AI Integrated With Optical Imaging Technology Enables Rapid Intraoperative Diagnosis
Posted on 17 May 2024
Rapid and accurate intraoperative diagnosis is essential for tumor surgery as it guides surgical decisions with precision. Traditional intraoperative assessments, such as frozen sections based on H&E histology, are demanding in terms of time, resources, and labor and also raise concerns about specimen consumption. D-FFOCT, a high-resolution optical imaging technology, allows for the quick generation of virtual histology. Researchers have now developed an intraoperative diagnostic workflow that uses deep learning algorithms to classify tumors from D-FFOCT images, offering rapid and automated diagnosis for surgical decision-making.
A prospective cohort study conducted by researchers from Peking University People’s Hospital (Beijing, China) included 224 breast samples imaged using D-FFOCT. This imaging technique is non-destructive and requires no tissue preparation or staining. The D-FFOCT images were segmented into patches, and slides were allocated into a training set (182 slides, 10,357 patches) and an external testing set (42 slides, 3,140 patches) based on the order in which they were collected. A five-fold cross-validation method was employed to train and fine-tune the model. A machine learning model aggregated the patch prediction results to the slide level after feature extraction.
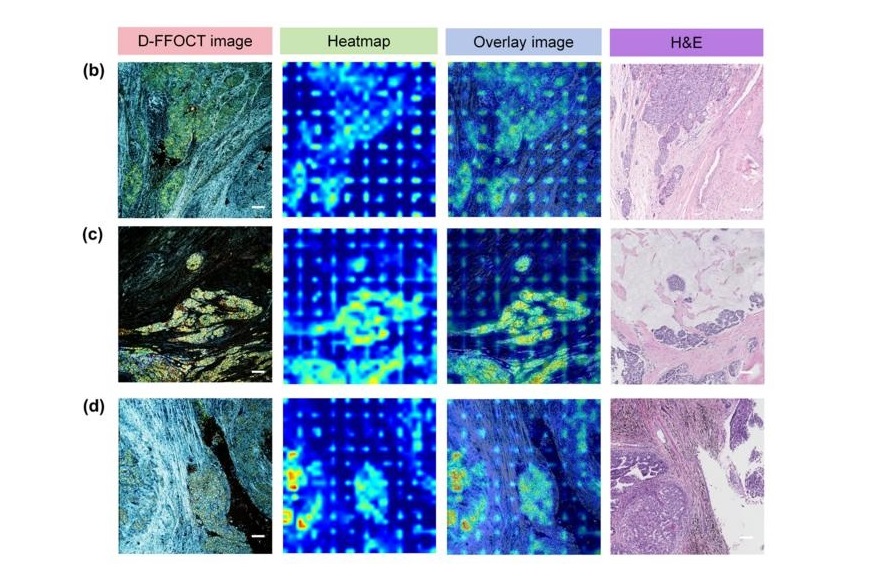
The testing set showed the model performed well at the patch level, identifying breast tissue types with an AUC of 0.926 (95% CI: 0.907–0.943). At the slide level, the diagnostic accuracy reached 97.62%, with a sensitivity of 96.88% and a specificity of 100%. Accuracy did not significantly differ across various molecular subtypes and histologic tumor types of breast cancer. Visualization heatmaps demonstrated that the deep learning models could identify features corresponding to metabolically active cell clusters in D-FFOCT images, aligning with expert assessments. This image analysis approach could potentially extend to various tumor types, given the conserved features detected in the model. In a margin simulation experiment, the diagnosis process took about three minutes, with the deep learning model achieving a high accuracy of 95.24%.
Based on the results, the study has proposed an intraoperative cancer diagnosis workflow integrating D-FFOCT with a deep learning model. In simulated intraoperative margin diagnosis, the workflow substantially reduced diagnosis time by about tenfold compared to traditional methods and proved to be highly cost-effective in terms of labor. No tissue was destroyed during optical imaging and analysis. Overall, this workflow offers a transparent solution for rapid and accurate intraoperative diagnosis, potentially guiding surgical decisions effectively.
Related Links:
Peking University People’s Hospital