Chromosome Interaction Profiling Reveals Cancer Risk Genes
By LabMedica International staff writers
Posted on 29 Mar 2018
Breast cancer is a heterogeneous disease with two main subtypes defined by the presence (ER+) or absence (ER−) of the estrogen receptor. Approximately 80% of newly diagnosed breast cancers are ER+ although this proportion varies with age at diagnosis and ethnicity.Posted on 29 Mar 2018
Genome-wide association studies (GWAS) coupled with large-scale replication and fine-mapping studies have led to the identification of approximately 100 breast cancer risk loci. Potential target and survival-related genes in breast cancer have been identified using chromatin interaction profiles to map parts of the genome previously implicated in risk of the disease.
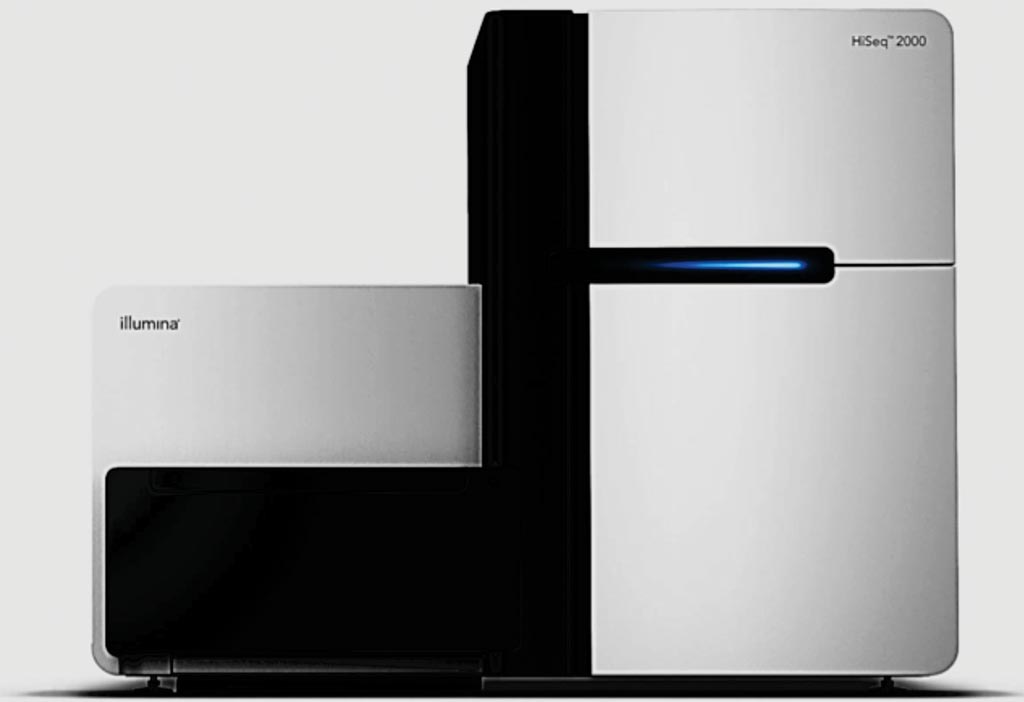
Image: The HiSeq2000 instrument used for whole-genome sequencing targeted resequencing, gene expression level detection, DNA methylation profiling, de novo sequencing, metagenomic studies, and ChiP-seq (Photo courtesy of Illumina).
A team of scientists led by those at the Institute of Cancer Research (London, UK) used a long-range interaction profiling technique called Capture Hi-C (CHi-C) to investigate dozens of regions previously implicated in breast cancer risk. Based on CHi-C data for four breast cancer cell lines with or without enhanced estrogen receptor (ER) activity, a cancer-free breast epithelial cell line, and a cell line from another tissue type, they focused in on 110 potential target genes at 33 of the risk loci.
By adding in related risk single nucleotide polymorphism (SNP) data, RNA sequence profiles, and somatic mutation data reported previously, the team looked at overlap between these proposed targets, expression quantitative trait loci (eQTL), and genes prone to mutation in breast cancer tumors. With the help of gene expression and outcome data from the Metabric breast cancer cohort, meanwhile, the authors highlighted 32 genes with ties to breast cancer survival time. After using HiSeq2000 instruments to sequence target-enriched Hi-C libraries, the team tallied the CHi-C interaction peaks at each risk locus. A dozen risk loci lacked discernable interaction peaks, leaving interaction peaks at 51 loci for further consideration within and across the six cell lines.
The team uncovered potential gene targets at 33 of the breast cancer-associated sites, spanning 94 protein-coding and 16 non-coding RNA gene targets neighboring the risk loci or falling more far afield. They subsequently considered the target genes alongside eQTLs (informed by RNA sequence data from the Cancer Genome Atlas) and somatic mutation profiles identified in hundreds of breast cancer genome sequences, which supported the notion that CHi-C can help unearth authentic target genes.
The authors concluded that a high-throughput CHi-C analysis can contribute to on-going efforts to functionally annotate GWAS risk loci and that CHi-C target genes that are supported by additional data sources are strong candidates for in-depth functional follow-up studies. The study was published on March 12, 2018, in the journal Nature Communications.
Related Links:
Institute of Cancer Research