AI-Based Tissue Staining Detects Amyloid Deposits Without Chemical Stains or Polarization Microscopy
Posted on 19 Sep 2024
Systemic amyloidosis, a disorder characterized by the buildup of misfolded proteins in organs and tissues, presents significant diagnostic difficulties. The condition affects millions of people each year, often resulting in severe organ damage, heart failure, and high mortality rates if not diagnosed and treated early. Traditionally, the detection of amyloid deposits has relied on Congo red staining viewed under polarized light microscopy, which has been considered the gold standard. However, this method is time-consuming, costly, and prone to variability that can lead to misdiagnoses. Researchers have now developed a groundbreaking method for imaging and detecting amyloid deposits in tissue samples. This innovative approach uses deep learning and autofluorescence microscopy to create virtual birefringence imaging and histological staining, removing the need for polarization imaging and traditional stains like Congo red.
The new technique, described in Nature Communications and developed by researchers at the University of California, Los Angeles (UCLA, Los Angeles, CA, USA), employs a single neural network to convert autofluorescence images of unstained tissue into high-resolution brightfield and polarized microscopy images. These images resemble those produced by conventional histochemical staining and polarization microscopy. The method was tested on cardiac tissue samples and demonstrated that the virtually stained images consistently and accurately identified amyloid patterns. This approach eliminates the need for chemical staining and specialized polarization microscopes, potentially accelerating diagnosis and lowering costs. The virtual staining process matched and even surpassed the quality of traditional methods, as confirmed by multiple board-certified pathologists from UCLA.
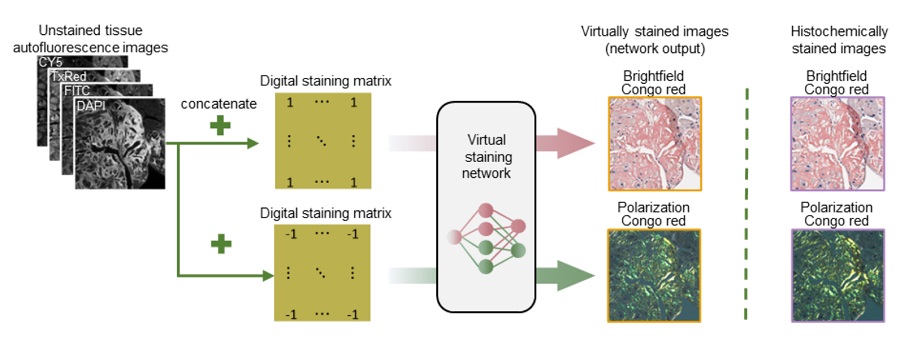
The study’s results indicate that this virtual staining technique could be easily incorporated into current clinical workflows, encouraging wider use of digital pathology. The method does not require specialized optical components and can be deployed on standard digital pathology scanners, making it accessible to a broad range of healthcare facilities. Researchers plan to extend their evaluations to other tissue types, including kidney, liver, and spleen, to further validate the technique's effectiveness across various forms of amyloidosis. They also aim to develop automated detection systems to assist pathologists in identifying problematic regions, potentially enhancing diagnostic accuracy and minimizing false negatives.
“Our deep learning model can perform both autofluorescence-to-birefringence and autofluorescence-to-brightfield image transformations, offering a reliable, consistent, and cost-effective alternative to traditional histology methods. This breakthrough could greatly enhance the speed and accuracy of amyloidosis diagnosis, reducing the risk of false negatives and improving patient outcomes,” said Dr. Aydogan Ozcan, the senior author of the study and the Volgenau Chair for Engineering Innovation at UCLA. “This innovation represents a significant step forward in the field of amyloidosis pathology. It not only simplifies the diagnostic process but also holds potential for expanding the use of digital pathology in routine clinical practice, particularly in resource-limited settings.”