New AI Technology Outperforms Traditional Methods in Biomedical Image Segmentation
Posted on 28 Nov 2024
Spatial omics is an emerging field that integrates molecular profiling techniques like genomics, transcriptomics, and proteomics with spatial information, enabling researchers to pinpoint the location of various molecules within cells in complex tissues. This approach offers valuable insights into the cellular mechanisms behind disease development and progression, which is crucial for improving diagnostics and advancing targeted therapies, a central focus in translational research. Spatial omics allows the study of diseases like cancer and chronic kidney disease by revealing how cellular interactions and microenvironments influence disease progression and therapeutic responses. The first step in analyzing spatial omics data involves tasks such as cell segmentation, which defines cell boundaries, and classification, which assigns cell types. Recent advancements in spatial omics technologies enable the examination of intact tissues at the cellular level, providing unparalleled insights into the relationship between cellular architecture and the function of different tissues and organs.
With the increasing volume of spatial omics data, there is a growing demand for advanced computational tools for analysis. In response, researchers at Children’s Hospital of Philadelphia (CHOP, Philadelphia, PA, USA) have developed an artificial intelligence (AI) technology called CelloType, a comprehensive model designed to improve the accuracy of cell identification and classification in high-content tissue images. CHOP is involved in prominent projects such as the Human Tumor Atlas Network, the Human BioMolecular Atlas Program (HuBMAP), and the BRAIN initiative, which use similar technologies to map the spatial organization of both healthy and diseased tissues. The CelloType model utilizes transformer-based deep learning, a type of AI that automates complex, high-dimensional data analysis. Deep learning enables the model to identify complex relationships and context, making it highly effective for natural language processing and image analysis tasks. The model is optimized to enhance accuracy in cell detection, segmentation, and classification.
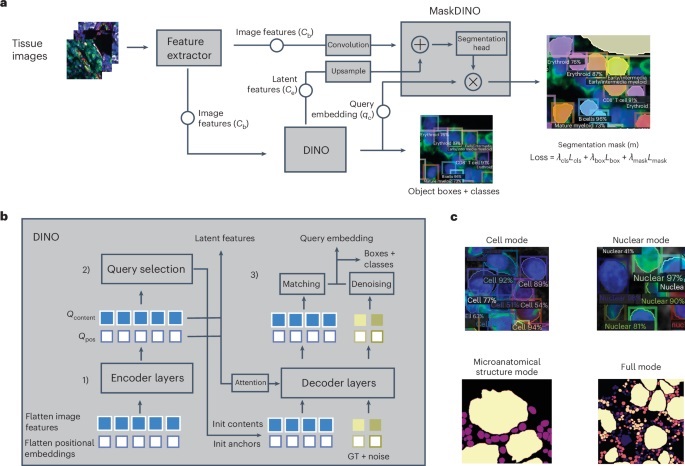
In their study, the researchers compared the performance of CelloType against various traditional methods using datasets from both animal and human tissues. Traditional approaches typically follow a two-stage process of segmentation followed by classification, which can be inefficient and inaccurate. In contrast, CelloType employs a multi-task learning strategy that integrates both segmentation and classification in one step, improving efficiency and accuracy. CelloType also outperformed existing segmentation methods across different types of images, including natural images, bright light images, and fluorescence images. For cell type classification, the study, published in Nature Methods, demonstrated that CelloType surpassed a model made up of state-of-the-art individual methods and a high-performance instance segmentation model, which uses AI to precisely outline objects in an image. Additionally, using a multiplexed tissue image—a type of advanced biomedical image that displays multiple biomarkers in a single tissue sample—researchers showcased how CelloType can perform multi-scale segmentation and classification of both cellular and non-cellular components within a tissue. This capability allows for more detailed analysis of both small and large cell structures, significantly expediting the process.
"We are just beginning to unlock the potential of this technology," said Kai Tan, PhD, the study's lead author and a professor in the Department of Pediatrics at CHOP. "This approach could redefine how we understand complex tissues at the cellular level, paving the way for transformative breakthroughs in healthcare."