Bioinformatics Tool for Evaluating the Evaluation of Cancer Genes
By LabMedica International staff writers
Posted on 28 Dec 2016
Researchers offer a new software tool being developed to help assess computational algorithms used in methods to identify mutant genes that drive cancer. The tool could thereby lead to more precise diagnostics and targeted treatments for patients.Posted on 28 Dec 2016
In the search for new ways to tackle cancer, many scientists use genome sequencing to hunt for mutations that facilitate tumor cell growth. To aid in this search, some researchers have developed new bioinformatics methods that each claim to help pinpoint the cancer driver mutants. But a question remains: Among the numerous new tactics, which produce more accurate results?
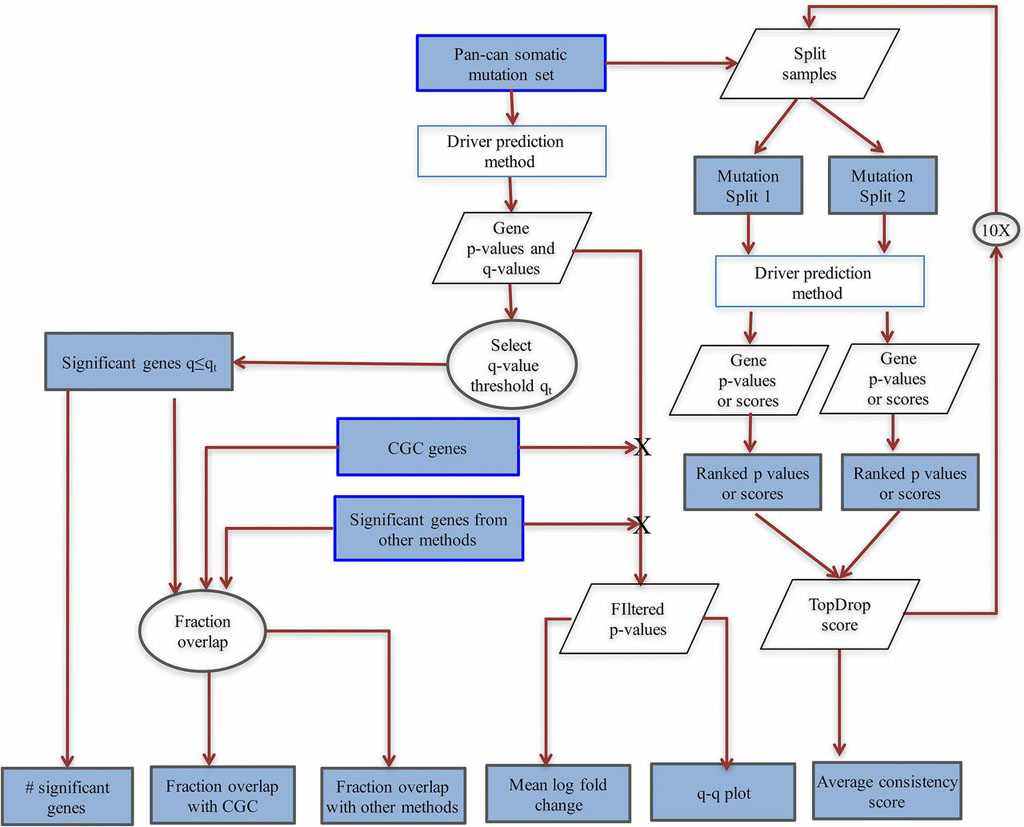
Image: Flowchart of evaluation protocol portraying how a cancer driver gene prediction method of interest can be evaluated. The input to the method is a provided pancan somatic mutation set. The initial output from the method to be evaluated is a list of predicted driver genes with associated P values and q values. A list of significant driver genes is produced by selecting a q value threshold. Additional details can be found in the paper (Photo courtesy of the journal Proceedings of the National Academy of Sciences).
To help solve this puzzle, a team of Johns Hopkins University (Baltimore, MD, USA) computational scientists and cancer experts devised the new tool to help assess how well current strategies work in identifying cancer-promoting mutations and distinguishing them from benign mutations in cancer cells.
"Identifying the genes that cause cancer when altered is often challenging, but is critical for directing research along the most fruitful course," said co-author Bert Vogelstein, Johns Hopkins Kimmel Cancer Center, "This paper establishes novel ways to judge the techniques used to identify true cancer-causing genes and should considerably facilitate advances in this field in the future."
Lead author Collin J. Tokheim, doctoral student in the laboratory of senior author Rachel Karchin, associate professor at Johns Hopkins, said one of the challenges the team faced was the lack of a widely accepted consensus on what qualifies as a cancer driver gene. "People have lists of what they consider to be cancer driver genes, but there's no official reference guide, no gold standard," said Tokheim. Nevertheless, the team was able to develop a machine-learning-based method for driver gene prediction and a framework for evaluating and comparing other prediction methods.
For the study, this evaluation tool was applied to 8 existing cancer driver gene prediction methods. The results were not very reassuring. “These methods still need to get better. We're sharing our methodology publicly, and it should help others to improve their systems for identifying cancer driver genes," said Tokheim.
The study, by Tokheim CJ et al, was published December 13, 2016, in the journal Proceedings of the National Academy of Sciences.
Related Links:
Johns Hopkins University