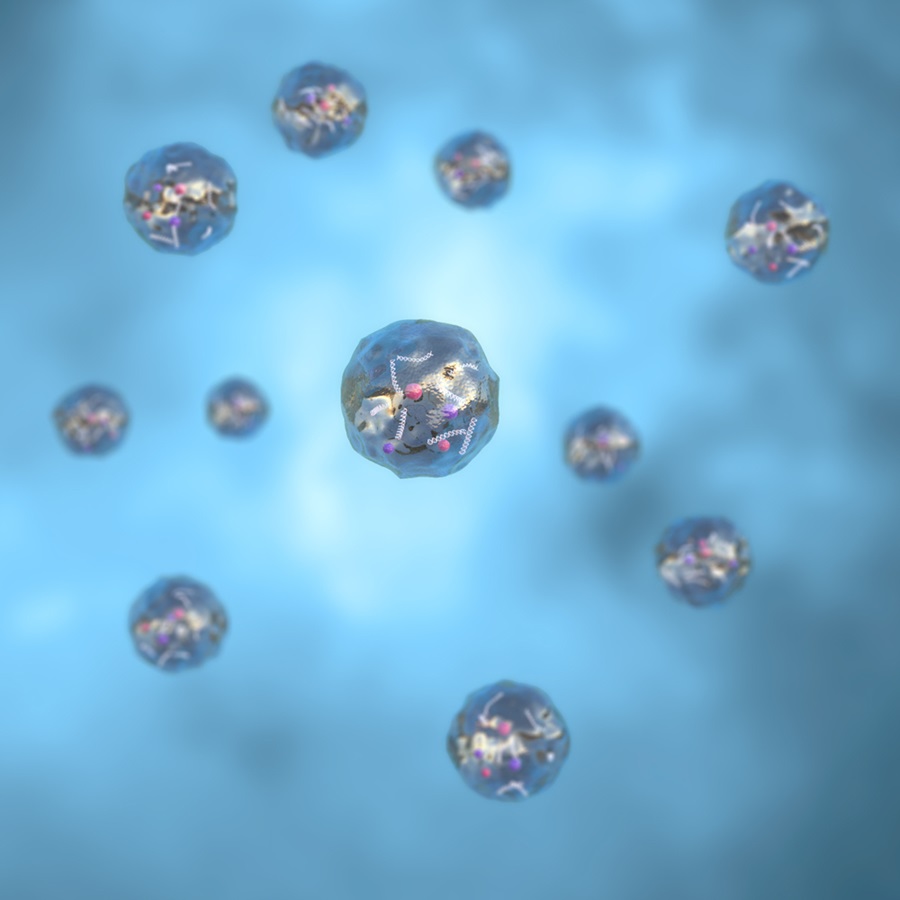
Diagnostic Blood Test for Cellular Rejection after Organ Transplant Could Replace Surgical Biopsies
Transplanted organs constantly face the risk of being rejected by the recipient's immune system which differentiates self from non-self using T cells and B cells. T cells are commonly associated with acute... Read more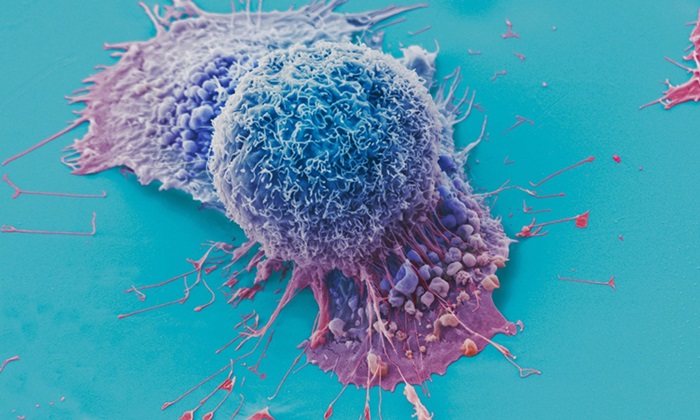
AI Tool Precisely Matches Cancer Drugs to Patients Using Information from Each Tumor Cell
Current strategies for matching cancer patients with specific treatments often depend on bulk sequencing of tumor DNA and RNA, which provides an average profile from all cells within a tumor sample.... Read more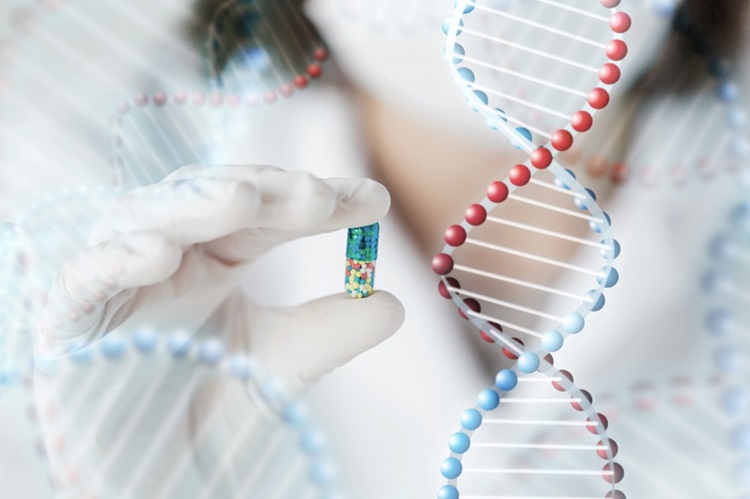
Genetic Testing Combined With Personalized Drug Screening On Tumor Samples to Revolutionize Cancer Treatment
Cancer treatment typically adheres to a standard of care—established, statistically validated regimens that are effective for the majority of patients. However, the disease’s inherent variability means... Read moreMicrobiology
view channel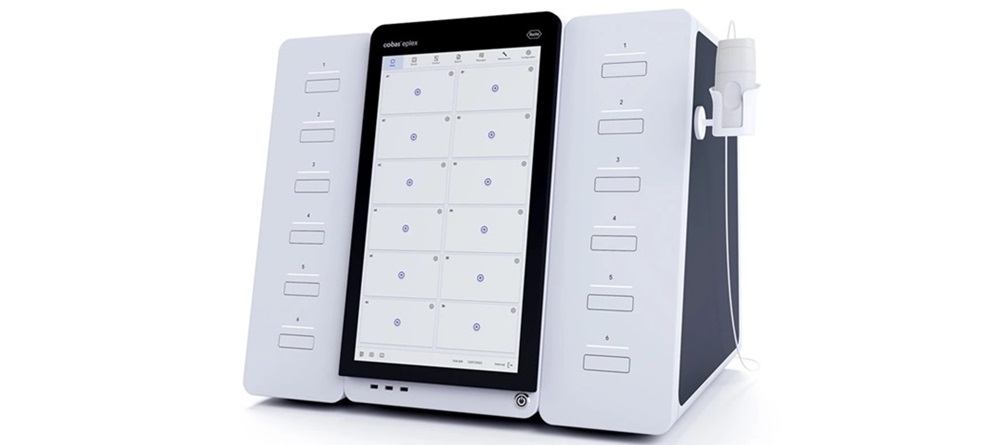
Enhanced Rapid Syndromic Molecular Diagnostic Solution Detects Broad Range of Infectious Diseases
GenMark Diagnostics (Carlsbad, CA, USA), a member of the Roche Group (Basel, Switzerland), has rebranded its ePlex® system as the cobas eplex system. This rebranding under the globally renowned cobas name... Read more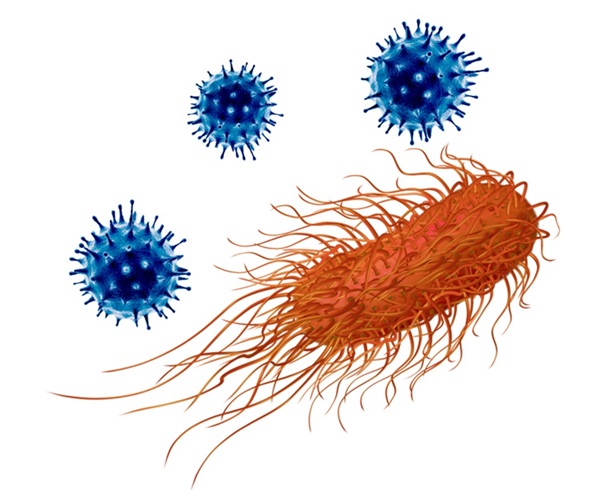
Clinical Decision Support Software a Game-Changer in Antimicrobial Resistance Battle
Antimicrobial resistance (AMR) is a serious global public health concern that claims millions of lives every year. It primarily results from the inappropriate and excessive use of antibiotics, which reduces... Read more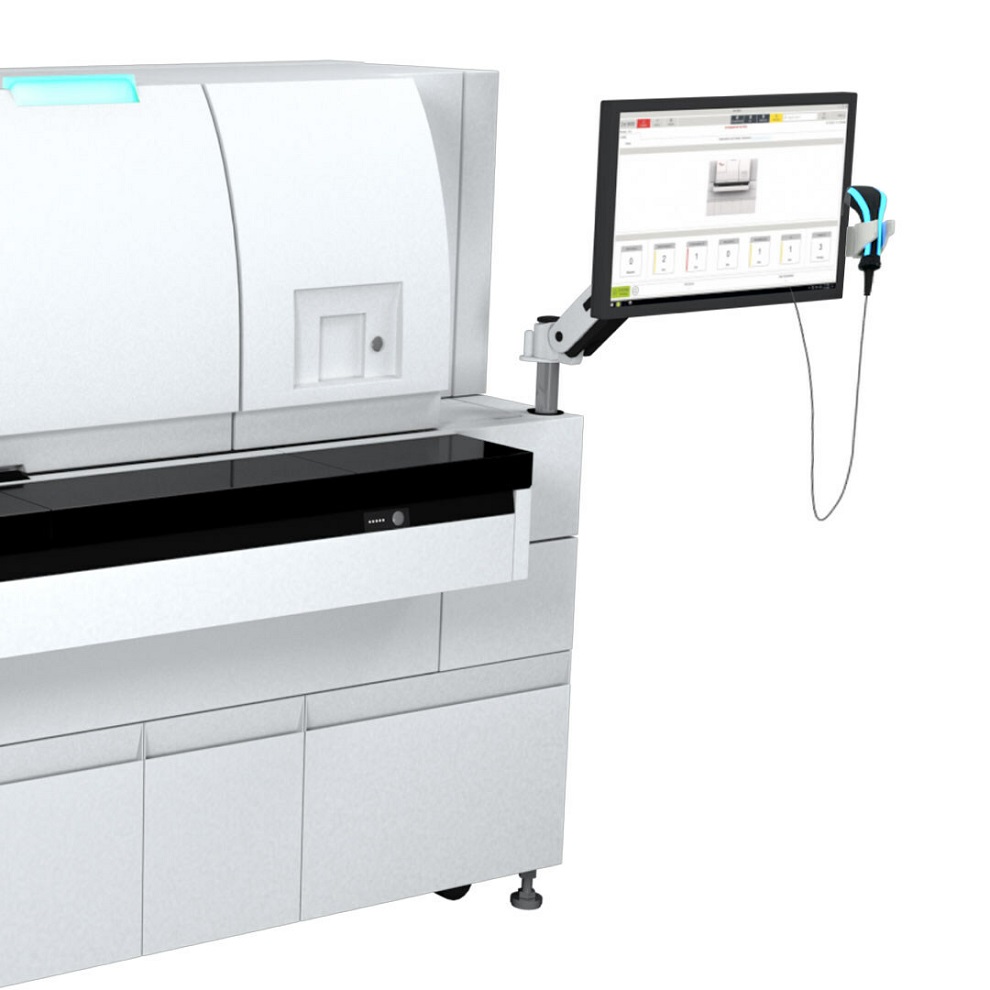
New CE-Marked Hepatitis Assays to Help Diagnose Infections Earlier
According to the World Health Organization (WHO), an estimated 354 million individuals globally are afflicted with chronic hepatitis B or C. These viruses are the leading causes of liver cirrhosis, liver... Read more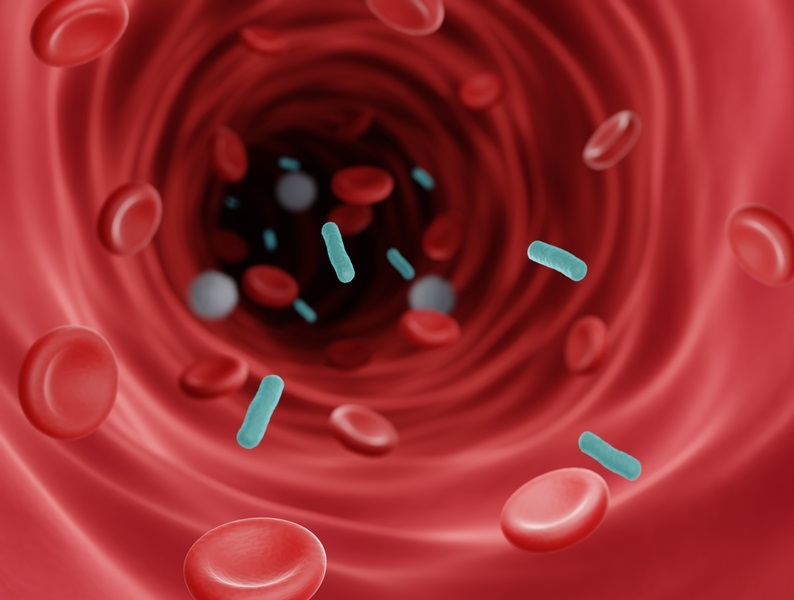
1 Hour, Direct-From-Blood Multiplex PCR Test Identifies 95% of Sepsis-Causing Pathogens
Sepsis contributes to one in every three hospital deaths in the US, and globally, septic shock carries a mortality rate of 30-40%. Diagnosing sepsis early is challenging due to its non-specific symptoms... Read morePathology
view channel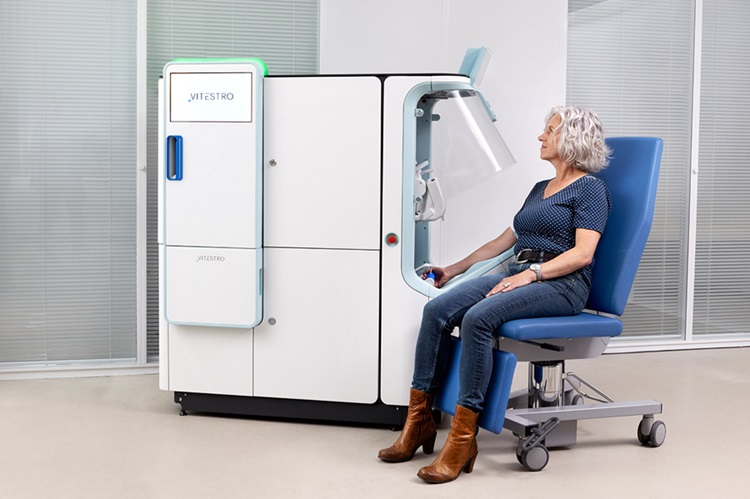
Robotic Blood Drawing Device to Revolutionize Sample Collection for Diagnostic Testing
Blood drawing is performed billions of times each year worldwide, playing a critical role in diagnostic procedures. Despite its importance, clinical laboratories are dealing with significant staff shortages,... Read more.jpg)
Use of DICOM Images for Pathology Diagnostics Marks Significant Step towards Standardization
Digital pathology is rapidly becoming a key aspect of modern healthcare, transforming the practice of pathology as laboratories worldwide adopt this advanced technology. Digital pathology systems allow... Read more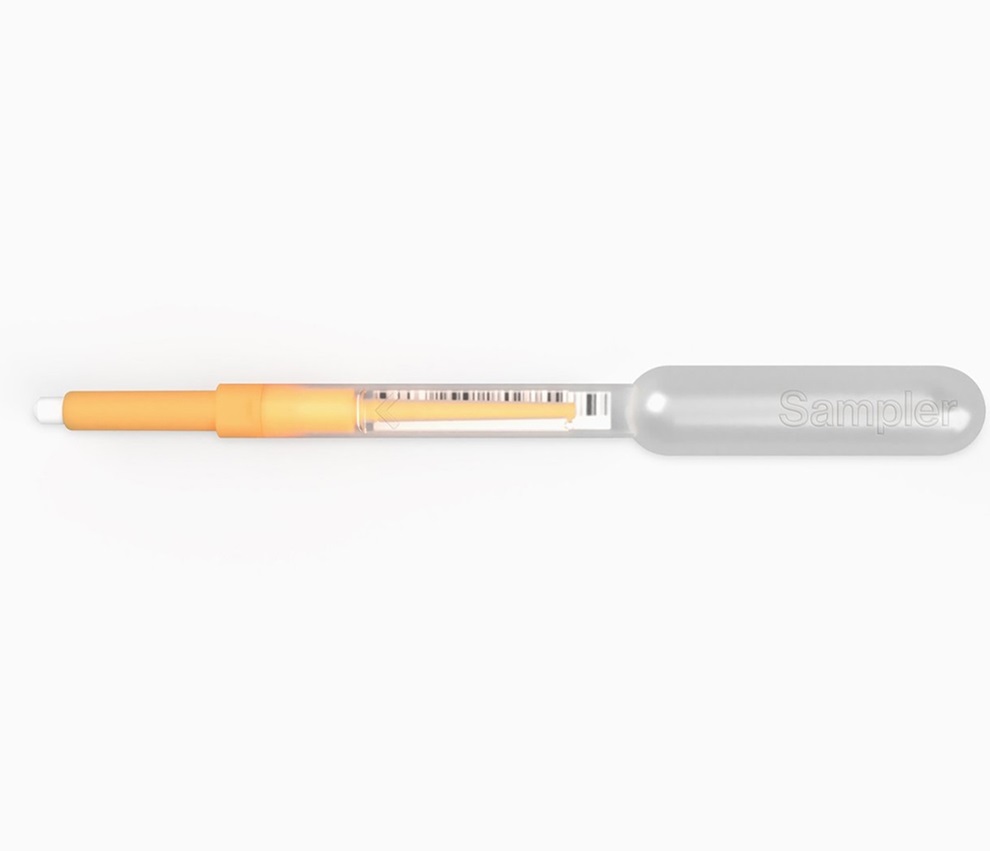
First of Its Kind Universal Tool to Revolutionize Sample Collection for Diagnostic Tests
The COVID pandemic has dramatically reshaped the perception of diagnostics. Post the pandemic, a groundbreaking device that combines sample collection and processing into a single, easy-to-use disposable... Read moreIndustry
view channel_1.jpg)
Thermo Fisher and Bio-Techne Enter Into Strategic Distribution Agreement for Europe
Thermo Fisher Scientific (Waltham, MA USA) has entered into a strategic distribution agreement with Bio-Techne Corporation (Minneapolis, MN, USA), resulting in a significant collaboration between two industry... Read more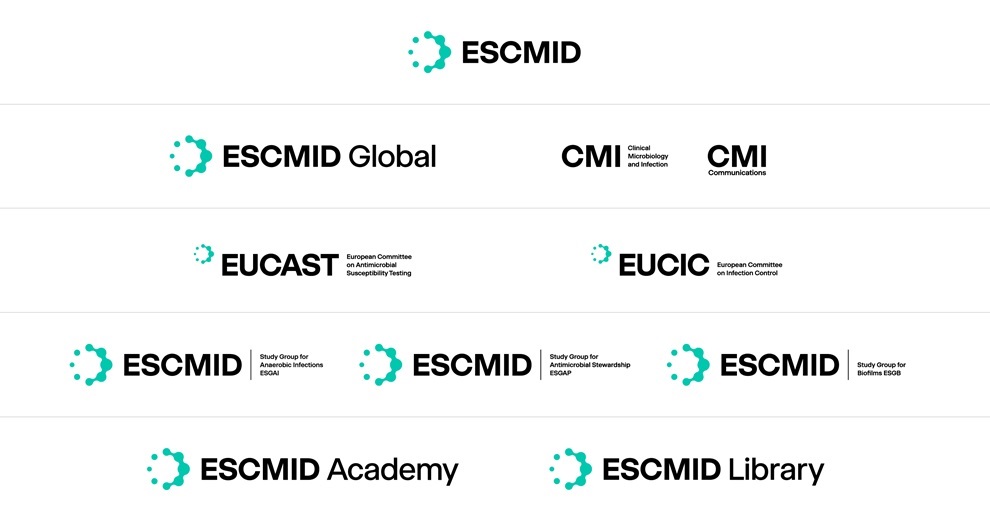
ECCMID Congress Name Changes to ESCMID Global
Over the last few years, the European Society of Clinical Microbiology and Infectious Diseases (ESCMID, Basel, Switzerland) has evolved remarkably. The society is now stronger and broader than ever before... Read more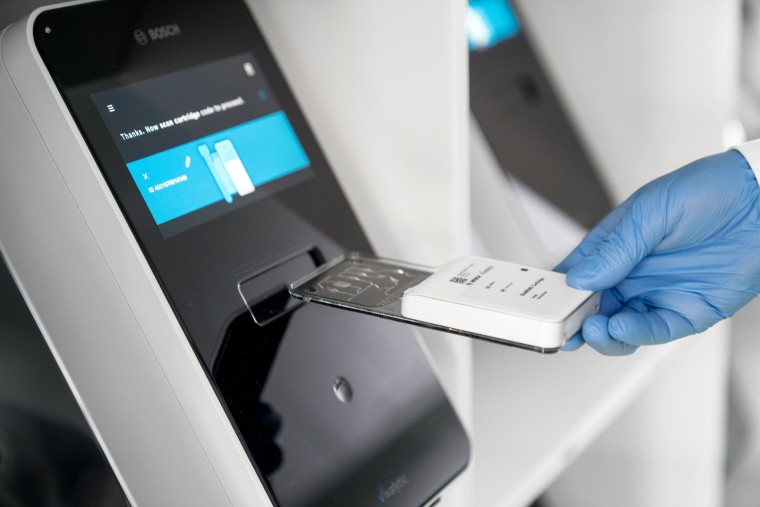