AI Tool Outperforms Human Pathologists in Predicting Survival after Colorectal Cancer Diagnosis
Posted on 14 Apr 2023
Colorectal cancer, the second most lethal cancer worldwide, exhibits varying behavior even among individuals with similar disease profiles who undergo the same treatment. Now, a new artificial intelligence (AI) model may now offer valuable insight to doctors making prognoses and determining treatments for patients with colorectal cancer.
Researchers at Harvard Medical School (Boston, MA, USA) and National Cheng Kung University (Tainan, Taiwan) have developed a tool called MOMA (Multi-omics Multi-cohort Assessment) that accurately predicts colorectal tumor aggressiveness, patient survival rates with and without disease recurrence, and the most effective therapy by analyzing tumor sample images alone. Unlike many existing AI tools that primarily replicate or optimize human expertise, MOMA identifies and interprets visual patterns on microscopy images that are undetectable to the human eye. The tool is freely available to researchers and clinicians.
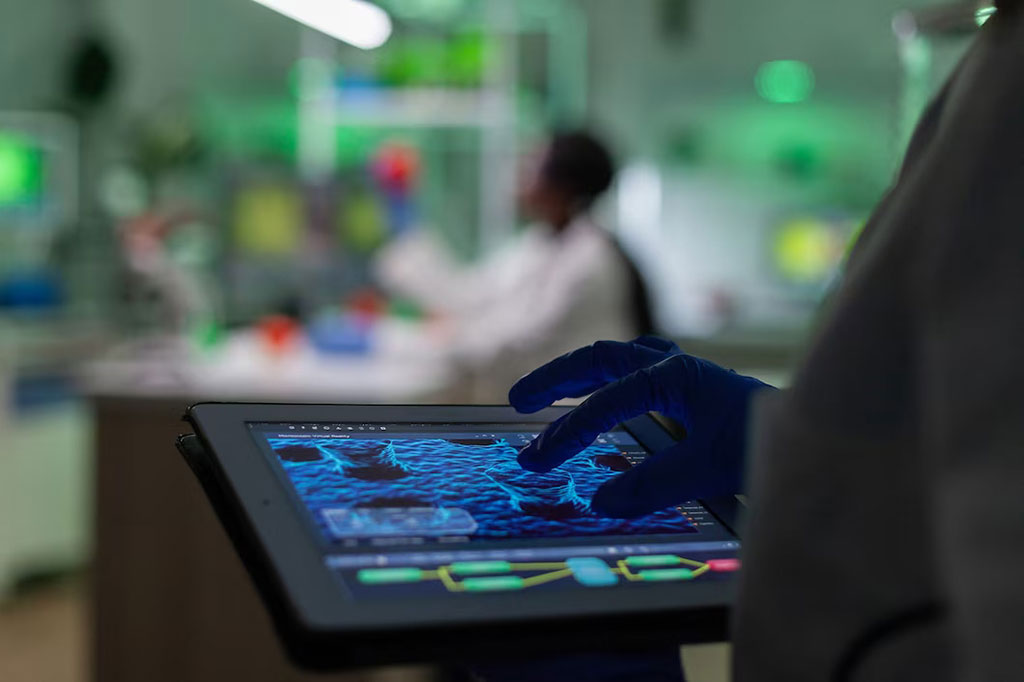
The model was trained using data from approximately 2,000 colorectal cancer patients from diverse national patient cohorts, totaling over 450,000 participants. During training, researchers provided the model with information about patients' age, sex, cancer stage, and outcomes, as well as genomic, epigenetic, protein, and metabolic profiles of the tumors. The model was then tasked with identifying visual markers related to tumor types, genetic mutations, epigenetic changes, disease progression, and patient survival using pathology images of tumor samples. The model's performance was assessed using a set of previously unseen tumor sample images from different patients, comparing its predictions to actual patient outcomes and other clinical data.
MOMA accurately predicted overall survival following diagnosis and the number of cancer-free years for patients. It also correctly anticipated individual patient responses to various therapies based on the presence of specific genetic mutations influencing cancer progression or spread. In both areas, the tool outperformed human pathologists and current AI models. The researchers recommend testing the model in a prospective, randomized trial evaluating its performance in real patients over time after initial diagnosis before deploying it in clinics and hospitals. Such a study would directly compare MOMA's real-life performance using only images with human clinicians who utilize additional knowledge and test results unavailable to the model, providing the gold-standard demonstration of its capabilities.
“Our model performs tasks that human pathologists cannot do based on image viewing alone,” said study co-senior author Kun-Hsing Yu, assistant professor of biomedical informatics in the Blavatnik Institute at Harvard Medical School, who led an international team of pathologists, oncologists, biomedical informaticians, and computer scientists. “What we anticipate is not a replacement of human pathology expertise, but augmentation of what human pathologists can do. We fully expect that this approach will augment the current clinical practice of cancer management.”
Related Links:
Harvard Medical School
National Cheng Kung University