Leukocyte Epigenomics and Artificial Intelligence Predict Late-Onset Alzheimer’s Disease
By LabMedica International staff writers
Posted on 12 Apr 2021
Alzheimer’s Disease (AD) is the most common form of age-related dementia, accounting for 60%–80% of such cases. The disorder causes a wide range of significant mental and physical disabilities, with profound behavioral changes and progressive impairment of social skills.Posted on 12 Apr 2021
AD is a complex disorder influenced by environmental and genetic factors. Genome-wide association studies (GWAS) have identified several late-onset AD (LOAD)-associated risk loci proliferation in peripheral blood leukocytes including in T-lymphocytes, B-lymphocytes, polymorphonuclear leucocytes, monocytes, and macrophages have been reported.
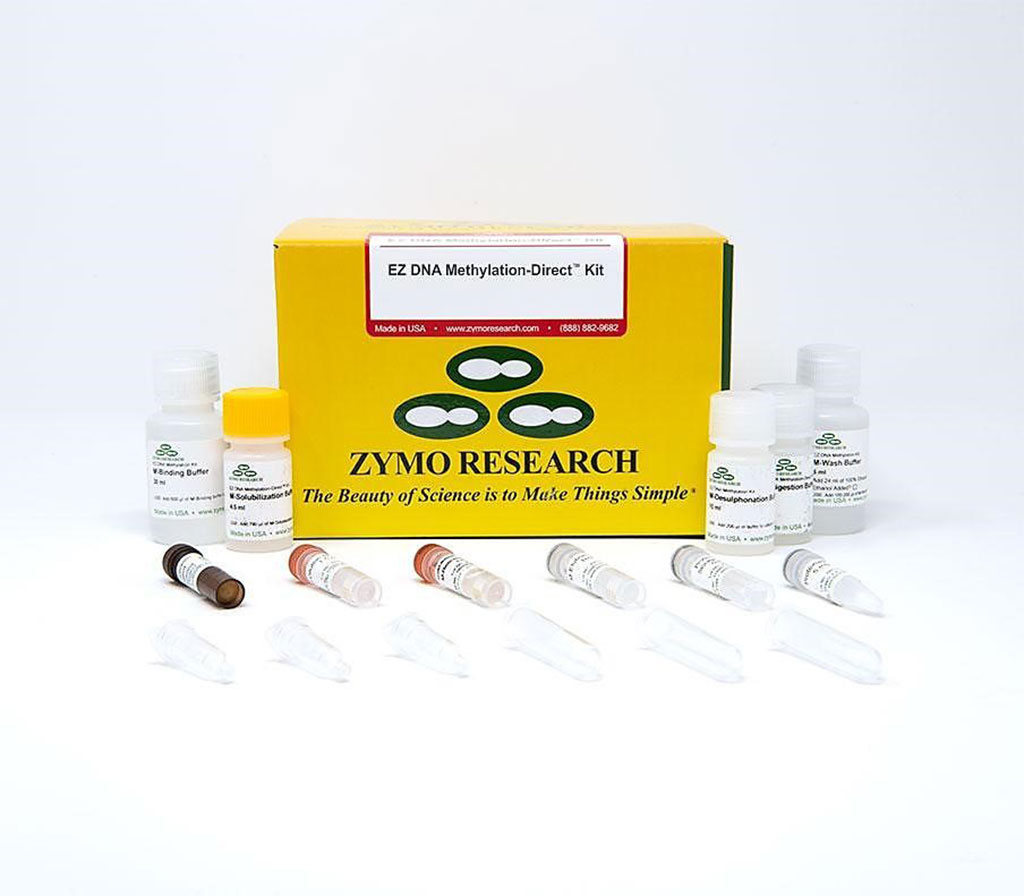
Image: The EZ DNA Methylation-Direct Kit (Photo courtesy of Zymo Research)
A team of Medical Scientists mainly from the Oakland University-William Beaumont School of Medicine (Royal Oak, MI, USA) evaluated the utility of leucocyte epigenomic-biomarkers for Alzheimer’s Disease (AD) detection and elucidated its molecular pathogeneses. The team studied blood samples from two dozen Alzheimer's disease patients and the same number of cognitively health controls.
Approximately 500 ng of genomic DNA was extracted from each of the 48 samples, which subsequently were bisulfite converted using the EZ DNA Methylation-Direct Kit (Zymo Research, Orange, CA, USA). They performed genome-wide DNA methylation analysis of the blood samples using Infinium MethylationEPIC BeadChip array (Illumina, San Diego, CA, USA). Artificial Intelligence (AI) analysis was performed using a combination of CpG sites from different genes. They also used six artificial intelligences approaches to analyze their dataset, including support vector machine, random forest, and deep learning. Deep learning is a branch of machine learning that aims to mimic the neural networks of animal brains.
The team reported that each of the AI approaches could predict Alzheimer's disease with high accuracy, yielding areas under the curve (AUC) of at least 0.93. Deep learning further improved upon that with an AUC of 0.99 and a sensitivity and specificity of 97% using intragenic markers. Similar results could be reached with intergenic markers, as well. The group noted that the addition of conventional clinical predictors or mental state analyses did not further improve performance. The analysis highlighted a number of genes and pathways known to be disrupted in Alzheimer's disease. Epigenetically altered genes included, for instance, CR1L and CTSV, which are involved in the morphology of the cerebral cortex, as well as S1PR1 and LTB4R, which are involved in inflammatory response.
Ray O. Bahado-Singh, MD, a Professor of Obstetrics and Gynecology and lead author of the study, said, “We found that the genetic analysis accurately predicted the absence or presence of Alzheimer's, allowing us to read what is going on in the brain through the blood. The results also gave us a readout of the abnormalities that are causing Alzheimer's disease. This has future promise for developing targeted treatment to interrupt the disease process.” The study was published on March 31, 2021 in the journal PLOS ONE.
Related Links:
Oakland University-William Beaumont School of Medicine
Zymo Research
Illumina