AI Tool Beats Expert Pathologists in Predicting Breast Cancer Outcomes
Posted on 11 Dec 2023
Pathologists traditionally assess cancerous tissues in patients to guide treatment strategies. This evaluation, known as grading, examines the appearance of cancer cells and is crucial in determining a patient's treatment plan. This grading process, focused on the morphology of cancer cells, has been a standard practice for many years. Now, a study has found that a new artificial intelligence (AI) tool outperforms expert pathologists in predicting the progression of a patient's disease based on tissue evaluations.
Developed by researchers at Northwestern University (Evanston, IL, USA), this AI tool offers the potential to significantly alter the treatment path for breast cancer patients. The tool's ability to more accurately identify those patients currently categorized as high or intermediate risk but who will have a favorable long-term prognosis could lead to less aggressive chemotherapy treatments. This advancement is particularly significant given the adverse side effects associated with chemotherapy, such as nausea and potential heart damage. Previous studies have highlighted the role of non-cancerous cells, including immune cells and structural tissue cells, in cancer progression. This AI tool is pioneering in its comprehensive assessment of both cancerous and non-cancerous elements in invasive breast cancer.
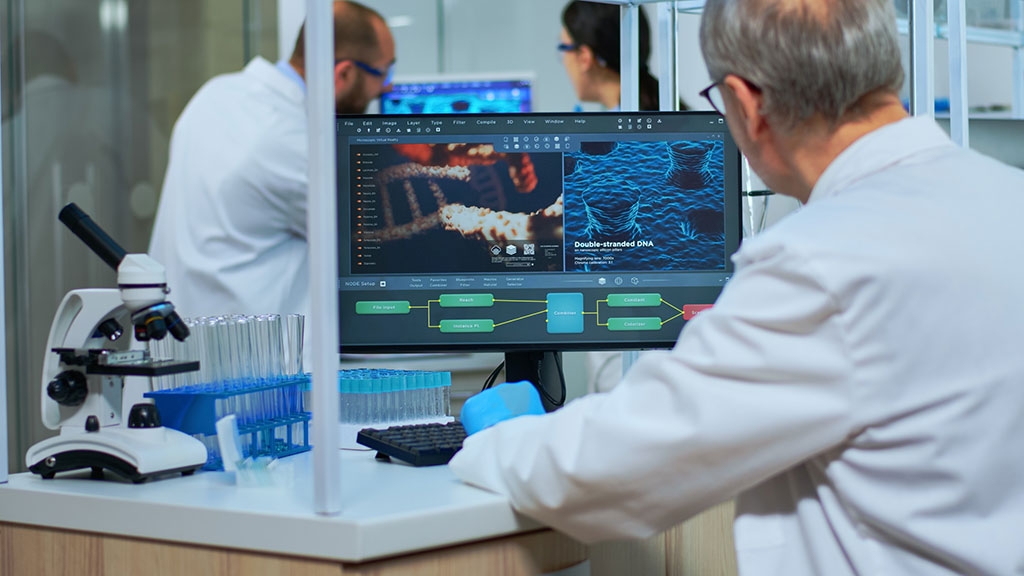
To train this AI model, the researchers utilized a large-scale, international collaboration of medical students and pathologists. This network provided extensive human-generated annotations of cells and tissue structures within digital images of breast cancer tissues, collected through a website over several years. The AI system evaluates 26 different aspects of breast tissue, producing an overall prognostic score. The system also generates individual scores for the cancer, immune and stromal cells to explain the overall score to the pathologist. For example, in some patients, a favorable prognosis score may be due to properties of their immune cells, where for others it may be due to properties of their cancer cells. This information could be used by a patient’s care team in creating an individualized treatment plan.
Adoption of the new model could provide patients diagnosed with breast cancer with a more accurate estimate of the risk associated with their disease, empowering them to make informed decisions about their clinical care, according to the researchers. Additionally, this model may help in assessing therapeutic response, allowing treatment to be escalated or de-escalated depending on how the microscopic appearance of the tissue changes over time. For example, the tool may be able to recognize the effectiveness of a patient’s immune system in targeting cancer during chemotherapy, which could be used to reduce the duration or intensity of chemotherapy. Next, the scientists will evaluate this model prospectively to validate it for clinical use. The scientists also are working to develop models for more specific types of breast cancers like triple-negative or HER2-positive. Invasive breast cancer encompasses several different categories, and the important tissue patterns may vary across these categories.
“Our study demonstrates the importance of non-cancer components in determining a patient’s outcome,” said corresponding study author Lee Cooper, associate professor of pathology at Northwestern University Feinberg School of Medicine. “The importance of these elements was known from biological studies, but this knowledge has not been effectively translated to clinical use.”
“These patterns are challenging for a pathologist to evaluate as they can be difficult for the human eye to categorize reliably,” said Cooper, also a member of the Robert H. Lurie Comprehensive Cancer Center of Northwestern University. “The AI model measures these patterns and presents information to the pathologist in a way that makes the AI decision-making process clear to the pathologist.”
Related Links:
Northwestern University