Prostate Cancer Diagnosed Better Using Artificial Intelligence
By LabMedica International staff writers Posted on 22 Jan 2020 |
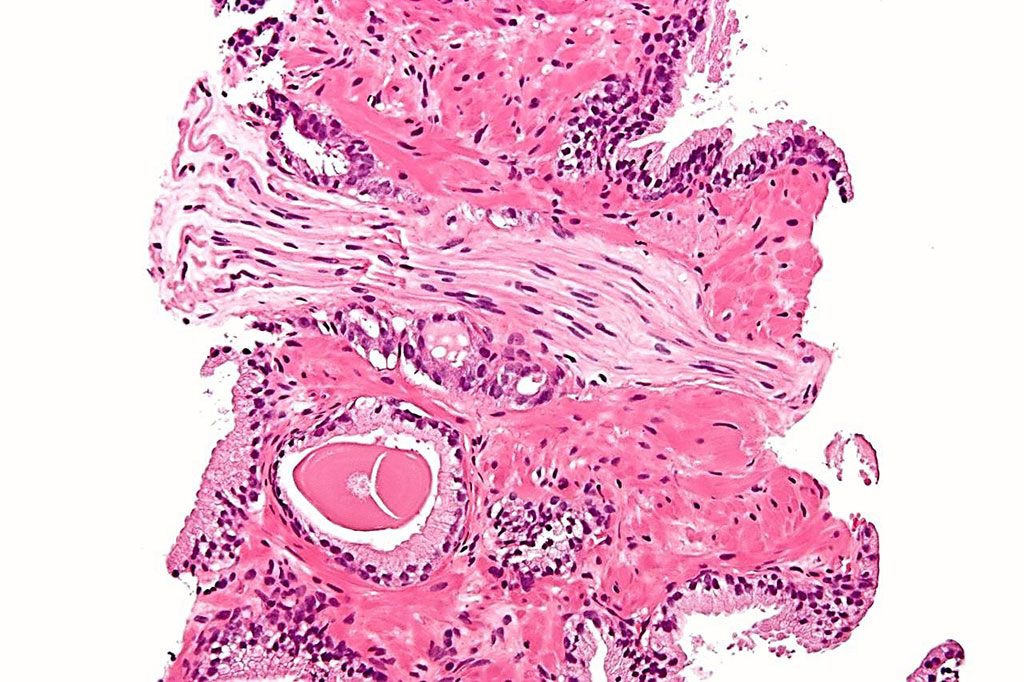
Image: Photomicrograph of a histological biopsy of a prostatic adenocarcinoma, conventional (acinar) type, the most common form of prostate cancer (Photo courtesy of Nephron).
Prostate cancer is a frequently occurring type of cancer, but not always aggressive: more men die with prostate cancer than from prostate cancer. However, its treatment has many consequences for the quality of life of patients, so determining aggressiveness is an important step in choosing a treatment.
To determine the aggressiveness of the cancer, biopsies are taken from the prostate, which are scored by a pathologist. This 'Gleason score' is then used to classify biopsies into five groups - the Gleason Grade Groups - which indicate the risk of dying from prostate cancer. However, this is a subjective process; whether and how a patient is treated may depend on the pathologist who assesses the tissue.
A team of scientists from the Radboud University Medical Center (Nijmegen, The Netherlands) developed an AI system that examines those biopsies the same way a pathologist does. The AI system also determines the Gleason score, and then the system can classify a biopsy according to the Gleason Grade Groups. By means of deep learning, the system examined thousands of images of biopsies to learn what a healthy prostate is, and what more or less aggressive prostate cancer tissue looks like. A semi-automatic labeling technique was used to circumvent the need for manual annotations by pathologists, using pathologists' reports as the reference standard during training. The system was developed to delineate individual glands, assign Gleason growth patterns, and determine the biopsy-level grade.
The investigators collected 5,759 biopsies from 1,243 patients. The developed system achieved a high agreement with the reference standard and scored highly at clinical decision thresholds: benign versus malignant (area under the curve 0.99), grade group of 2 or more (0.978), and grade group of 3 or more (0.974). In an observer experiment, the deep-learning system scored higher (kappa 0.854) than the panel (median kappa 0.819), outperforming 10 of 15 pathologist observers. On the external test dataset, the system obtained a high agreement with the reference standard set independently by two pathologists (quadratic Cohen's kappa 0.723 and 0.707) and within inter-observer variability (kappa 0.71).
The authors concluded that their automated deep-learning system achieved a performance similar to pathologists for Gleason grading and could potentially contribute to prostate cancer diagnosis. The system could potentially assist pathologists by screening biopsies, providing second opinions on grade group, and presenting quantitative measurements of volume percentages. The study was published on January 8, 2020 in the journal The Lancet Oncology.
Related Links:
Radboud University Medical Center
To determine the aggressiveness of the cancer, biopsies are taken from the prostate, which are scored by a pathologist. This 'Gleason score' is then used to classify biopsies into five groups - the Gleason Grade Groups - which indicate the risk of dying from prostate cancer. However, this is a subjective process; whether and how a patient is treated may depend on the pathologist who assesses the tissue.
A team of scientists from the Radboud University Medical Center (Nijmegen, The Netherlands) developed an AI system that examines those biopsies the same way a pathologist does. The AI system also determines the Gleason score, and then the system can classify a biopsy according to the Gleason Grade Groups. By means of deep learning, the system examined thousands of images of biopsies to learn what a healthy prostate is, and what more or less aggressive prostate cancer tissue looks like. A semi-automatic labeling technique was used to circumvent the need for manual annotations by pathologists, using pathologists' reports as the reference standard during training. The system was developed to delineate individual glands, assign Gleason growth patterns, and determine the biopsy-level grade.
The investigators collected 5,759 biopsies from 1,243 patients. The developed system achieved a high agreement with the reference standard and scored highly at clinical decision thresholds: benign versus malignant (area under the curve 0.99), grade group of 2 or more (0.978), and grade group of 3 or more (0.974). In an observer experiment, the deep-learning system scored higher (kappa 0.854) than the panel (median kappa 0.819), outperforming 10 of 15 pathologist observers. On the external test dataset, the system obtained a high agreement with the reference standard set independently by two pathologists (quadratic Cohen's kappa 0.723 and 0.707) and within inter-observer variability (kappa 0.71).
The authors concluded that their automated deep-learning system achieved a performance similar to pathologists for Gleason grading and could potentially contribute to prostate cancer diagnosis. The system could potentially assist pathologists by screening biopsies, providing second opinions on grade group, and presenting quantitative measurements of volume percentages. The study was published on January 8, 2020 in the journal The Lancet Oncology.
Related Links:
Radboud University Medical Center
Latest Pathology News
- AI-Powered Digital Imaging System to Revolutionize Cancer Diagnosis
- New Mycobacterium Tuberculosis Panel to Support Real-Time Surveillance and Combat Antimicrobial Resistance
- New Method Offers Sustainable Approach to Universal Metabolic Cancer Diagnosis
- Spatial Tissue Analysis Identifies Patterns Associated With Ovarian Cancer Relapse
- Unique Hand-Warming Technology Supports High-Quality Fingertip Blood Sample Collection
- Image-Based AI Shows Promise for Parasite Detection in Digitized Stool Samples
- Deep Learning Powered AI Algorithms Improve Skin Cancer Diagnostic Accuracy
- Microfluidic Device for Cancer Detection Precisely Separates Tumor Entities
- Virtual Skin Biopsy Determines Presence of Cancerous Cells
- AI Detects Viable Tumor Cells for Accurate Bone Cancer Prognoses Post Chemotherapy
- First Ever Technique Identifies Single Cancer Cells in Blood for Targeted Treatments
- Innovative Blood Collection Device Overcomes Common Obstacles Related to Phlebotomy
- Intra-Operative POC Device Distinguishes Between Benign and Malignant Ovarian Cysts within 15 Minutes
- Simple Skin Biopsy Test Detects Parkinson’s and Related Neurodegenerative Diseases
- Bioinformatics Tool to Identify Chromosomal Alterations in Tumor Cells Can Improve Cancer Diagnosis
- Coin-Sized Device Rapidly Isolates Blood Plasma for Quicker and More Precise Clinical Diagnoses
Channels
Clinical Chemistry
view channel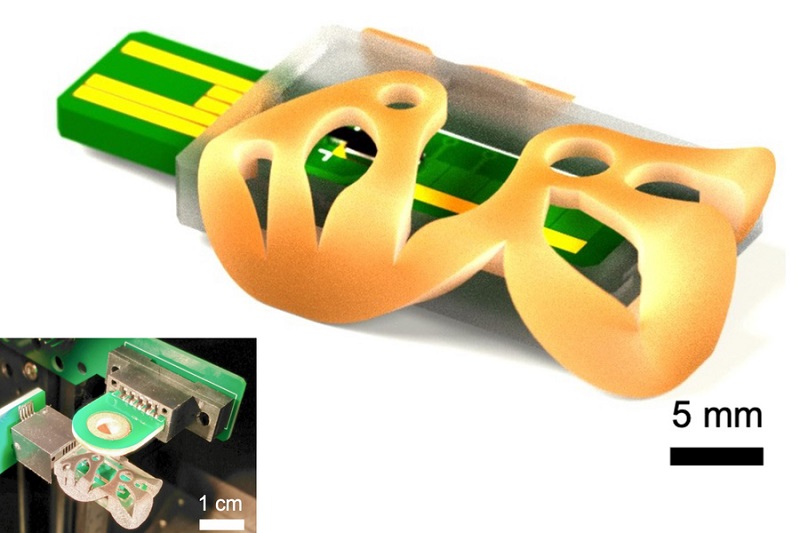
3D Printed Point-Of-Care Mass Spectrometer Outperforms State-Of-The-Art Models
Mass spectrometry is a precise technique for identifying the chemical components of a sample and has significant potential for monitoring chronic illness health states, such as measuring hormone levels... Read more.jpg)
POC Biomedical Test Spins Water Droplet Using Sound Waves for Cancer Detection
Exosomes, tiny cellular bioparticles carrying a specific set of proteins, lipids, and genetic materials, play a crucial role in cell communication and hold promise for non-invasive diagnostics.... Read more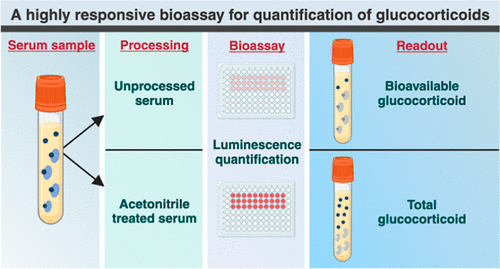
Highly Reliable Cell-Based Assay Enables Accurate Diagnosis of Endocrine Diseases
The conventional methods for measuring free cortisol, the body's stress hormone, from blood or saliva are quite demanding and require sample processing. The most common method, therefore, involves collecting... Read moreMolecular Diagnostics
view channel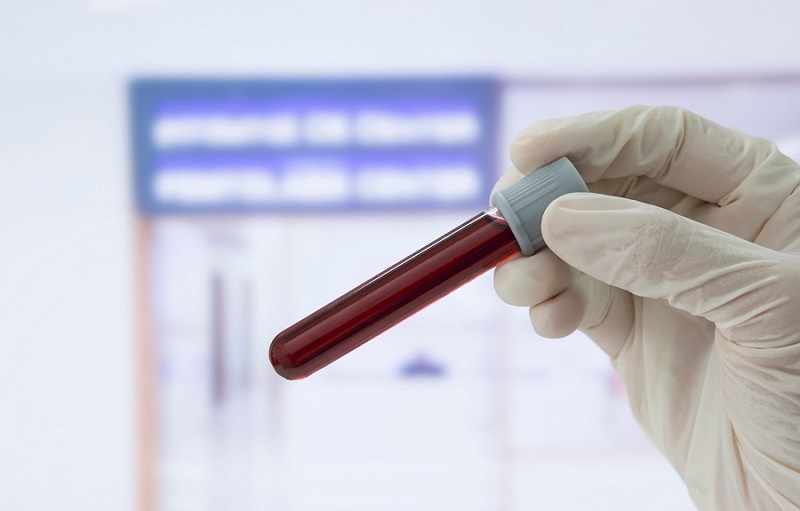
Blood Test Accurately Predicts Lung Cancer Risk and Reduces Need for Scans
Lung cancer is extremely hard to detect early due to the limitations of current screening technologies, which are costly, sometimes inaccurate, and less commonly endorsed by healthcare professionals compared... Read more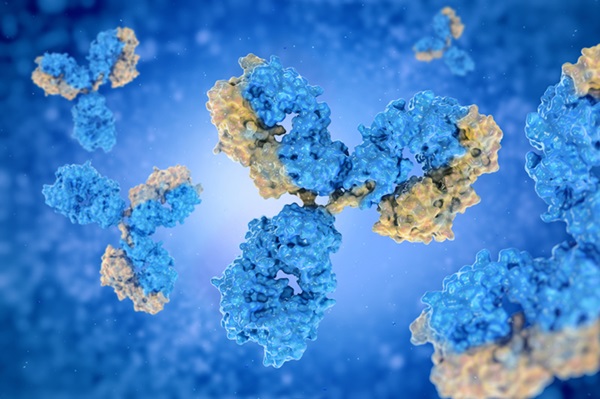
Unique Autoantibody Signature to Help Diagnose Multiple Sclerosis Years before Symptom Onset
Autoimmune diseases such as multiple sclerosis (MS) are thought to occur partly due to unusual immune responses to common infections. Early MS symptoms, including dizziness, spasms, and fatigue, often... Read more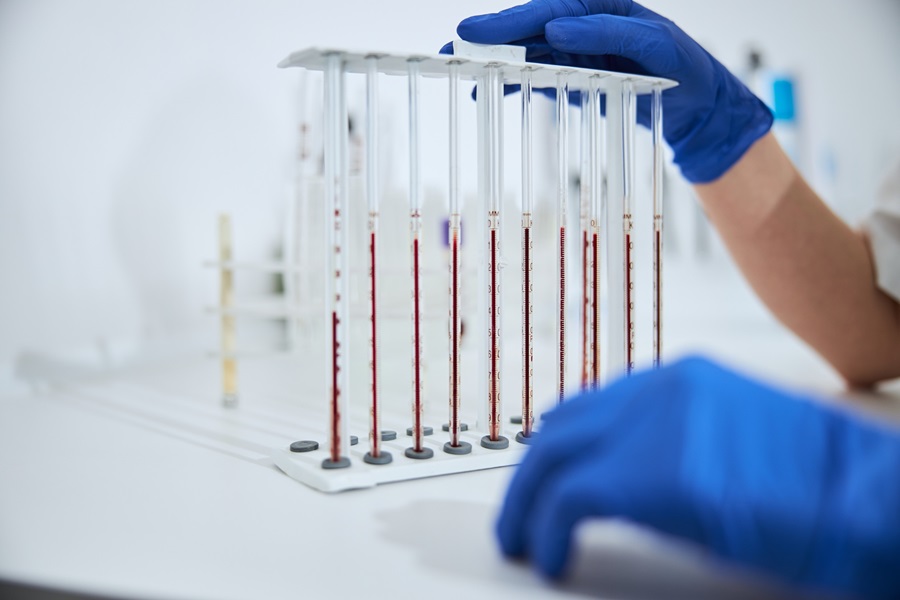
Blood Test Could Detect HPV-Associated Cancers 10 Years before Clinical Diagnosis
Human papilloma virus (HPV) is known to cause various cancers, including those of the genitals, anus, mouth, throat, and cervix. HPV-associated oropharyngeal cancer (HPV+OPSCC) is the most common HPV-associated... Read moreHematology
view channel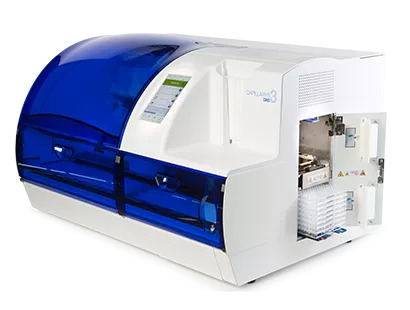
Next Generation Instrument Screens for Hemoglobin Disorders in Newborns
Hemoglobinopathies, the most widespread inherited conditions globally, affect about 7% of the population as carriers, with 2.7% of newborns being born with these conditions. The spectrum of clinical manifestations... Read more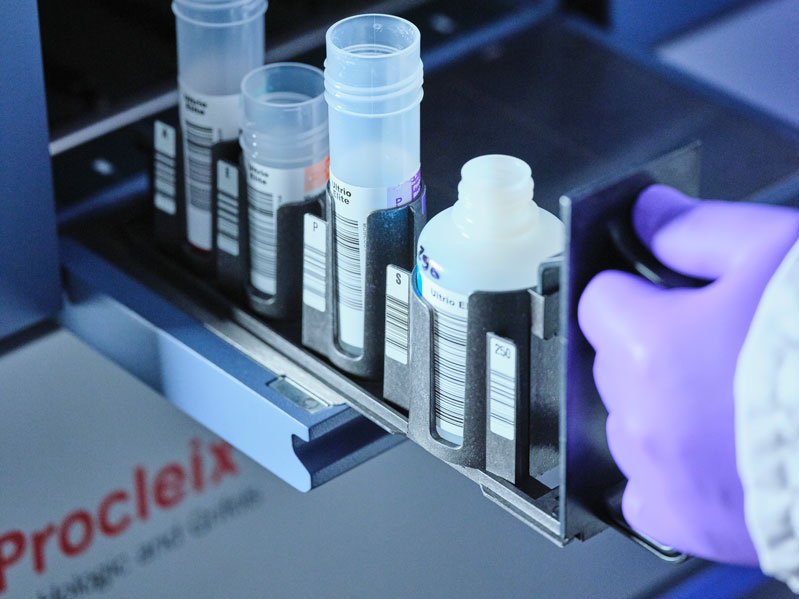
First 4-in-1 Nucleic Acid Test for Arbovirus Screening to Reduce Risk of Transfusion-Transmitted Infections
Arboviruses represent an emerging global health threat, exacerbated by climate change and increased international travel that is facilitating their spread across new regions. Chikungunya, dengue, West... Read more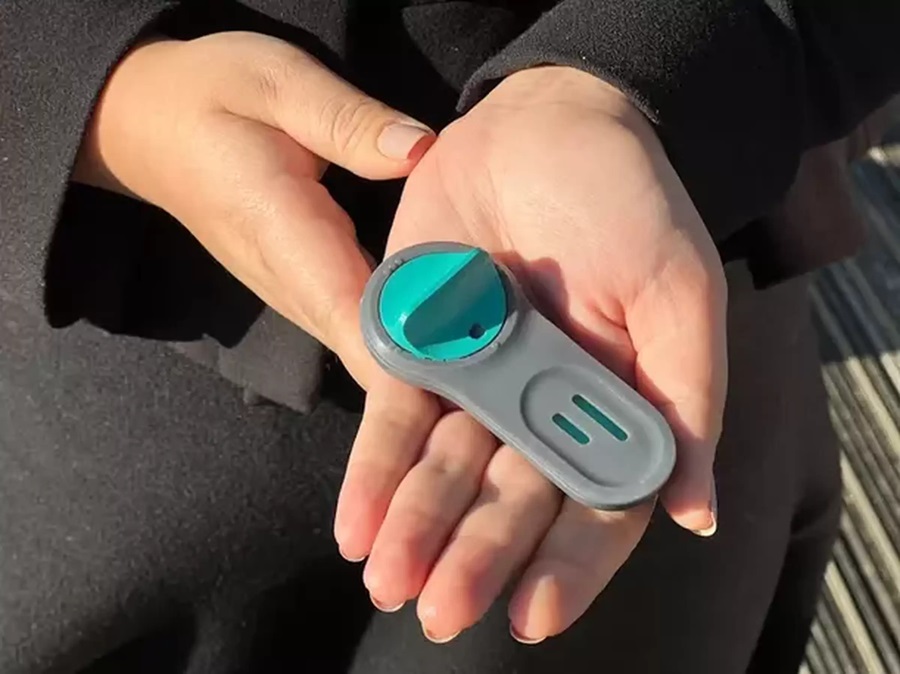
POC Finger-Prick Blood Test Determines Risk of Neutropenic Sepsis in Patients Undergoing Chemotherapy
Neutropenia, a decrease in neutrophils (a type of white blood cell crucial for fighting infections), is a frequent side effect of certain cancer treatments. This condition elevates the risk of infections,... Read more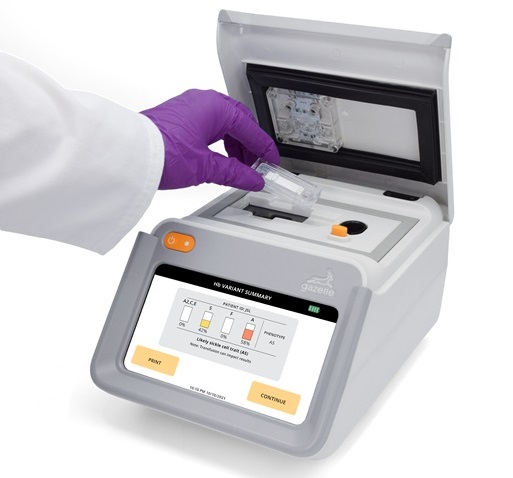
First Affordable and Rapid Test for Beta Thalassemia Demonstrates 99% Diagnostic Accuracy
Hemoglobin disorders rank as some of the most prevalent monogenic diseases globally. Among various hemoglobin disorders, beta thalassemia, a hereditary blood disorder, affects about 1.5% of the world's... Read moreImmunology
view channel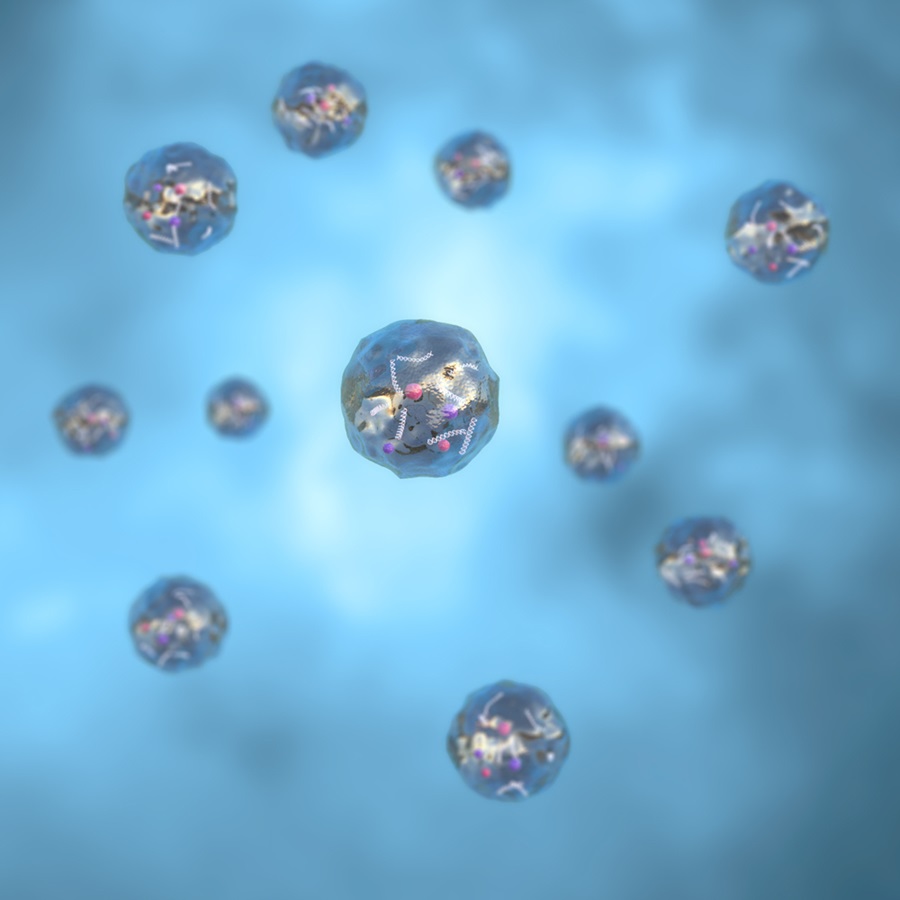
Diagnostic Blood Test for Cellular Rejection after Organ Transplant Could Replace Surgical Biopsies
Transplanted organs constantly face the risk of being rejected by the recipient's immune system which differentiates self from non-self using T cells and B cells. T cells are commonly associated with acute... Read more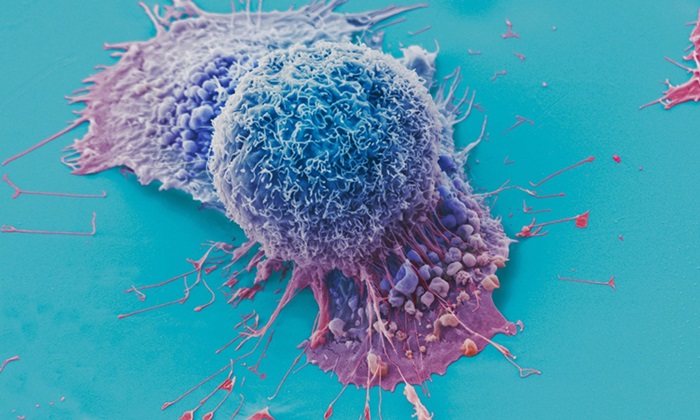
AI Tool Precisely Matches Cancer Drugs to Patients Using Information from Each Tumor Cell
Current strategies for matching cancer patients with specific treatments often depend on bulk sequencing of tumor DNA and RNA, which provides an average profile from all cells within a tumor sample.... Read more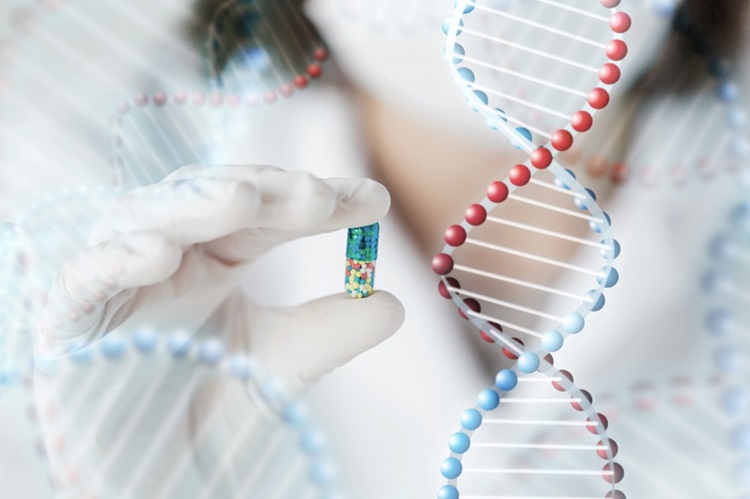
Genetic Testing Combined With Personalized Drug Screening On Tumor Samples to Revolutionize Cancer Treatment
Cancer treatment typically adheres to a standard of care—established, statistically validated regimens that are effective for the majority of patients. However, the disease’s inherent variability means... Read moreMicrobiology
view channel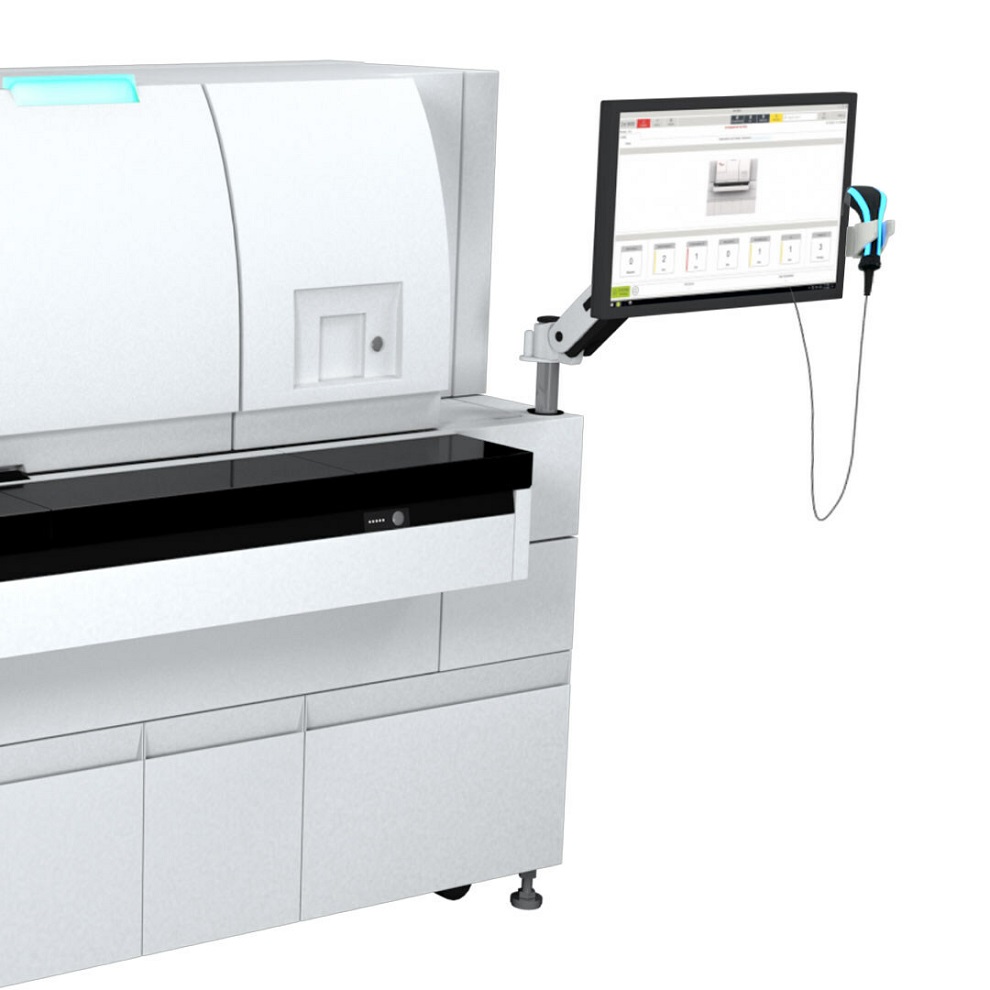
New CE-Marked Hepatitis Assays to Help Diagnose Infections Earlier
According to the World Health Organization (WHO), an estimated 354 million individuals globally are afflicted with chronic hepatitis B or C. These viruses are the leading causes of liver cirrhosis, liver... Read more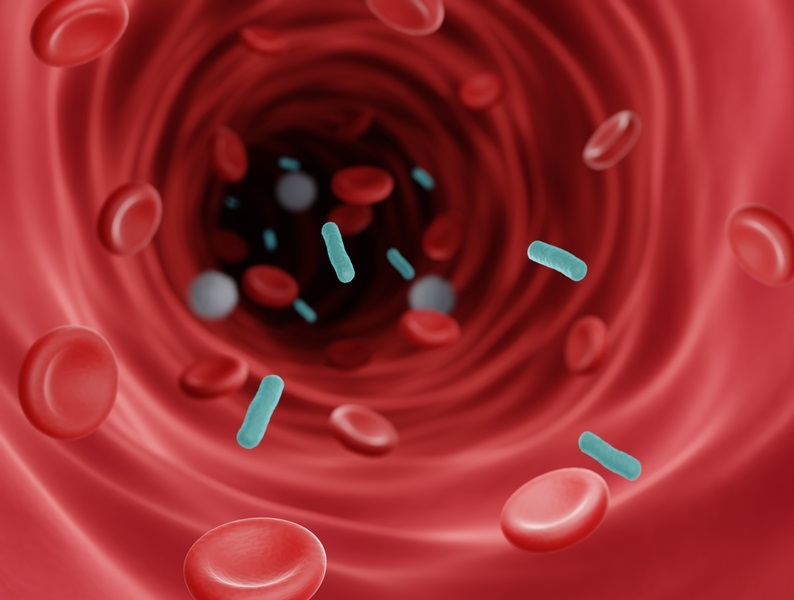
1 Hour, Direct-From-Blood Multiplex PCR Test Identifies 95% of Sepsis-Causing Pathogens
Sepsis contributes to one in every three hospital deaths in the US, and globally, septic shock carries a mortality rate of 30-40%. Diagnosing sepsis early is challenging due to its non-specific symptoms... Read moreTechnology
view channel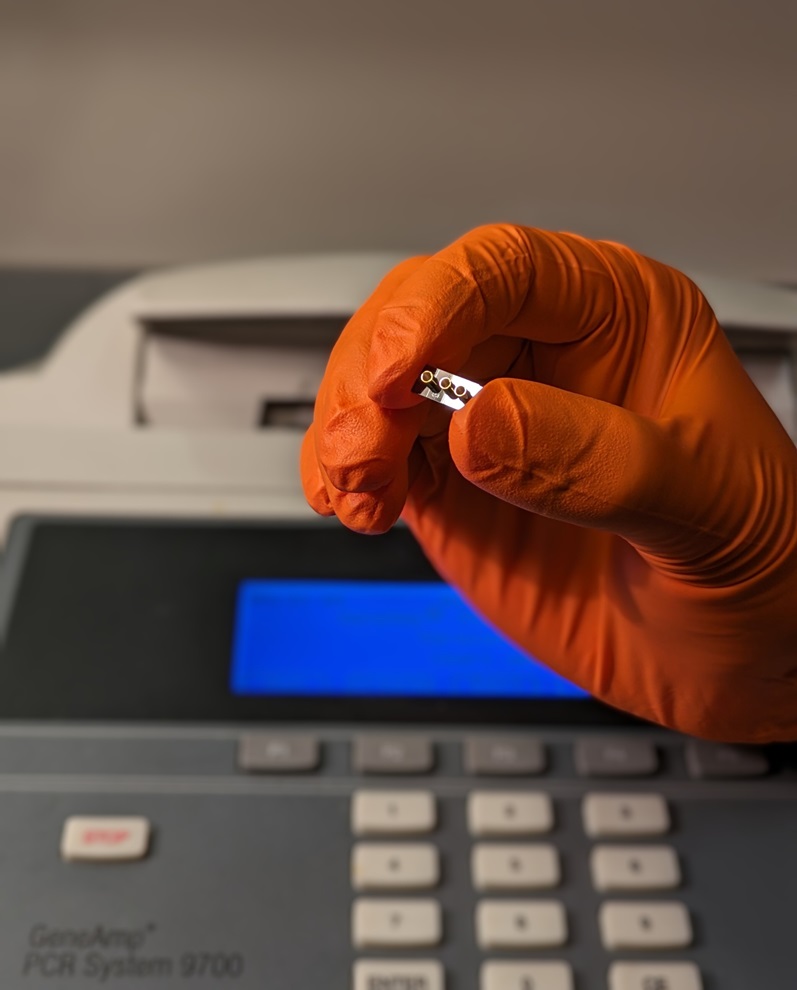
New Diagnostic System Achieves PCR Testing Accuracy
While PCR tests are the gold standard of accuracy for virology testing, they come with limitations such as complexity, the need for skilled lab operators, and longer result times. They also require complex... Read more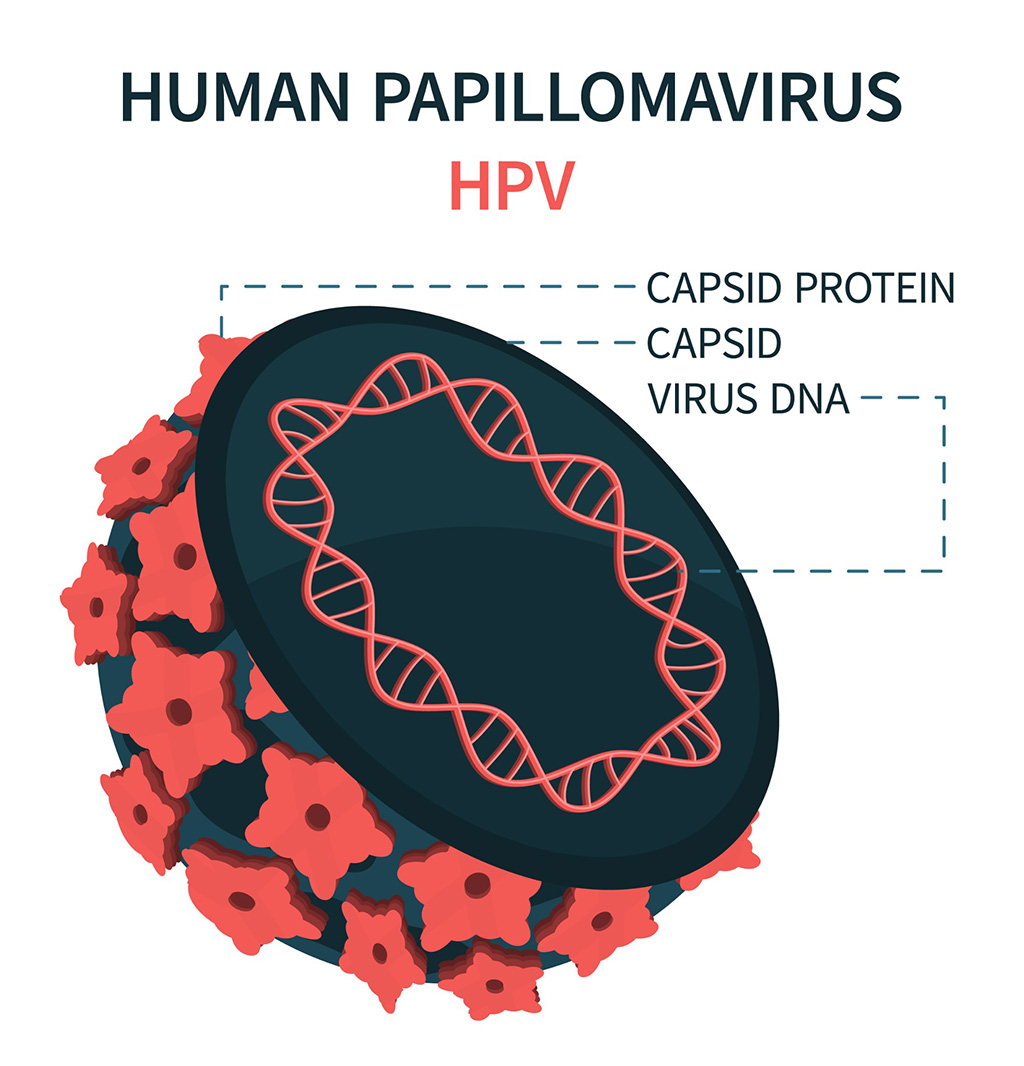
DNA Biosensor Enables Early Diagnosis of Cervical Cancer
Molybdenum disulfide (MoS2), recognized for its potential to form two-dimensional nanosheets like graphene, is a material that's increasingly catching the eye of the scientific community.... Read more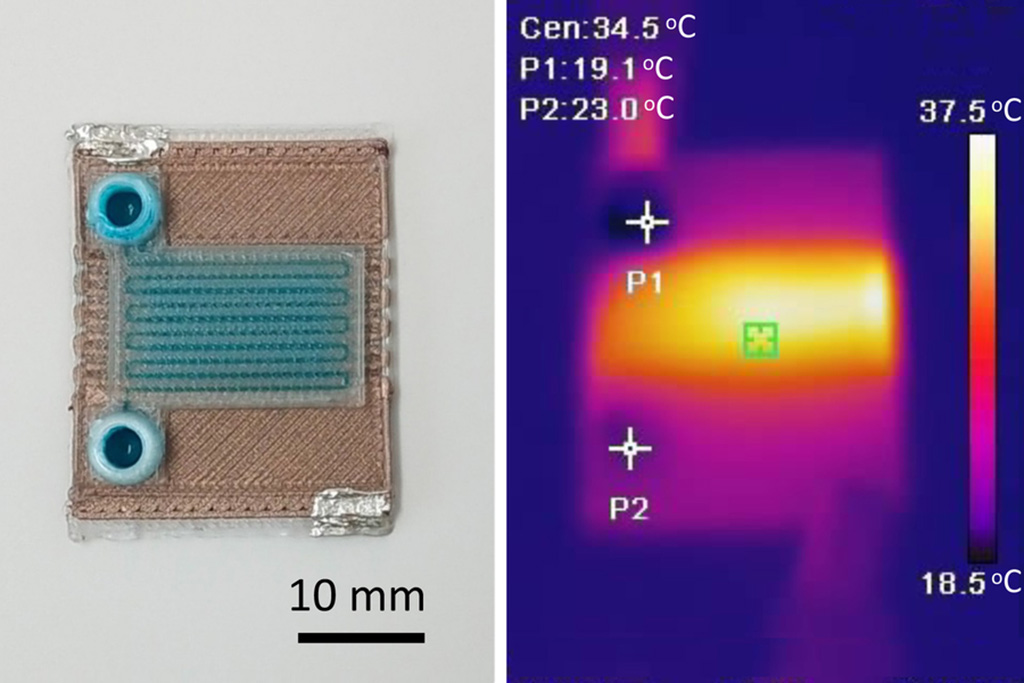
Self-Heating Microfluidic Devices Can Detect Diseases in Tiny Blood or Fluid Samples
Microfluidics, which are miniature devices that control the flow of liquids and facilitate chemical reactions, play a key role in disease detection from small samples of blood or other fluids.... Read more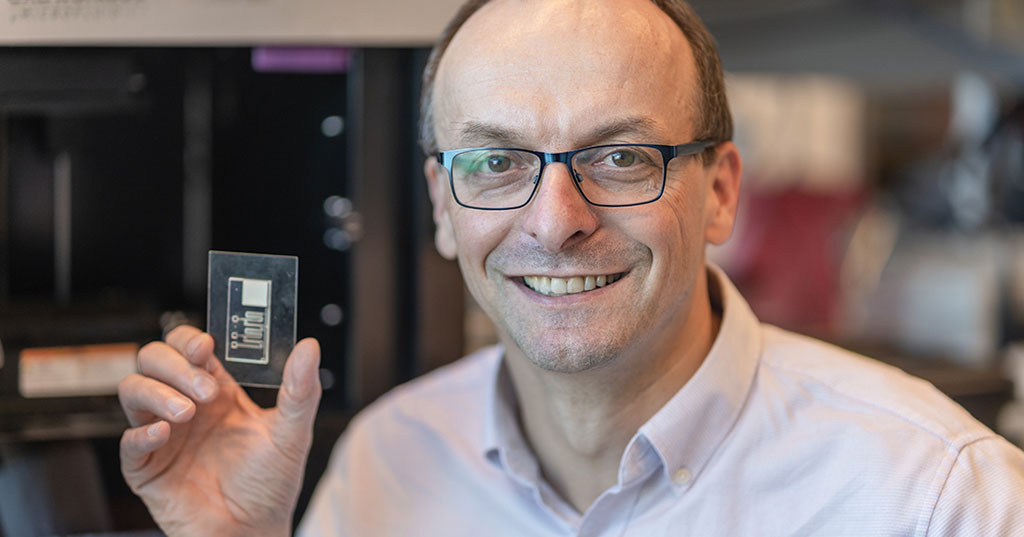
Breakthrough in Diagnostic Technology Could Make On-The-Spot Testing Widely Accessible
Home testing gained significant importance during the COVID-19 pandemic, yet the availability of rapid tests is limited, and most of them can only drive one liquid across the strip, leading to continued... Read moreIndustry
view channel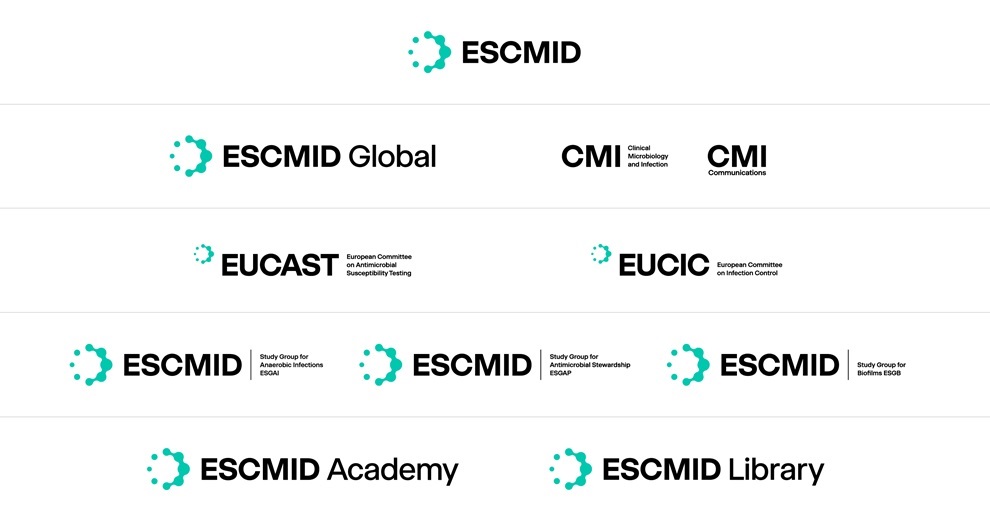
ECCMID Congress Name Changes to ESCMID Global
Over the last few years, the European Society of Clinical Microbiology and Infectious Diseases (ESCMID, Basel, Switzerland) has evolved remarkably. The society is now stronger and broader than ever before... Read more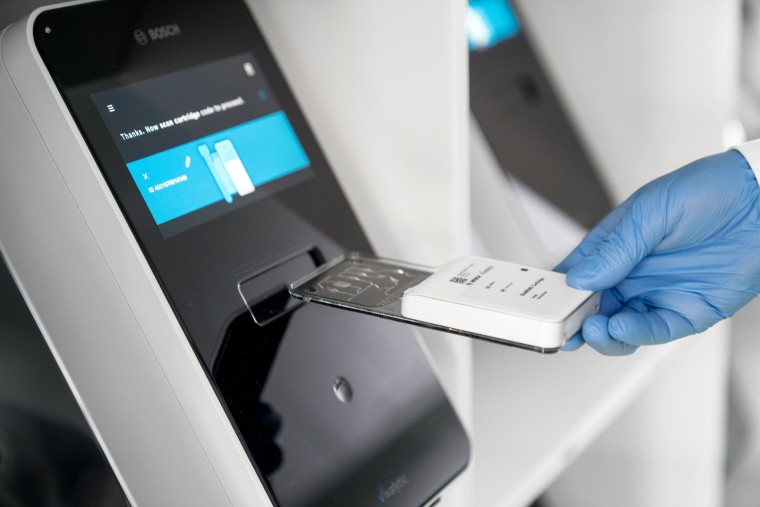
Bosch and Randox Partner to Make Strategic Investment in Vivalytic Analysis Platform
Given the presence of so many diseases, determining whether a patient is presenting the symptoms of a simple cold, the flu, or something as severe as life-threatening meningitis is usually only possible... Read more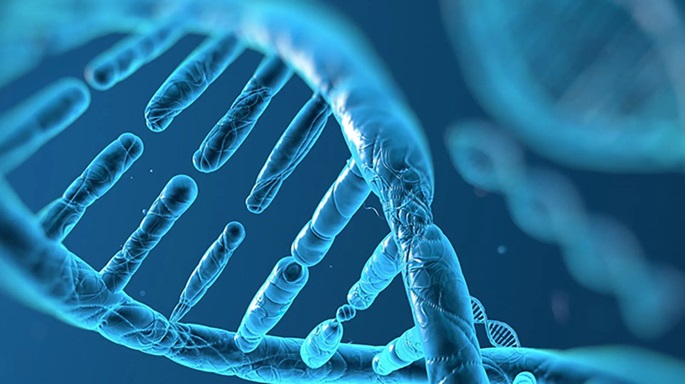