AI Tool Merges Patient Data with Blood Test Results to Detect Heart Failure
Posted on 20 Jun 2022
Acute heart failure is a life-threatening condition caused when the heart is suddenly unable to pump blood around the body. It affects millions of people and accounts for a large percentage of all unplanned hospital admissions. Diagnosis is difficult because symptoms, such as shortness of breath and leg swelling, occur in many other illnesses. Previous research has shown that patients who are diagnosed quickly benefit the most from treatment. Now, research suggests that using artificial intelligence (AI) can help diagnose acute heart failure with more accuracy than current blood tests alone.
The research conducted by The University of Edinburgh (Edinburgh, UK) found that using AI to combine patient data with results from a test for levels of a protein made by the heart could help doctors spot heart failure sooner and improve patient care. Researchers combined data from 10,369 patients with suspected acute heart failure to develop a tool - called CoDE-HF – to inform clinicians’ decisions. CoDE-HF uses AI to combine routinely collected patient information with results from a blood test for the heart protein NT-proBNP to produce an estimate of whether they suffered heart failure. The current recommended diagnosis method is to test to see if levels of NT-proBNP are below a certain cut-off value, but this is not widely used as levels can vary depending on an individual’s age, weight and other health conditions.
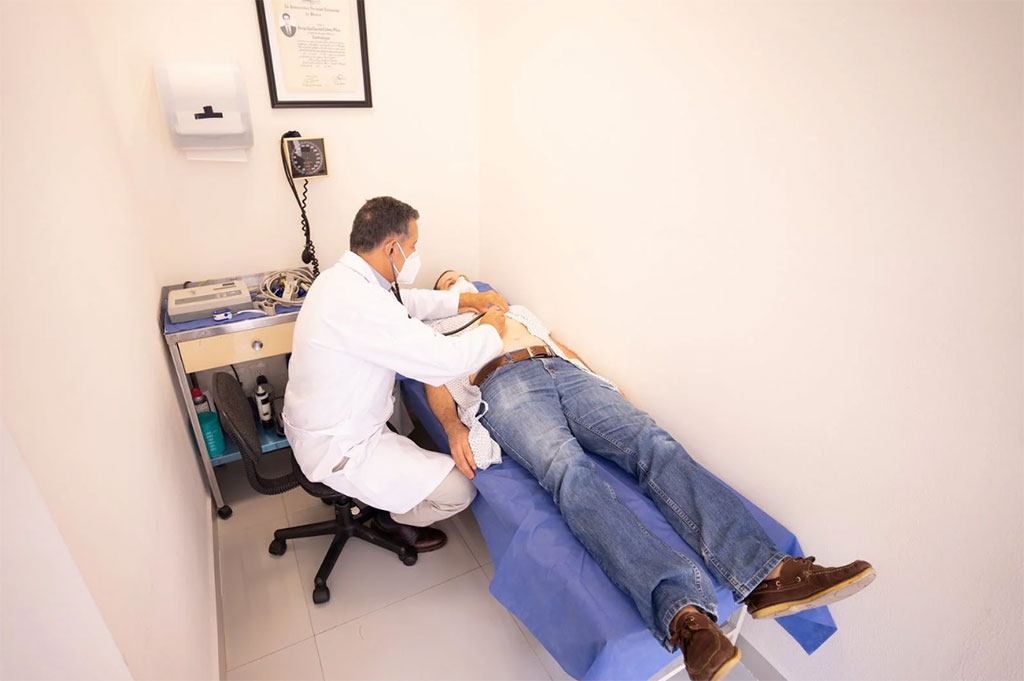
As well as spotting acute heart failure more accurately than heart protein blood tests on their own, CoDE-HF was especially precise in difficult to diagnose patient groups - such as older people and those with pre-existing medical conditions. The team is currently conducting further studies to understand how this decision-support tool will work in the hospital environment and influence patient outcomes.
“Heart failure can be a very challenging diagnosis to make in practice. We have shown that CoDE-HF, our decision-support tool, can substantially improve the accuracy of diagnosing heart failure compared to current blood tests,” said Dr. Ken Lee, cardiology specialist registrar and clinical lecturer at the University of Edinburgh.
“Our study demonstrates that the application of artificial intelligence in healthcare has major potential to help doctors deliver more personalized patient care,” added Dimitrios Doudesis, research fellow and data scientist at the University of Edinburgh.
“The application of artificial intelligence in decision-support tools as CoDE-HF to deliver more personalized care is particularly important given our ageing patient population who are living longer with more pre-existing medical conditions. We are currently conducting further studies to identify ways to implement CoDE-HF effectively in routine care,” stated Professor Nicholas Mills, British Heart Foundation professor of cardiology at the University of Edinburgh and consultant cardiologist.
Related Links:
The University of Edinburgh