Images Identified for Breast Cancer Cell Histopathology
By LabMedica International staff writers
Posted on 11 Nov 2016
Breast cancer is the most prevalent form of cancer for women worldwide. Current breast cancer clinical practice and treatment mainly relies on the evaluation of the disease's prognosis using the Bloom-Richardson grading system.Posted on 11 Nov 2016
The advent of digital pathology and fast digital slide scanners has opened the possibility of automating the prognosis by applying image-processing methods and while this undoubtedly represents progress, image-processing methods have struggled to analyze high-grade breast cancer cells as these cells are often clustered together and have vague boundaries, which make successful detection extremely challenging.
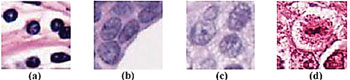
Image: Photomicrographs of a) Lymphocytes, b) Normal epithelial nuclei, c) Cancerous epithelial nuclei, and d) Mitotic nuclei (Photo courtesy of Trinity College Dublin).
An international team comprising engineers, mathematicians and doctors led by those at Trinity College Dublin (Ireland) have has applied a technique used for detecting damage in underwater marine structures to identify cancerous cells in breast cancer histopathology images. The team has proposed a novel segmentation algorithm for detecting individual nuclei from hematoxylin and eosin (H&E) stained breast histopathology images. This detection framework estimates a nuclei saliency map using tensor voting followed by boundary extraction of the nuclei on the saliency map using a Loopy Back Propagation (LBP) algorithm on a Markov Random Field (MRF).
The method was tested on both whole-slide images and frames of breast cancer histopathology images. The investigators considered the likelihood of every point in a histopathology image either being near a cell center or a cell boundary and using a belief propagation algorithm, the most suitable cell boundaries were then traced out. Test results show that the proposed method is suitable for nuclei segmentation in high-grade breast cancer histopathology images containing scenes depicting grade 3 nuclear pleomorphism (cancerous nuclei with marked variations from normal nuclei) even though these are quite challenging for traditional segmentation methods to detect.
Maqlin Paramanandam, PhD, the lead author of the study said, “The potential for this technology is very exciting and we are delighted that this international and inter-disciplinary team has worked so well at tackling a real bottle-neck in automating the diagnosis of breast cancer using histopathology images.” The study was published on September 20, 2016, in the journal Public Library of Science ONE.
Related Links:
Trinity College Dublin