Rapid Method Developed to Identify Bacteria in Blood Samples
By LabMedica International staff writers
Posted on 23 Feb 2017
In clinical diagnostics and pathogen detection, profiling of complex samples for low-level genotypes represents a significant challenge. Advances in speed, sensitivity, and extent of multiplexing of molecular pathogen detection assays are needed to improve patient care.Posted on 23 Feb 2017
A desktop diagnosis tool has been developed that detects the presence of harmful bacteria in a blood sample in a matter of hours instead of days. The tool was made possible by a combination of proprietary chemistry, innovative electrical engineering and high-end imaging and analysis techniques powered by machine learning.
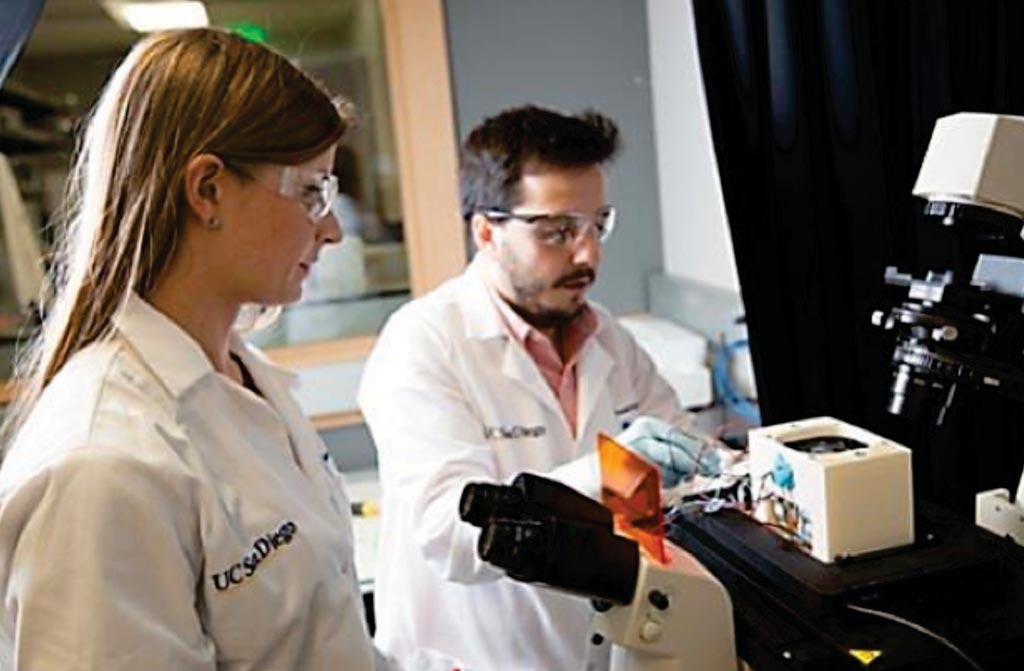
Image: The bioengineering team setting up the device\'s hardware on the high-throughput microscope for analysis (Photo courtesy of the University of California San Diego).
Bioengineers at the University of California San Diego extracted and purified blood from a clinical sample known to be negative for bacteria. Approximately 2,000 genomes of Listeria monocytogenes were added to the purified blood extraction. The maximum amount of the blood and bacterial DNA mixture (8.63 μL) was added to the polymerase chain reaction (PCR) master mix. The DNA was then placed on a digital chip that allowed each piece to independently multiply in its own small reaction. For the process to work at such small scales, each well containing DNA in the chip was only 20 pL in volume.
An MJ Research PTC-200 Thermal Cycler was used for endpoint amplification. The engineers imaged the melting process with the high-throughput microscope and fluorescent imaging was accomplished using a Nikon Eclipse Ti platform and they were able to capture the bacteria's melting curves. They then analyzed the curves with a machine-learning algorithm they developed. In previous work, the algorithm was trained on 37 different types of bacteria undergoing different reactions in different conditions. The scientists showed that it was able to identify bacteria strains with 99% and by contrast, the error rate for traditional methods can be up to 22.6%.
The team concluded that the resulting bacteria-specific melt curves are identified by Support Vector Machine learning, and individual pathogen loads are quantified. The platform reduces reaction volumes by 99.995% and achieves a greater than 200-fold increase in dynamic range of detection compared to traditional PCR high-resolution melting (HRM) approaches. The study was published on February 8, 2017, in the journal Scientific Reports.