AI Predicts Multiple Sclerosis Risk, Flags Potentially Contaminated Lab Results
Posted on 27 Jul 2023
New research presented at the 2023 AACC Annual Scientific Meeting & Clinical Lab Expo has shown that an artificial intelligence (AI) model can predict the likelihood of individuals developing multiple sclerosis (MS) years before its diagnosis. Such prediction could allow for earlier treatment initiation, potentially slowing the progression of this neurological disorder. Breaking results from another study have revealed that machine learning (ML) can be instrumental in identifying laboratory samples contaminated with intravenous fluids. This important discovery could help minimize laboratory errors that tend to slow down diagnosis, increase healthcare expenses, and lead to incorrect treatments. Both these studies indicate the huge strides made in the use of AI and ML to enhance patient care.
MS, a disease of the nervous system, affects over 2.8 million people globally. While its exact cause remains unclear, the disease is linked to autoimmunity, where the immune system mistakenly attacks healthy cells, as well as to genetics, the Epstein-Barr virus, and other factors. Currently, MS diagnosis relies on imaging, cerebrospinal fluid studies, and clinical history. However, there is a need for early-detection methods as they could help start treatment earlier, thus slowing down disease progression.
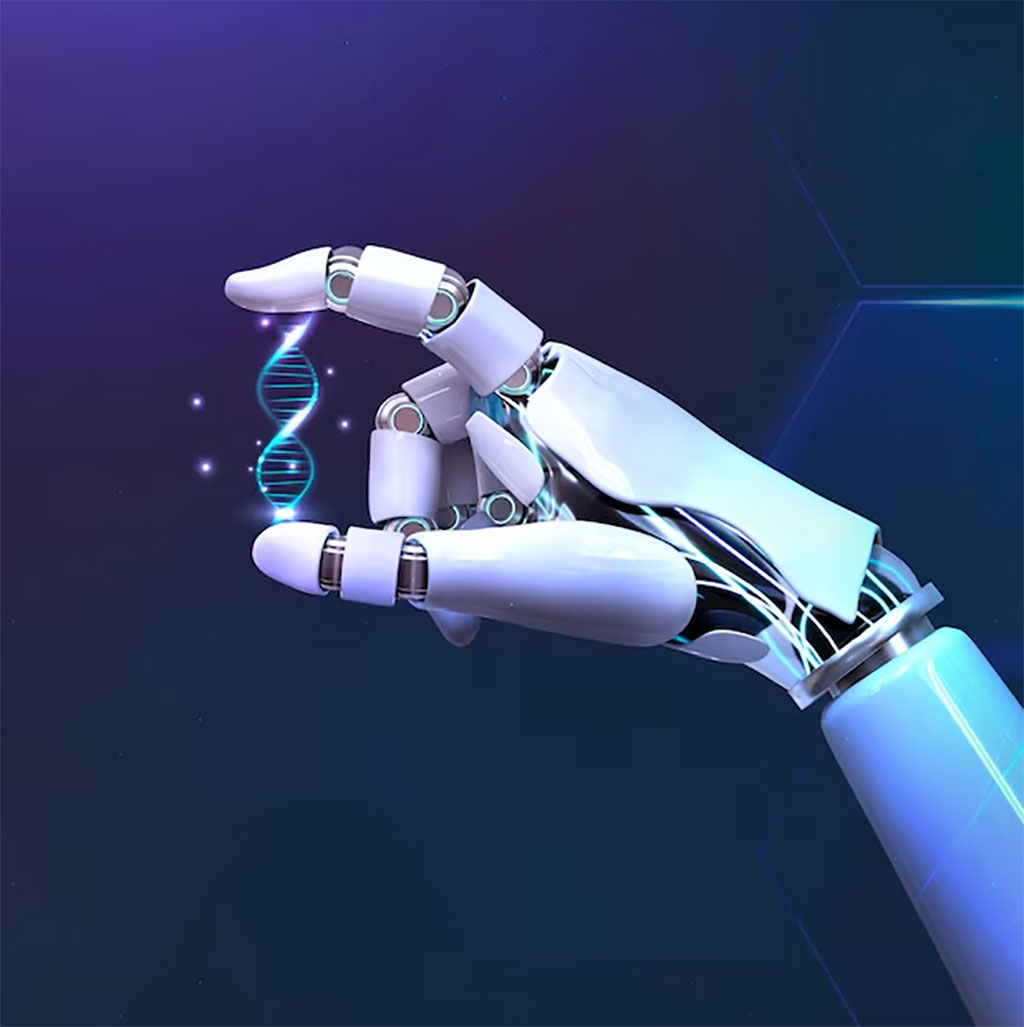
In the first study, a team of researchers at Siemens Healthineers (Erlangen, Germany) trained machine-learning models to predict the risk of MS. Over 3,000 data sets from the electronic health records of MS patients and others were used for the study. Their "random forest model" parses data on a patient’s age, gender, blood, and metabolic markers, obtained up to three years prior to diagnosis. The model demonstrated high accuracy and strong predictive ability. The key factors contributing to the model's ability to identify high-risk patients were blood measurements of neutrophils, red blood cells, and other markers. These predictions remained consistent up to three years before diagnosis.
“Our model’s performance suggests that AI-based prediction models could identify the risk for multiple sclerosis years before neurological symptoms appear,” said Raj Gopalan, MD, at Siemens Healthineers who led the research team. “This could reveal which patients should be monitored for periodic neurological and cognitive exams when symptoms appear. In addition, early confirmation of the diagnosis with imaging and cerebrospinal fluid studies could facilitate disease-modifying treatment.”
In a separate study, a research team led by scientists at Washington University School of Medicine in St. Louis (St. Louis, MO, USA) used a "mixture-of-experts" modeling technique to develop an ML-based system capable of detecting instances of IV fluid contamination that were missed by manual methods. Currently, scientists are utilizing ML to identify potential contaminations in lab samples that could affect test results. When samples are collected directly from IV catheters instead of a fresh blood draw, the fluid within can lead to false lab results that delay diagnosis, increase healthcare costs, and result in incorrect treatments. Existing contamination detection methods are not always reliable and often require technicians to undertake extensive manual analysis.
The research team gathered over 9.6 million chemistry results from patients and simulated IV fluid contamination in some samples with common IV solutions. By training different machine-learning models using the simulated results, they generated a final set of predictions. The models detected significant contamination in several thousand samples. The newly-developed pipeline is capable of detecting 5 to 10 times more contaminated samples compared to the existing methods. A vast majority of these tests evaded being previously flagged using manual methods –up to 94% in the case of samples contaminated with lactated Ringer's solution.
“While this won’t immediately reduce the number of contaminated tests, it will hopefully substantially reduce the operational and clinical impact of these events when they do happen, and provide us with a better quality metric with which we can prioritize areas for improvement initiatives,” said Nicholas Spies, MD, at Washington University School of Medicine in St. Louis, who led the research team.
Related Links:
Siemens Healthineers
Washington University School of Medicine in St. Louis