Artificial Intelligence Achieves Near-Human Performance in Image Analysis
By LabMedica International staff writers
Posted on 27 Jun 2016
A research team has developed an artificial intelligence (AI) method that trains computers to interpret pathology images. In a recent test of the method developed to examine images of lymph nodes, human complemented with computer analyses together identified breast cancer with 99.5% accuracy.Posted on 27 Jun 2016
The method was put to the test in a “challenge” competition held at the 2016 annual meeting of the International Symposium of Biomedical Imaging (ISBI; April 2016, Prague, Czech Republic). “Identifying the presence or absence of metastatic cancer in a patient’s lymph nodes is a routine and critically important task for pathologists,” said team leader Prof. Andrew Beck, MD, PhD, pathologist, Beth Israel Deaconess Medical Center (BIDMC; Boston, MA, USA) and Harvard Medical School (HMS; Boston, MA, USA), “Peering into the microscope to sift through millions of normal cells to identify just a few malignant cells can prove extremely laborious using conventional methods. We thought this was a task that the computer could be quite good at – and that proved to be the case.”
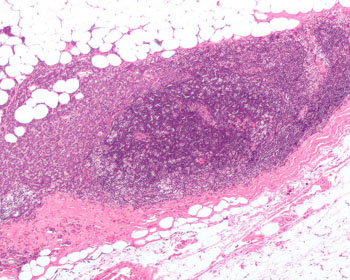
Image: A micrograph showing a lymph node invaded by ductal breast carcinoma, with extension of the tumor beyond the lymph node. H&E stain. The dark purple (center) is lymphocytes (part of a normal lymph node). Surrounding the lymphocytes and extending into the surrounding fat (top of image) is ductal breast carcinoma (Photo courtesy of Michael Bonert / Wikimedia).
“Our AI method is based on deep learning, a machine-learning algorithm used for a range of applications including speech recognition and image recognition,” explained Prof. Beck, “This approach teaches machines to interpret the complex patterns and structure observed in real-life data by building multi-layer artificial neural networks, in a process which is thought to show similarities with the learning process that occurs in layers of neurons in the brain’s neocortex, the region where thinking occurs.”
In an objective evaluation in which researchers were given slides of lymph node cells and asked to determine whether or not they contained cancer, the team’s automated diagnostic method was accurate approximately 92% of the time. “This nearly matched the success rate of a human pathologist, whose results were 96% accurate,” said Aditya Khosla of MIT, who with Prof. Beck recently formed a start-up company, PathAI, to further develop AI technology for pathology.
“The truly exciting thing was when we combined the pathologist’s analysis with our automated computational diagnostic method, the result improved to 99.5% accuracy,” said Prof. Beck, “Combining these two methods yielded a major reduction in errors.”
The team trained the computer to distinguish between cancerous tumor regions and normal regions based on a deep multilayer convolutional network. “In our approach, we started with hundreds of training slides for which a pathologist has labeled regions of cancer and regions of normal cells,” said Dayong Wang, PhD, “We then extracted millions of these small training examples and used deep learning to build a computational model to classify them.” They then identified the specific training examples for which the computer is prone to making mistakes and re-trained the computer using greater numbers of the more difficult training examples. In this way, the computer’s performance continued to improve.
Only recently “improved scanning, storage, processing, and algorithms have made it possible to pursue this mission effectively. Our results in the ISBI competition show that what the computer is doing is genuinely intelligent and that the combination of human and computer interpretations will result in more precise and more clinically valuable diagnoses to guide treatment decisions,” said Prof. Beck.
Jeroen van der Laak, PhD, who leads a digital pathology research group at Radboud University Medical Center in the Netherlands and was an organizer for the competition, said, “When we started this challenge, we expected some interesting results. The fact that computers had almost comparable performance to humans is way beyond what I had anticipated. It is a clear indication that AI is going to shape the way we deal with histopathological images in the years to come.”
The team described their method in a technical report posted June 2016 in the arXiv.org repository.
Related Links:
Beth Israel Deaconess Medical Center
Harvard Medical School