Microscope-Based AI Identifies Bacteria Accurately
By LabMedica International staff writers
Posted on 26 Dec 2017
Microscopes enhanced with artificial intelligence (AI) could help clinical microbiologists diagnose potentially deadly blood infections and improve patients' odds of survival.Posted on 26 Dec 2017
Scientists have demonstrated that an automated AI-enhanced microscope system is "highly adept" at identifying images of bacteria quickly and accurately. The automated system could help alleviate the current lack of highly trained microbiologists, expected to worsen as 20% of technologists reach retirement age in the next five years.
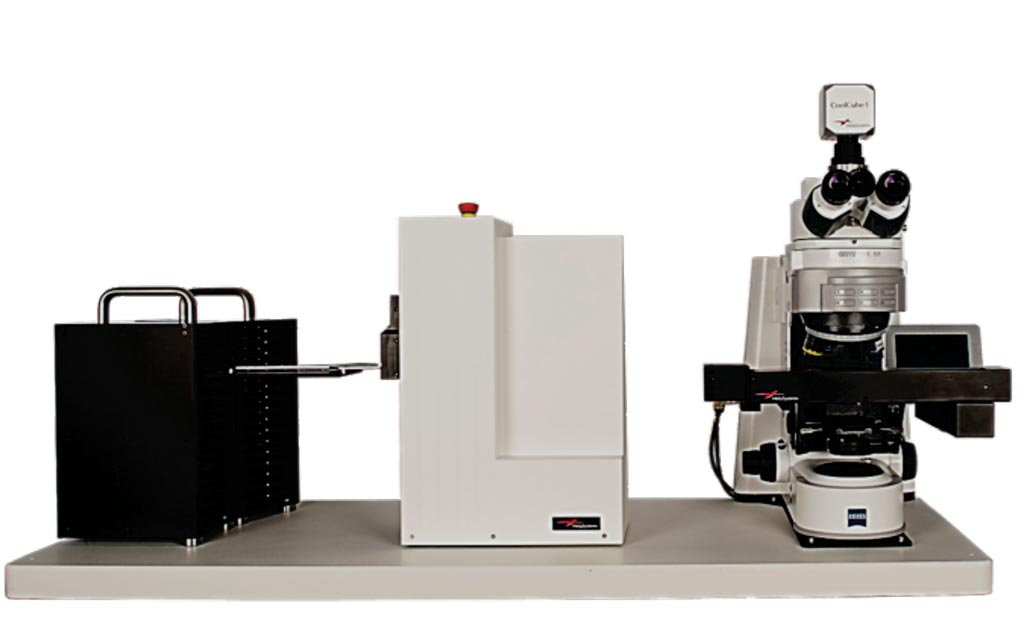
Image: The MetaFer Slide Scanning and Imaging platform with a Zeiss microscope (Photo courtesy of MetaSystems Group).
Scientists working with the Department of Pathology, Beth Israel Deaconess Medical Center, (Boston, MA, USA) used an automated microscope designed to collect high-resolution image data from microscopic slides. In this case, blood samples taken from patients with suspected bloodstream infections were incubated to increase bacterial numbers. Then, slides were prepared by placing a drop of blood on a glass slide and stained with dye to make the bacterial cell structures more visible.
The investigators then trained a convolutional neural network (CNN), a class of artificial intelligence modeled on the mammalian visual cortex and used to analyze visual data, to categorize bacteria based on their shape and distribution. These characteristics were selected to represent bacteria that most often cause bloodstream infections; the rod-shaped bacteria including Escherichia coli; the round clusters of Staphylococcus species; and the pairs or chains of Streptococcus species. All slides were imaged without coverslips using a MetaFer Slide Scanning and Imaging platform with a 140-slide capacity automated slide loader equipped with a 40× magnification Plan-Neofluar objective.
To train it, the scientists fed their unschooled neural network more than 25,000 images from blood samples prepared during routine clinical workups. By cropping these images, in which the bacteria had already been identified by human clinical microbiologists, the scientists generated more than 100,000 training images. The machine intelligence learned how to sort the images into the three categories of bacteria (rod-shaped, round clusters, and round chains or pairs), ultimately achieving nearly 95% accuracy.
The team challenged the algorithm to sort new images from 189 slides without human intervention. Overall, the algorithm achieved more than 93% accuracy in all three categories. Sensitivity/specificity was 98.4/75.0% for Gram-positive cocci in chains/pairs; 93.2/97.2% for Gram-positive cocci in clusters; and 96.3/98.1% for Gram-negative rods. The study was published on November 29, 2017, in the Journal of Clinical Microbiology.
Related Links:
Beth Israel Deaconess Medical Center