Advanced Predictive Algorithms Identify Patients Having Undiagnosed Cancer
Posted on 07 May 2025
Two newly developed advanced predictive algorithms leverage a person’s health conditions and basic blood test results to accurately predict the likelihood of having an undiagnosed cancer, including challenging-to-diagnose liver and oral cancers. These innovative models have the potential to transform cancer detection in primary care, making it easier for patients to receive treatment at much earlier stages.
Currently, the UK's NHS uses prediction tools like the QCancer scores, which integrate various patient data to identify individuals at high risk for undiagnosed cancer, allowing general practitioners and specialists to refer them for further testing. Researchers from Queen Mary University of London (London, UK) and the University of Oxford (Oxford, UK) utilized anonymized electronic health records from over 7.4 million adults in England to develop two new algorithms. These models are more sensitive than existing tools and could lead to improved clinical decision-making and earlier cancer detection. Significantly, the new algorithms incorporate not only patient details like age, family history, medical diagnoses, symptoms, and general health, but also include the results of seven routine blood tests. These blood tests, which measure full blood count and liver function, serve as biomarkers to enhance early cancer diagnosis.
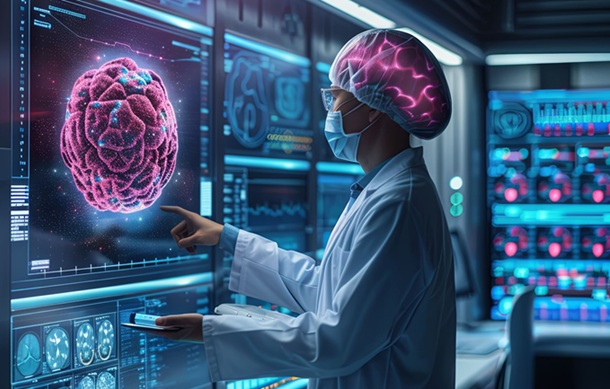
When compared with the current QCancer models, the new algorithms identified four additional medical conditions associated with an elevated risk of 15 different types of cancer, including those affecting the liver, kidneys, and pancreas. The new models also discovered two additional links between family history and lung or blood cancer, along with seven new symptoms—such as itching, bruising, back pain, hoarseness, flatulence, abdominal mass, and dark urine—that were associated with various types of cancer. The findings, published in Nature Communications, show that these new algorithms significantly improve diagnostic capabilities and are currently the only models applicable in primary care settings to assess the likelihood of undiagnosed liver cancer.
“These algorithms are designed to be embedded into clinical systems and used during routine GP consultations,” said Professor Julia Hippisley-Cox, Professor of Clinical Epidemiology and Predictive Medicine at Queen Mary University of London, and lead author of the study. “They offer a substantial improvement over current models, with higher accuracy in identifying cancers — especially at early, more treatable stages. They use existing blood test results which are already in the patients’ records making this an affordable and efficient approach to help the NHS meet its targets to improve its record on diagnosing cancer early by 2028.”