Neural Network Recognizes Breast Cancer on Histological Samples With 100% Accuracy
Posted on 02 Feb 2024
The likelihood of a favorable outcome for a breast cancer patient is greatly influenced by the stage at which the cancer is diagnosed. Histological examination is the benchmark for diagnosis, but its reliability can be affected by subjective interpretations and the quality of the tissue sample. Inaccuracies in these examinations can lead to incorrect diagnoses. Now, a team of mathematicians has developed a machine learning model that significantly enhances the accuracy of identifying cancer in histological images. The highlight of this model is the incorporation of an additional module that boosts the neural network's "attention" capability, enabling it to achieve near-perfect accuracy.
The mathematicians at RUDN University (Moscow, Russia) conducted tests on several convolutional neural networks and supplemented them with two convolutional attention modules. These modules are crucial for detecting objects within images. The model underwent training and testing using the BreakHis dataset, which comprises nearly 10,000 histological images at various scales, sourced from 82 patients. The most impressive performance came from a model that combined the DenseNet211 convolutional network with the attention modules, achieving a remarkable accuracy rate of 99.6%. The research team noted that the detection of cancerous formations is affected by image scale. This is because images differ in quality at various zoom levels, and cancerous formations appear differently. Therefore, during practical application, selecting the appropriate scale for image analysis must be a critical consideration.
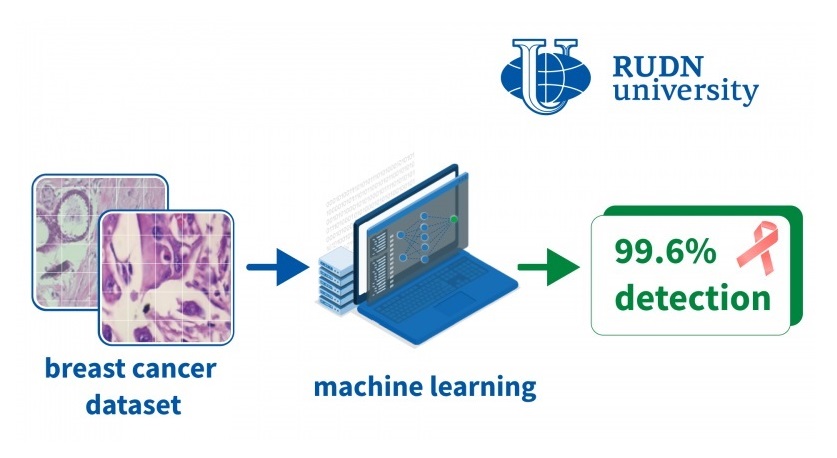
“Computer classification of histological images will reduce the burden on doctors and increase the accuracy of tests. Such technologies will improve the treatment and diagnosis of breast cancer. Deep learning methods have shown promising results in medical image analysis problems in recent years,” said Ammar Muthanna, Ph.D., Director of the Scientific Center for Modeling Wireless 5G Networks at RUDN University. “The attention modules in the model improved feature extraction and the overall performance of the model. With their help, the model focused on significant areas of the image and highlighted the necessary information. It shows the importance of attention mechanisms in the analysis of medical images.”
Related Links:
RUDN University