AI Model Accurately Shows Presence and Location of Cancer in Pathological Images
Posted on 15 Aug 2022
It is usually necessary to accurately mark the location of the cancer site in pathological images to solve the problems involved with zoning that indicates the location information of cancer, which takes a long time and therefore increased cost. Existing deep learning models need to construct a dataset, in which the location of the cancer was accurately drawn, to specify the cancer site. Now, researchers have developed a weakly supervised deep learning model that can accurately show the presence and location of cancer in pathological images based only on data where the cancer is present. The deep learning model improves efficiency and is expected to make a significant contribution to the relevant research field.
Scientists at Daegu Gyeongbuk Institute of Science and Technology (DGIST, Daegu, Korea) developed the weakly supervised learning model that zones cancer sites with only rough data such as 'whether the cancer in the image is present or not' is under active study. However, there would be a significant deterioration in performance if the existing weakly supervised learning model is applied to a huge pathological image dataset where the size of one image is as large as a few gigabytes. To solve this problem, the researchers attempted to improve performance by dividing the pathological image into patches, although the divided patches lose the correlation between the location information and each split data, which means that there is a limit to using all of the available information.
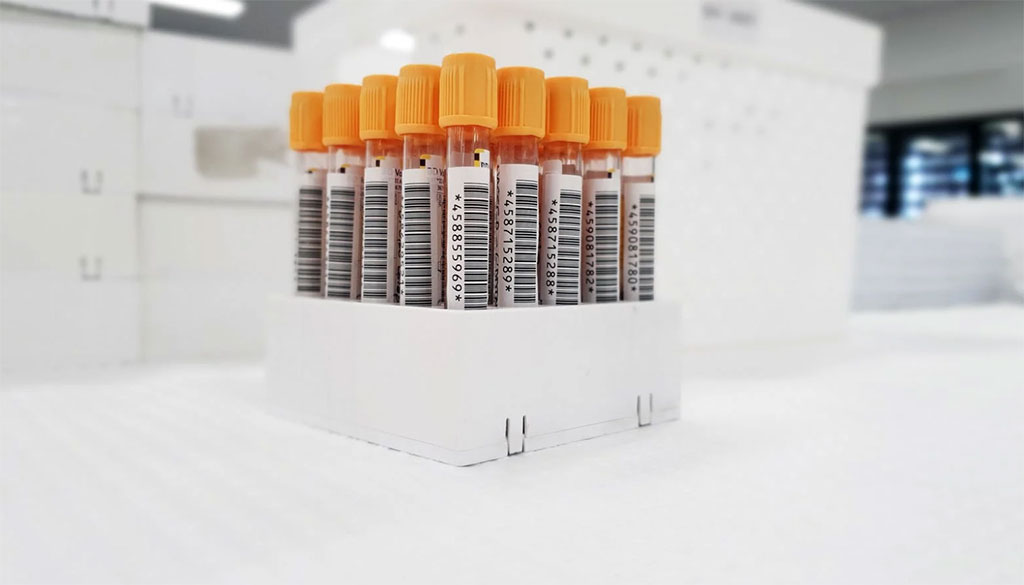
In response, the research team discovered a technique of segmenting down to the cancer site solely based on the learned data indicating the presence of cancer by slide. The team developed a pathological image compression technology that first teaches the network to effectively extract significant features from the patches through unsupervised contrastive learning and uses this to detect the main features while maintaining each location information to reduce the size of the image while maintaining the correlation between the patches. Later, the team developed a model that can find the regions that are highly likely to have cancer from the compressed pathology images by using a class activation map and zone all of these regions from the entire pathology images using a pixel correlation module (PCM). The newly developed deep learning model showed a dice similarity coefficient (DSC) score of up to 81-84 only with the learning data with slide-level cancer labels in the cancer zoning problem. It significantly exceeded the performance of previously proposed patch level methods or other weakly supervised learning techniques (DSC score: 20 - 70).
“The model developed through this study has greatly improved the performance of weakly supervised learning of pathological images, and it is expected to contribute to improving the efficiency of various studies requiring pathological image analysis,” said Professor Park Sang-Hyun of the Department of Robotics and Mechatronics Engineering at DGIST. “If we can improve the related technology further in the future, it will be possible to use it universally for various medical image zoning issues.”
Related Links:
DGIST