New Data-Driven Testing Strategy Could Enable Fast Turnaround of COVID-19 Test Results for Healthcare Workers
By LabMedica International staff writers
Posted on 27 Apr 2021
A new methodology for testing pooled samples that maximizes the proportion of samples resolved after a single round of testing can speed up COVID-19 test results for healthcare workers.Posted on 27 Apr 2021
Investigators at University of Manchester (Manchester, UK) have developed a COVID-19 testing strategy that maximizes the proportion of negative results after a single round of testing, allowing prompt notification of results. The method also reduces the need for increasingly limited test reagents, as fewer additional tests are required.
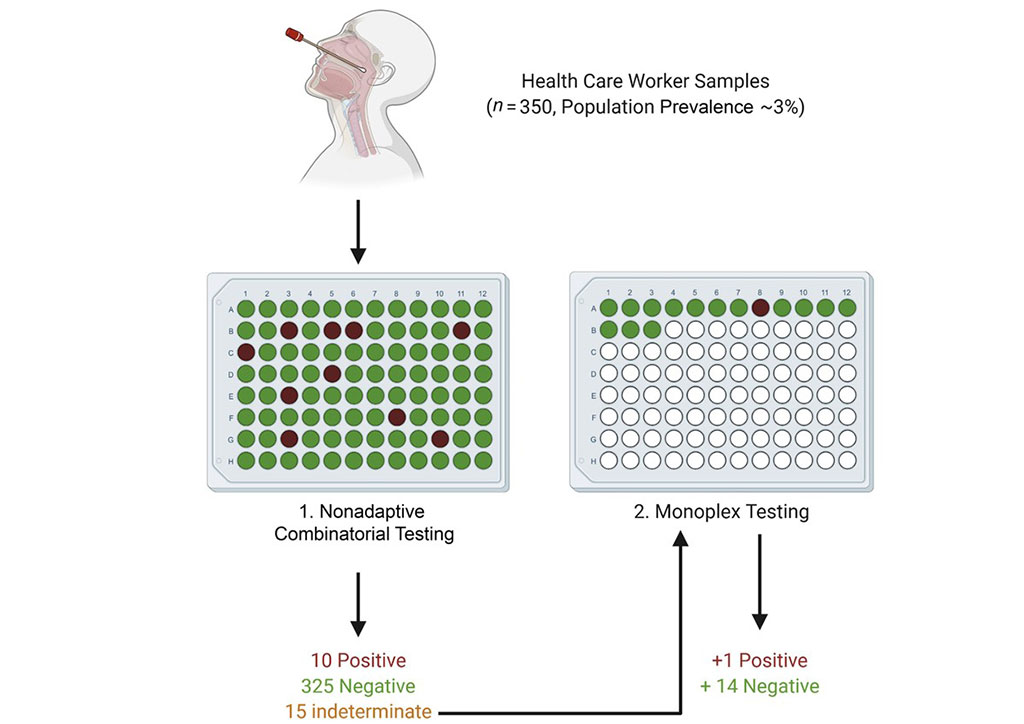
Image: Proposed testing approach for healthcare worker screening (Photo courtesy of The Journal of Molecular Diagnostics)
Fast turnaround of COVID-19 test results for healthcare workers is critical. There is an urgent need to reduce the spread of COVID-19 transmission in hospitals and care facilities and to maintain adequate levels of staffing. Group testing strategies with pooled samples have been proposed to increase capacity; however, the currently used strategies are slow.
Traditional Dorfman sequential (DS) pooling combines multiple samples and, if a pool test returns positive, all of the constituent samples undergo further testing. In a healthcare setting, this means that even individuals who ultimately test negative for COVID-19 will have to isolate. The investigators developed a non-adaptive combinatorial (NAC) pooling approach that tests the same sample in several simultaneously assayed pools. The algorithm assumes initially that each sample is positive. It then attempts to disprove this assumption by finding a well in which the sample has been placed that has tested as negative. Then another algorithm is used to find positive wells that contain a single sample on the list of the remaining potentially positive samples. Indeterminate samples are retested.
To establish a suitable limit of detection for pooling, nasopharyngeal samples of known SARS-CoV-2 status were placed in two pools, each containing 14 SARS-CoV-2 negative samples and one SARS-CoV-2 positive sample, with the positive samples at differing viral loads. Pooling matrices were generated for 700, 350, and 250 samples, with each sample assigned to 2, 4, and 5 wells, respectively. The samples were also tested in a DS testing scheme. The efficacy of each matrix was tested under different SARS-CoV-2 prevalence levels of 0.1%, 3%, 7%, and 10% of the population.
All NAC matrices performed well at low prevalence levels, with an average of 585 tests saved per assay in the 700 sample matrix. In simulations of low-to-medium prevalence levels (0.1% – 3%), which is the prevalence expected in an asymptomatic healthcare worker population, all the NAC matrices required fewer retests than the DS testing scheme. However, as the population prevalence increased, the performance of each matrix deteriorated. The matrices and system to decode the results are freely available and laboratories can choose the matrix that best suits their current population prevalence and sample size, facilitating a context-specific, relatively low cost data-driven testing approach.
“Using the method we have developed, any laboratory could adapt their testing scheme based on the current throughput and the current prevalence of infection in the population, facilitating a data-driven testing strategy,” explained lead investigators Graeme Black, DPhil, and John Henry McDermott, MD, both from the Division of Evolution and Genomic Sciences, School of Biological Sciences, University of Manchester, Manchester, UK. “Many high-throughput testing schemes for SARS-CoV-2 detection have been developed over the past year. We illustrate the potential power that adaptable automated, innovative mathematical approaches have to increase COVID-19 diagnostic capability in a safe manner. Such an approach could reach far greater numbers, save lives, and be delivered in a sustainable way. Undoubtedly, this has considerable relevance to other future population-based screening approaches.”
Related Links:
University of Manchester